filmov
tv
7 Popular LLM Benchmarks Explained [OpenLLM Leaderboard & Chatbot Arena]
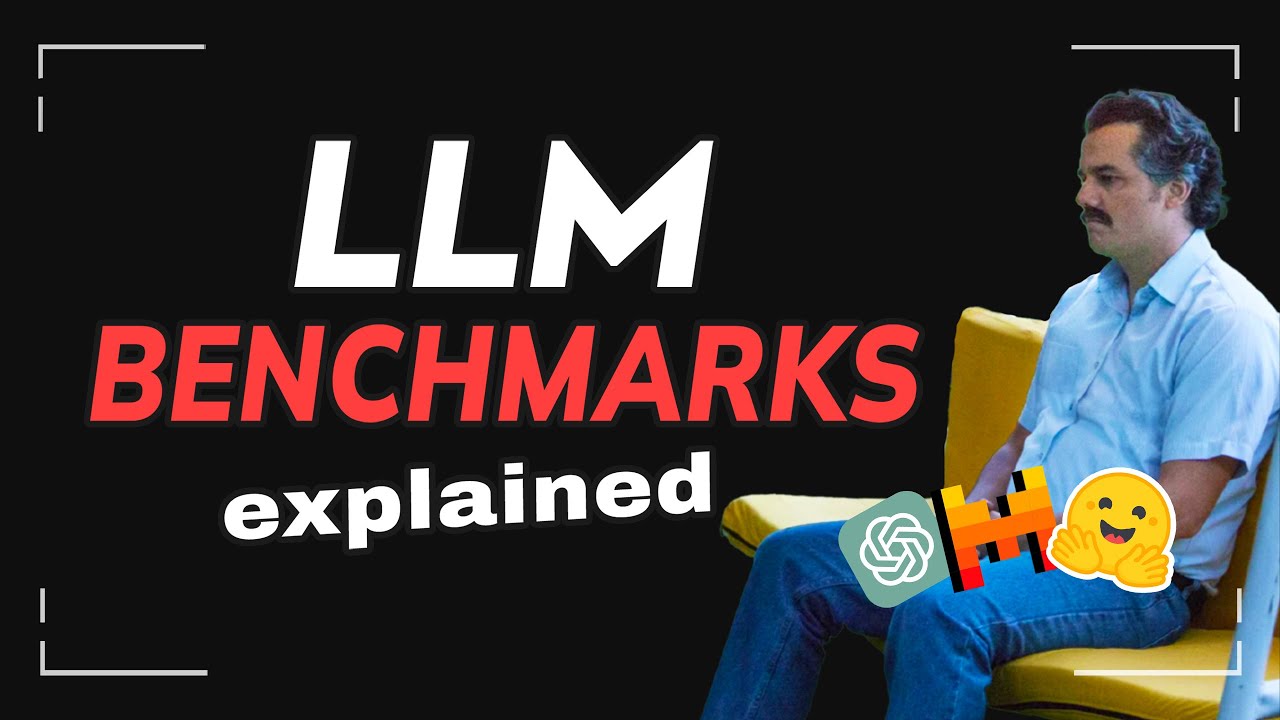
Показать описание
In this video, I will be going through and explain the benchmarks for Chatbot Arena & Open LLM leaderboard. These are more general benchmarks for text-based LLMs, so HumanEval is not here. Let me know any other benchmarks you want me to explain in the future!
This video is supported by the kind Patrons & YouTube Members:
🙏Andrew Lescelius, alex j, Chris LeDoux, Alex Maurice, Miguilim, Deagan, FiFaŁ, Daddy Wen, Tony Jimenez, Panther Modern, Jake Disco, Demilson Quintao, Shuhong Chen, Hongbo Men, happi nyuu nyaa, Carol Lo, Mose Sakashita, Miguel, Bandera, Gennaro Schiano, gunwoo, Ravid Freedman, Mert Seftali, Mrityunjay, Richárd Nagyfi, Timo Steiner, Henrik G Sundt, projectAnthony, Brigham Hall, Kyle Hudson, Kalila, Jef Come, Jvari Williams, Tien Tien, BIll Mangrum, owned, Janne Kytölä, SO, Richárd Nagyfi
[Video Editor] Silas
0:00 Intro
0:57 MMLU
1:41 ARC
2:10 HELLASWAG
2:57 Winograde
3:27 TruthfulQA
3:52 GSM8K
4:26 MT-Bench
5:05 Outro
Комментарии