filmov
tv
Machine Learning Tutorial Python - 15: Naive Bayes Classifier Algorithm Part 2
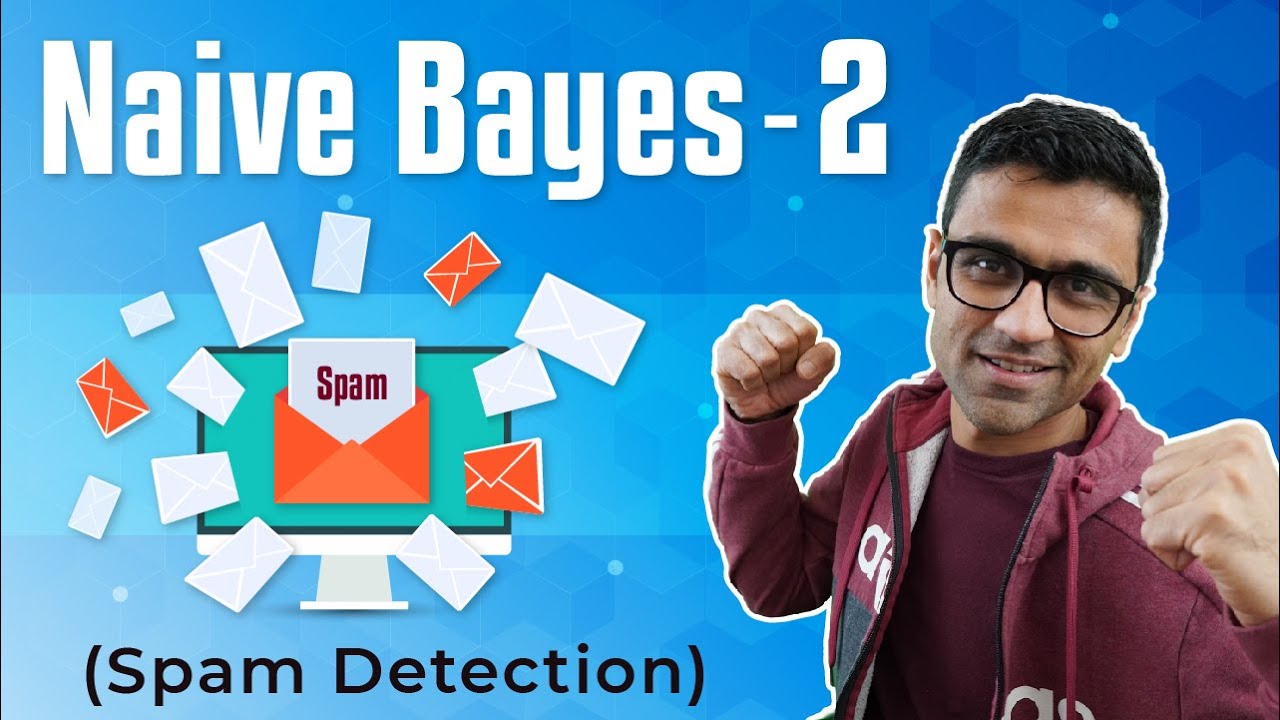
Показать описание
In this python machine learning tutorial for beginners we will build email spam classifier using naive bayes algorithm. We will use sklearn CountVectorizer to convert email text into a matrix of numbers and then use sklearn MultinomialNB classifier to train our model. The model score
with this approach comes out to be very high (around 98%). Sklearn pipeline allows us to handle pre processing transformations easily with its convenient api. In the end there is an exercise where you need to classify sklearn wine dataset using naive bayes.
#MachineLearning #PythonMachineLearning #MachineLearningTutorial #Python #PythonTutorial #PythonTraining #MachineLearningCource #NaiveBayes #sklearntutorials #scikitlearntutorials
Topics that are covered in this Video:
00:00 explore spam email dataset
02:33 sklearn CountVectorizer
04:30 types of naive bayes classifiers
05:23 sklearn MultinomialNB classifier
06:48 sklearn pipeline
09:35 Exercise
Next Video:
Populor Playlist:
Tools and Libraries:
Scikit learn tutorials
Sklearn tutorials
Machine learning with scikit learn tutorials
Machine learning with sklearn tutorials
with this approach comes out to be very high (around 98%). Sklearn pipeline allows us to handle pre processing transformations easily with its convenient api. In the end there is an exercise where you need to classify sklearn wine dataset using naive bayes.
#MachineLearning #PythonMachineLearning #MachineLearningTutorial #Python #PythonTutorial #PythonTraining #MachineLearningCource #NaiveBayes #sklearntutorials #scikitlearntutorials
Topics that are covered in this Video:
00:00 explore spam email dataset
02:33 sklearn CountVectorizer
04:30 types of naive bayes classifiers
05:23 sklearn MultinomialNB classifier
06:48 sklearn pipeline
09:35 Exercise
Next Video:
Populor Playlist:
Tools and Libraries:
Scikit learn tutorials
Sklearn tutorials
Machine learning with scikit learn tutorials
Machine learning with sklearn tutorials
Комментарии