filmov
tv
Advanced Feature Engineering Tips and Tricks - Data Science Festival
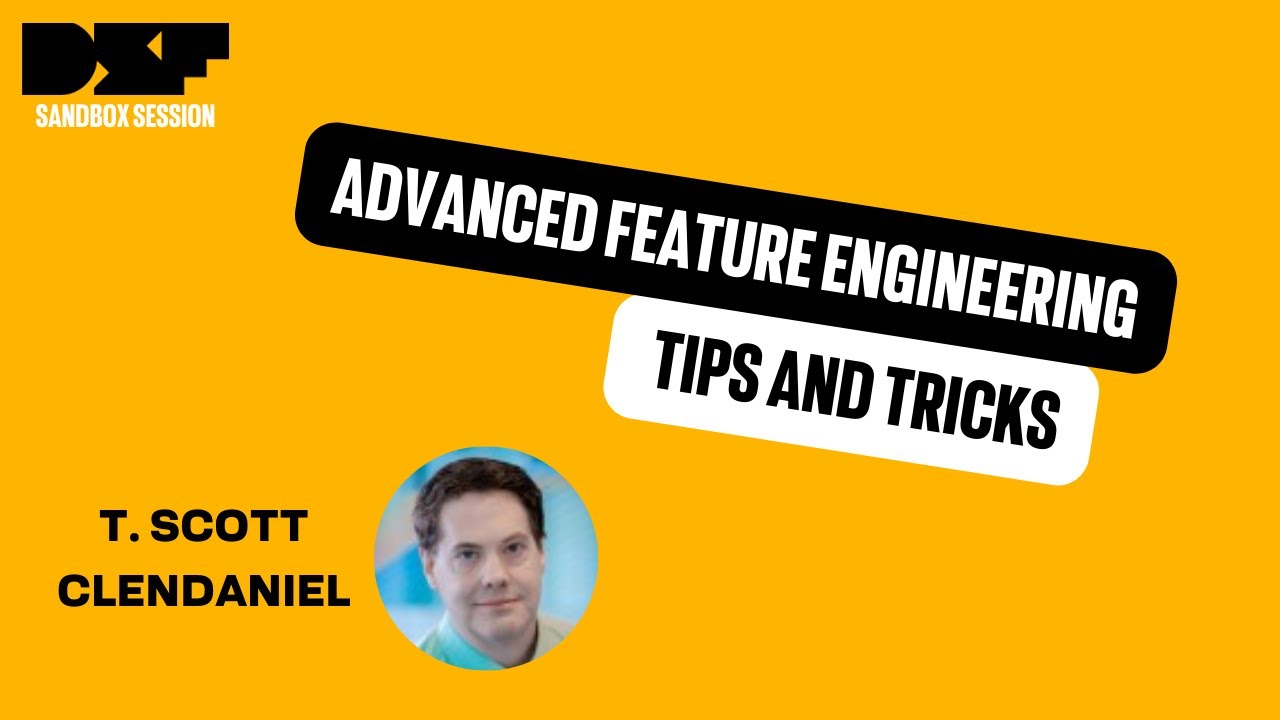
Показать описание
Title: Advanced Feature Engineering Tips and Tricks
Speaker: T. Scott Clendaniel
Abstract: While beginners often focus on algorithm selection, professionals know that the real power in Artificial Intelligence and Machine Learning is often Feature Engineering. Unfortunately, this process can be incredibly time-consuming and complicated. This training will give you a robust set of Tips and Tricks to get the most performance in the shortest time, regardless of the algorithm you choose.
Speaker: T. Scott Clendaniel
Abstract: While beginners often focus on algorithm selection, professionals know that the real power in Artificial Intelligence and Machine Learning is often Feature Engineering. Unfortunately, this process can be incredibly time-consuming and complicated. This training will give you a robust set of Tips and Tricks to get the most performance in the shortest time, regardless of the algorithm you choose.
Advanced Feature Engineering Tips and Tricks - Data Science Festival
Feature Engineering Secret From A Kaggle Grandmaster
Feature Engineering Techniques For Machine Learning in Python
Feature engineering vs Feature Learning (tips tricks 46 )
Intro to Feature Engineering with TensorFlow - Machine Learning Recipes #9
Feature Engineering Full Course - in 1 Hour | Beginner Level
How to use Feature Engineering for Machine Learning, Equations
Kishan Manani - Feature Engineering for Time Series Forecasting | PyData London 2022
Introduction to Feature Engineering in Machine Learning
The Data Hour: An Overview of Feature Engineering for Data Science
Feature Engineering and Deep Learning
Step By Step Process In EDA And Feature Engineering In Data Science Projects
Day in My Life as a Quantum Computing Engineer!
How to think feature engineering | feature engineering tutorial | feature engineering explained
Complete Feature Engineering And EDA In Data Science in 31 Videos
Zipline - A Declarative Feature Engineering Framework
Advance House Price Prediction-Feature Engineering Part 1
Art of Feature Engineering for Data Science - Nabeel Sarwar
Beyond Feature Engineering and HPO | by Jean-François Puget | Kaggle Days Paris
What is Feature Engineering | Feature Engineering Techniques | Feature Engineering | Intellipaat
Automated Feature Engineering and Selection in Python
The Data Hour: Hands-on Workshop on Feature Engineering
Data Science: Quiz Bowl Feature Engineering (11b)
Feature Engineering | Applied Machine Learning, Part 1
Комментарии