filmov
tv
Marlos C. Machado - Representation-driven Option Discovery in Reinforcement Learning
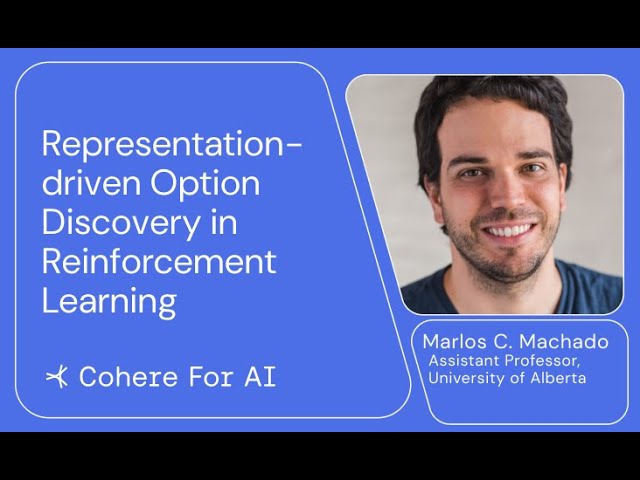
Показать описание
Representation-driven Option Discovery in Reinforcement Learning
Abstract: The ability to reason at multiple levels of temporal abstraction is a fundamental aspect of intelligence. In reinforcement learning, this attribute is often modeled through temporally extended courses of actions called options. Despite the popularity of options as a research topic, they are seldom included as an explicit component in traditional solutions within the field. In this talk, I will try to provide an answer for why this is the case and emphasize the vital role options can play in continual learning. Rather than assuming a predetermined set of options, I will introduce a general framework for option discovery, which utilizes the agent's representation to discover useful options. By leveraging these options to generate a rich stream of experience, the agent can improve its representations and learn more effectively.
This session is brought to you by the Cohere For AI Open Science Community - a space where ML researchers, engineers, linguists, social scientists, and lifelong learners connect and collaborate with each other. Thank you to our Community Leads for organizing and hosting this event.
Abstract: The ability to reason at multiple levels of temporal abstraction is a fundamental aspect of intelligence. In reinforcement learning, this attribute is often modeled through temporally extended courses of actions called options. Despite the popularity of options as a research topic, they are seldom included as an explicit component in traditional solutions within the field. In this talk, I will try to provide an answer for why this is the case and emphasize the vital role options can play in continual learning. Rather than assuming a predetermined set of options, I will introduce a general framework for option discovery, which utilizes the agent's representation to discover useful options. By leveraging these options to generate a rich stream of experience, the agent can improve its representations and learn more effectively.
This session is brought to you by the Cohere For AI Open Science Community - a space where ML researchers, engineers, linguists, social scientists, and lifelong learners connect and collaborate with each other. Thank you to our Community Leads for organizing and hosting this event.