filmov
tv
Keynote Presentation: Putting Machine Learning Models into Large Scale Production for Drug Discovery
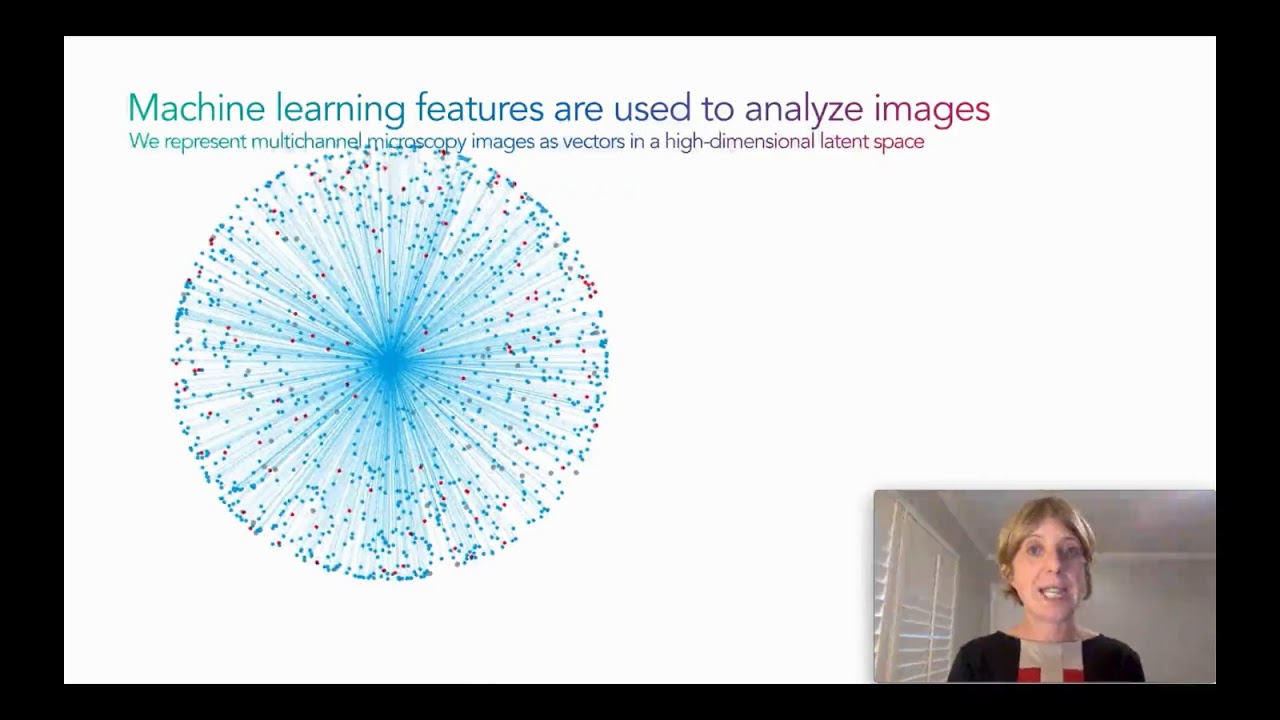
Показать описание
Presented By: Lina Nilsson, PhD
Speaker Biography: Lina Nilsson is Senior Director of Data Science Product at Recursion, a biotech that is reimagining drug discovery using machine learning and large-scale laboratory automation. Previously, she was the COO of Enlitic, a startup that uses deep learning to improve clinical radiology. As Innovation Director at University of California, Berkeley, she helped spin out social-impact technologies. Lina has been recognized on MIT Technology Review's "TR35" annual list of the world's top innovators. Her writings on open science, social impact technologies, and gender & tech have been published in the New York Times, Washington Post, and Science. Lina has a PhD in biomedical engineering from the ETH Zurich.
Webinar: Keynote Presentation: Putting Machine Learning Models into Large Scale Production for Drug Discovery
Webinar Abstract: This talk will peek under the hood to show how we combine deep learning models with biology lab automation at Recursion Pharmaceuticals. Every week, we generate millions of microscopy images of human cells on our high throughput screening platform as part of our search for new drug treatments. Here, I’ll describe how we use machine learning analytics to make sense of all of this complex data - that is, to identify promising drug compounds while simultaneously avoiding high risk ones. As part of our aim to ‘industrialize drug discovery’, we have developed a single, large-volume platform approach that can be applied across large numbers of diseases. I will show how running such a high sophisticated experiment pipeline relies on a close integration of biology, engineering and data science product development: Engineering engineering infrastructure that can handle petabytes of data; Experimental biology setups that are optimized best enable downstream machine learning tasks; deep learning models that work not just on small, pre-defined datasets, but on large quantities of future data. Cumulatively, you will see a vision of medical discovery at an industrial scale.
Speaker Biography: Lina Nilsson is Senior Director of Data Science Product at Recursion, a biotech that is reimagining drug discovery using machine learning and large-scale laboratory automation. Previously, she was the COO of Enlitic, a startup that uses deep learning to improve clinical radiology. As Innovation Director at University of California, Berkeley, she helped spin out social-impact technologies. Lina has been recognized on MIT Technology Review's "TR35" annual list of the world's top innovators. Her writings on open science, social impact technologies, and gender & tech have been published in the New York Times, Washington Post, and Science. Lina has a PhD in biomedical engineering from the ETH Zurich.
Webinar: Keynote Presentation: Putting Machine Learning Models into Large Scale Production for Drug Discovery
Webinar Abstract: This talk will peek under the hood to show how we combine deep learning models with biology lab automation at Recursion Pharmaceuticals. Every week, we generate millions of microscopy images of human cells on our high throughput screening platform as part of our search for new drug treatments. Here, I’ll describe how we use machine learning analytics to make sense of all of this complex data - that is, to identify promising drug compounds while simultaneously avoiding high risk ones. As part of our aim to ‘industrialize drug discovery’, we have developed a single, large-volume platform approach that can be applied across large numbers of diseases. I will show how running such a high sophisticated experiment pipeline relies on a close integration of biology, engineering and data science product development: Engineering engineering infrastructure that can handle petabytes of data; Experimental biology setups that are optimized best enable downstream machine learning tasks; deep learning models that work not just on small, pre-defined datasets, but on large quantities of future data. Cumulatively, you will see a vision of medical discovery at an industrial scale.
Комментарии