filmov
tv
Gregory Schwartzman - SGD Through the Lens of Kolmogorov Complexity
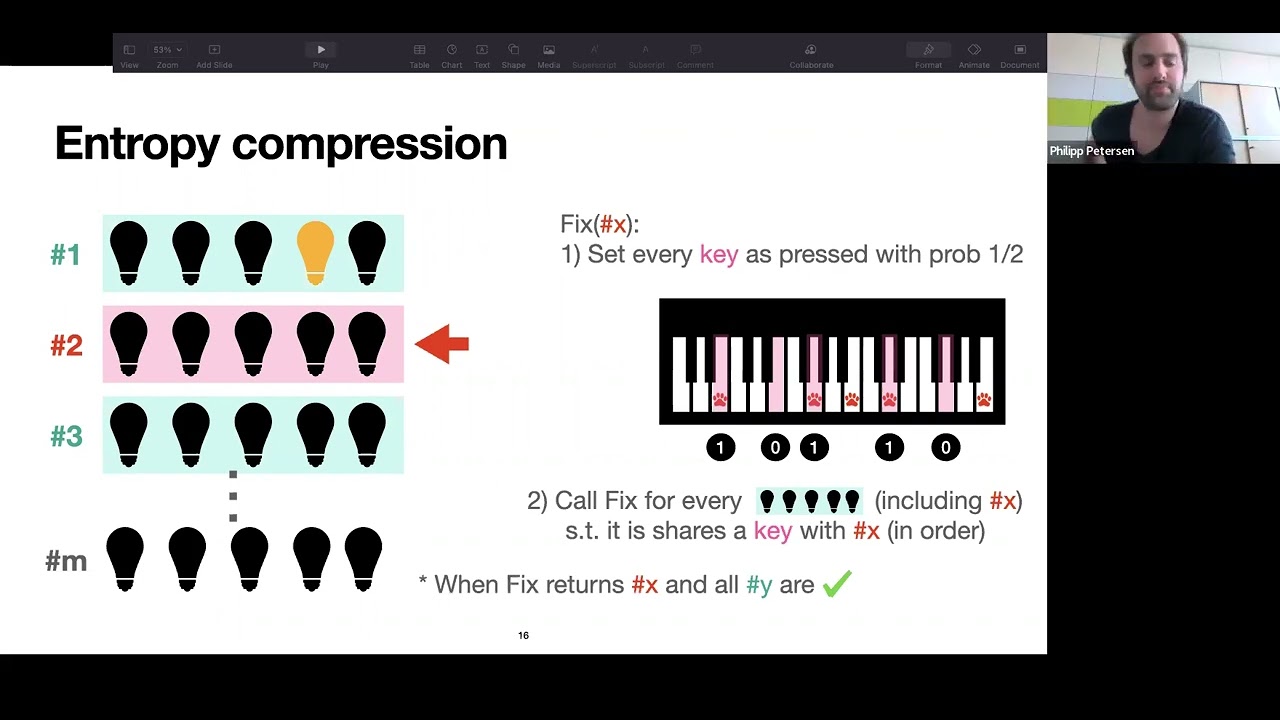
Показать описание
Abstract: In this talk, we present global convergence guarantees for stochastic gradient descent (SGD) via an entropy compression argument. We do so under two main assumptions: (1. Local progress) The model accuracy improves on average over batches. (2. Models compute simple functions) The function computed by the model has low Kolmogorov complexity. It is sufficient that these assumptions hold only for a tiny fraction of the epochs. Intuitively, our results imply that intermittent local progress of SGD implies global progress. Assumption 2 trivially holds for underparameterized models, hence, our work gives the first convergence guarantee for general, underparameterized models. Furthermore, this is the first result that is completely model agnostic - we don't require the model to have any specific architecture or activation function, it may not even be a neural network.