filmov
tv
Discover Apache Iceberg: The Top 5 Features You Need to Know
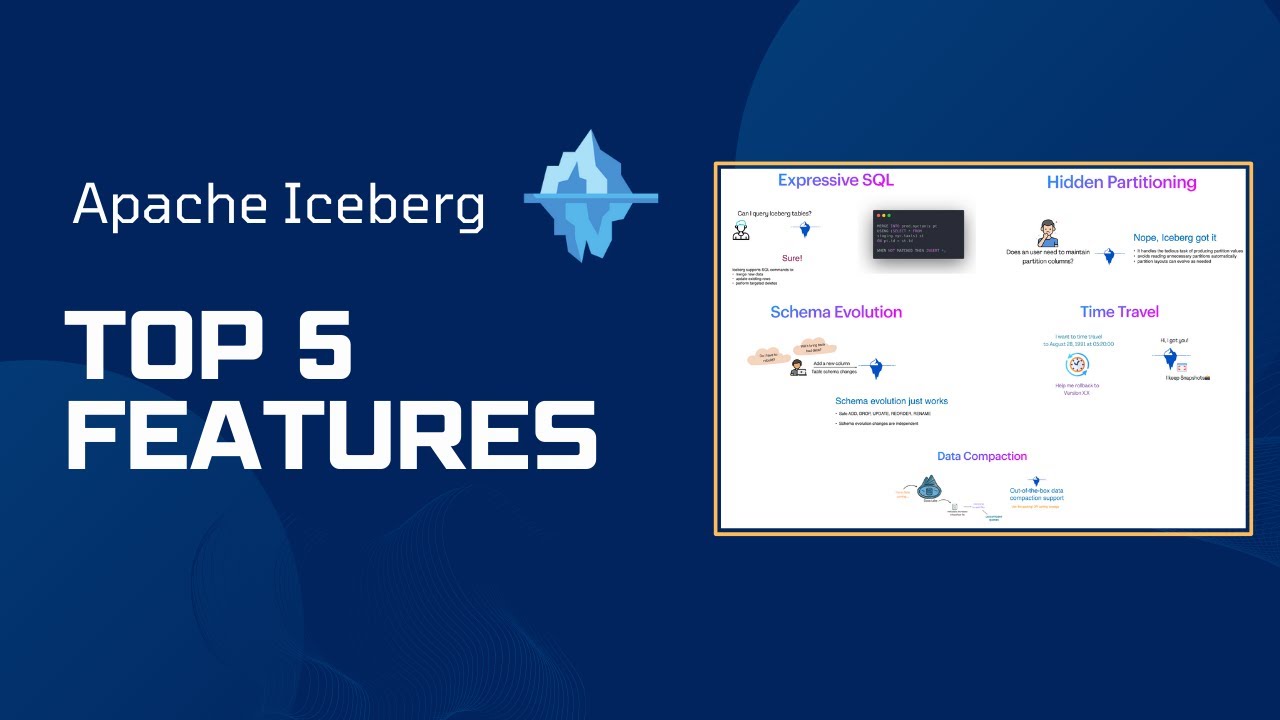
Показать описание
Discover the Top 5 Features of Apache Iceberg with Dremio's Developer Advocate Dipankar! Apache Iceberg is a table format that enables data lakes to store large amounts of data in an efficient and cost-effective manner. It was created to facilitate the storage of large, immutable datasets and is suitable for use in both data warehouses and data lakehouses. In this video, Dipankar will provide an overview of the five most important features of Apache Iceberg, so you can use it to its full potential.
The first feature of Apache Iceberg is its ability to store large amounts of data without sacrificing performance or scalability. By utilizing advanced techniques such as partitioning and bucketing, Apache Iceberg can store enormous datasets without sacrificing performance or scalability. This makes it perfect for both Data Lakehouse and Data Warehouse applications.
The second feature is its support for a wide variety of file formats. Apache Iceberg supports both Parquet and ORC file formats, allowing users to store their data in whatever format best suits their needs. This makes it easy to integrate with existing systems and ensures that users can access the most up-to-date versions of their datasets quickly and easily.
The third feature is its built-in security measures. With Apache Iceberg, users can easily control access to their data by setting up fine-grained permissions on individual files or directories. This helps ensure that only authorized users are able to access sensitive information stored in the Data Lakehouse or Data Warehouse.
The fourth feature is its ability to manage versioning efficiently. With Apache Iceberg, users are able to easily manage multiple versions of their datasets without sacrificing performance or scalability. This makes it easy for users to back up their data while ensuring that they always have access to the most up-to-date version available.
Finally, the fifth feature is its integration with popular analytics frameworks such as Spark and PrestoDB . By leveraging these frameworks, users can take advantage of advanced analytics capabilities while still being able to use the same underlying Data Lake Engine technology as before. This allows users to gain insights from their datasets faster than ever before while still being able to leverage existing infrastructure investments they may have already made into these popular analytics frameworks..
Connect with us!
The first feature of Apache Iceberg is its ability to store large amounts of data without sacrificing performance or scalability. By utilizing advanced techniques such as partitioning and bucketing, Apache Iceberg can store enormous datasets without sacrificing performance or scalability. This makes it perfect for both Data Lakehouse and Data Warehouse applications.
The second feature is its support for a wide variety of file formats. Apache Iceberg supports both Parquet and ORC file formats, allowing users to store their data in whatever format best suits their needs. This makes it easy to integrate with existing systems and ensures that users can access the most up-to-date versions of their datasets quickly and easily.
The third feature is its built-in security measures. With Apache Iceberg, users can easily control access to their data by setting up fine-grained permissions on individual files or directories. This helps ensure that only authorized users are able to access sensitive information stored in the Data Lakehouse or Data Warehouse.
The fourth feature is its ability to manage versioning efficiently. With Apache Iceberg, users are able to easily manage multiple versions of their datasets without sacrificing performance or scalability. This makes it easy for users to back up their data while ensuring that they always have access to the most up-to-date version available.
Finally, the fifth feature is its integration with popular analytics frameworks such as Spark and PrestoDB . By leveraging these frameworks, users can take advantage of advanced analytics capabilities while still being able to use the same underlying Data Lake Engine technology as before. This allows users to gain insights from their datasets faster than ever before while still being able to leverage existing infrastructure investments they may have already made into these popular analytics frameworks..
Connect with us!