filmov
tv
The ARC Prize 2024 Winning Algorithm
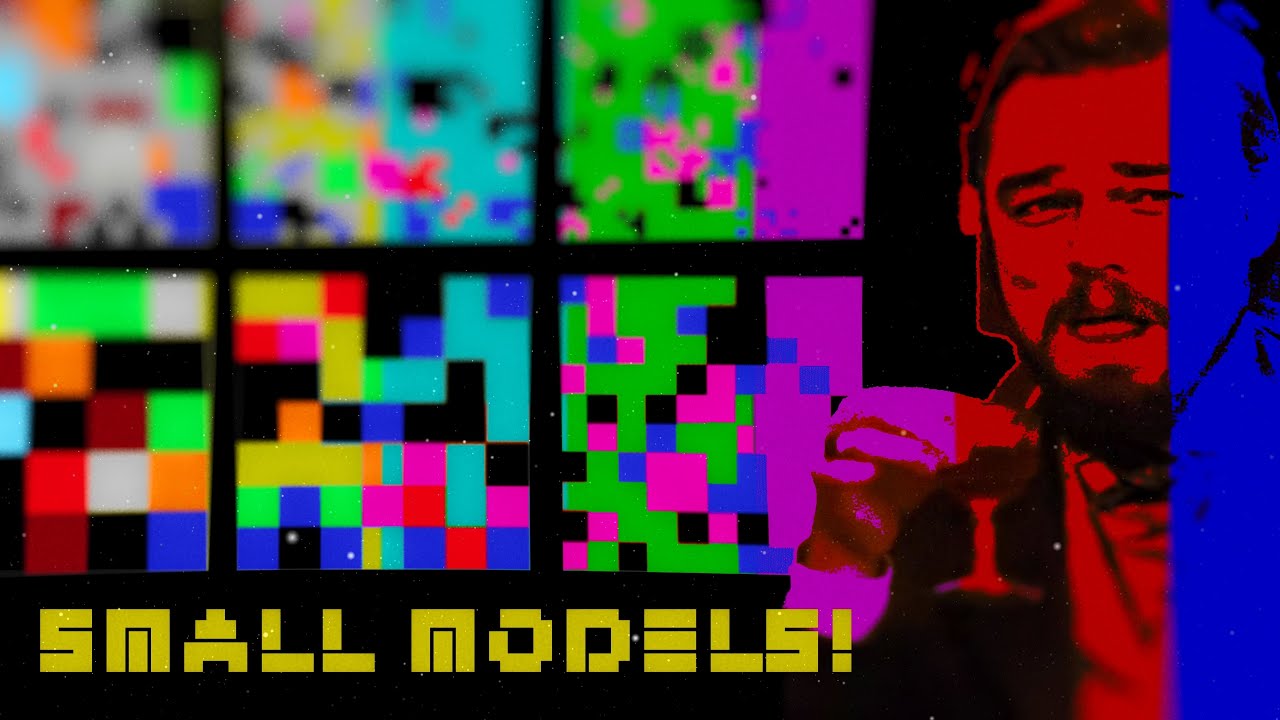
Показать описание
Daniel Franzen and Jan Disselhoff, the "ARChitects" are the official winners (with co-researcher David Hartmann) of the ARC Prize 2024. Filmed at Tufa Labs in Zurich - they revealed how they achieved a remarkable 53.5% accuracy by creatively utilising large language models (LLMs) in new ways. Discover their innovative techniques, including depth-first search for token selection, test-time training, and a novel augmentation-based validation system. Their results were extremely surprising.
SPONSOR MESSAGES:
***
CentML offers competitive pricing for GenAI model deployment, with flexible options to suit a wide range of models, from small to large-scale deployments. Check out their super fast DeepSeek R1 hosting!
Tufa AI Labs is a brand new research lab in Zurich started by Benjamin Crouzier focussed on o-series style reasoning and AGI. They are hiring a Chief Engineer and ML engineers. Events in Zurich.
***
Jan Disselhoff
Daniel Franzen
TRANSCRIPT AND BACKGROUND READING:
TOC
1. Solution Architecture and Strategy Overview
[00:00:00] 1.1 Initial Solution Overview and Model Architecture
[00:04:25] 1.2 LLM Capabilities and Dataset Approach
[00:10:51] 1.3 Test-Time Training and Data Augmentation Strategies
[00:14:08] 1.4 Sampling Methods and Search Implementation
[00:17:52] 1.5 ARC vs Language Model Context Comparison
2. LLM Search and Model Implementation
[00:21:53] 2.1 LLM-Guided Search Approaches and Solution Validation
[00:27:04] 2.2 Symmetry Augmentation and Model Architecture
[00:30:11] 2.3 Model Intelligence Characteristics and Performance
[00:37:23] 2.4 Tokenization and Numerical Processing Challenges
3. Advanced Training and Optimization
[00:45:15] 3.1 DFS Token Selection and Probability Thresholds
[00:49:41] 3.2 Model Size and Fine-tuning Performance Trade-offs
[00:53:07] 3.3 LoRA Implementation and Catastrophic Forgetting Prevention
[00:56:10] 3.4 Training Infrastructure and Optimization Experiments
[01:02:34] 3.5 Search Tree Analysis and Entropy Distribution Patterns
REFS
[00:01:05] Winning ARC 2024 solution using 12B param model, Franzen, Disselhoff, Hartmann
[00:03:40] Robustness of analogical reasoning in LLMs, Melanie Mitchell
[00:07:50] Re-ARC dataset generator for ARC task variations, Michael Hodel
[00:15:00] Analysis of search methods in LLMs (greedy, beam, DFS), Chen et al.
[00:16:55] Language model reachability space exploration, University of Toronto
[00:22:30] GPT-4 guided code solutions for ARC tasks, Ryan Greenblatt
[00:41:20] GPT tokenization approach for numbers, OpenAI
[00:46:25] DFS in AI search strategies, Russell & Norvig
[00:53:10] Paper on catastrophic forgetting in neural networks, Kirkpatrick et al.
[00:54:00] LoRA for efficient fine-tuning of LLMs, Hu et al.
[00:57:20] NVIDIA H100 Tensor Core GPU specs, NVIDIA
[01:04:55] Original MCTS in computer Go, Yifan Jin
SPONSOR MESSAGES:
***
CentML offers competitive pricing for GenAI model deployment, with flexible options to suit a wide range of models, from small to large-scale deployments. Check out their super fast DeepSeek R1 hosting!
Tufa AI Labs is a brand new research lab in Zurich started by Benjamin Crouzier focussed on o-series style reasoning and AGI. They are hiring a Chief Engineer and ML engineers. Events in Zurich.
***
Jan Disselhoff
Daniel Franzen
TRANSCRIPT AND BACKGROUND READING:
TOC
1. Solution Architecture and Strategy Overview
[00:00:00] 1.1 Initial Solution Overview and Model Architecture
[00:04:25] 1.2 LLM Capabilities and Dataset Approach
[00:10:51] 1.3 Test-Time Training and Data Augmentation Strategies
[00:14:08] 1.4 Sampling Methods and Search Implementation
[00:17:52] 1.5 ARC vs Language Model Context Comparison
2. LLM Search and Model Implementation
[00:21:53] 2.1 LLM-Guided Search Approaches and Solution Validation
[00:27:04] 2.2 Symmetry Augmentation and Model Architecture
[00:30:11] 2.3 Model Intelligence Characteristics and Performance
[00:37:23] 2.4 Tokenization and Numerical Processing Challenges
3. Advanced Training and Optimization
[00:45:15] 3.1 DFS Token Selection and Probability Thresholds
[00:49:41] 3.2 Model Size and Fine-tuning Performance Trade-offs
[00:53:07] 3.3 LoRA Implementation and Catastrophic Forgetting Prevention
[00:56:10] 3.4 Training Infrastructure and Optimization Experiments
[01:02:34] 3.5 Search Tree Analysis and Entropy Distribution Patterns
REFS
[00:01:05] Winning ARC 2024 solution using 12B param model, Franzen, Disselhoff, Hartmann
[00:03:40] Robustness of analogical reasoning in LLMs, Melanie Mitchell
[00:07:50] Re-ARC dataset generator for ARC task variations, Michael Hodel
[00:15:00] Analysis of search methods in LLMs (greedy, beam, DFS), Chen et al.
[00:16:55] Language model reachability space exploration, University of Toronto
[00:22:30] GPT-4 guided code solutions for ARC tasks, Ryan Greenblatt
[00:41:20] GPT tokenization approach for numbers, OpenAI
[00:46:25] DFS in AI search strategies, Russell & Norvig
[00:53:10] Paper on catastrophic forgetting in neural networks, Kirkpatrick et al.
[00:54:00] LoRA for efficient fine-tuning of LLMs, Hu et al.
[00:57:20] NVIDIA H100 Tensor Core GPU specs, NVIDIA
[01:04:55] Original MCTS in computer Go, Yifan Jin
Комментарии