filmov
tv
Solving Inverse Problems with Latent Diffusion Models via Hard Data Consistency
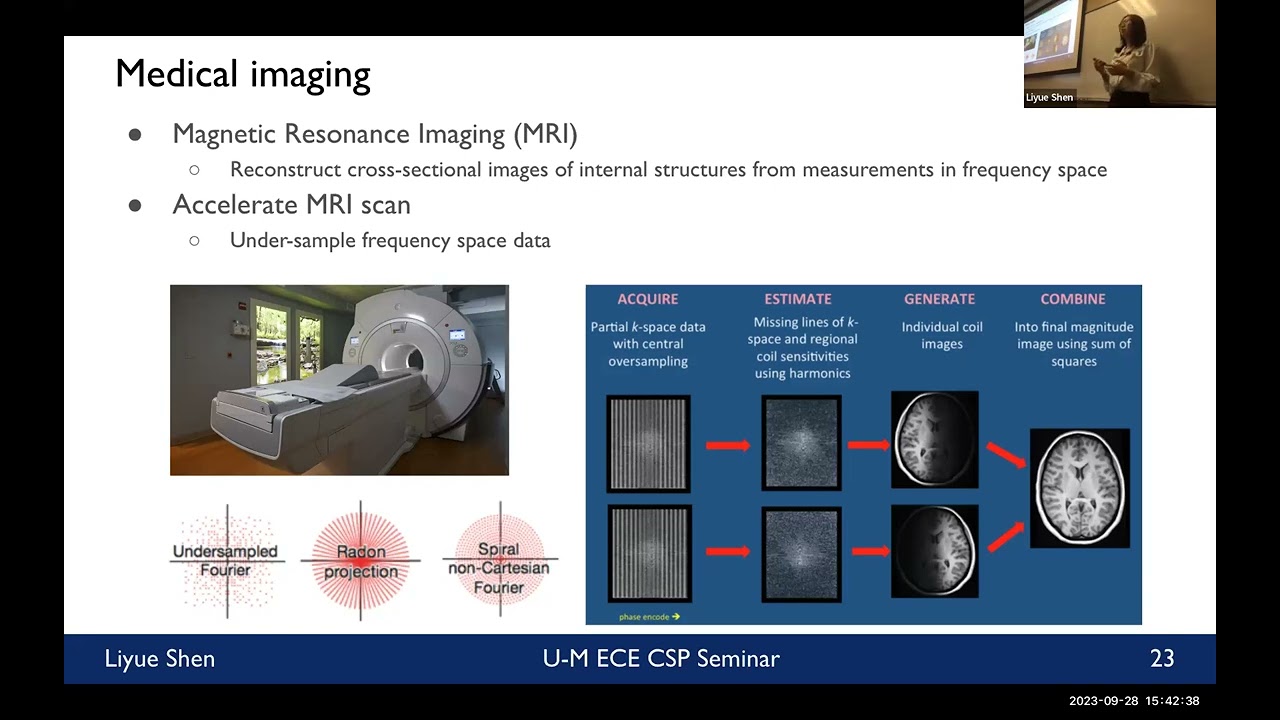
Показать описание
Liyue Shen
Assistant Professor of Electrical and Computer Engineering
University of Michigan, College of Engineering
Abstract: Diffusion models have recently emerged as powerful generative priors for solving inverse problems. However, training diffusion models in the pixel space are data-intensive and computationally demanding, which restricts their applicability in domains such as medical imaging. Latent diffusion models, which operate in a lower-dimensional space, offer a potential solution to these challenges. Though, their direct application to solving inverse problems remains an unsolved technical challenge due to the nonlinearity of the encoder and decoder. In this talk, I will introduce our recently proposed algorithm named ReSample, which can solve general inverse problems with latent diffusion models. This algorithm incorporates data consistency by solving an optimization problem during the reverse sampling process, a concept that we term as hard data consistency. I will show the considerable efficiency and flexibility offered by this approach with high-quality image reconstruction in both linear and non-linear inverse problems, as well as an easy generalization to different domains by simply fine-tuning the pre-trained latent diffusion model with a minimal amount of data samples. Finally, I will provide further discussion about our interesting ongoing works with continuous efforts to develop computationally efficient generative diffusion models for high-resolution and high-dimensional data including 3D and dynamic medical images. This research opens the door to leverage generative diffusion models in tackling complex real-world data for addressing various crucial problems in many scientific disciplines.
Assistant Professor of Electrical and Computer Engineering
University of Michigan, College of Engineering
Abstract: Diffusion models have recently emerged as powerful generative priors for solving inverse problems. However, training diffusion models in the pixel space are data-intensive and computationally demanding, which restricts their applicability in domains such as medical imaging. Latent diffusion models, which operate in a lower-dimensional space, offer a potential solution to these challenges. Though, their direct application to solving inverse problems remains an unsolved technical challenge due to the nonlinearity of the encoder and decoder. In this talk, I will introduce our recently proposed algorithm named ReSample, which can solve general inverse problems with latent diffusion models. This algorithm incorporates data consistency by solving an optimization problem during the reverse sampling process, a concept that we term as hard data consistency. I will show the considerable efficiency and flexibility offered by this approach with high-quality image reconstruction in both linear and non-linear inverse problems, as well as an easy generalization to different domains by simply fine-tuning the pre-trained latent diffusion model with a minimal amount of data samples. Finally, I will provide further discussion about our interesting ongoing works with continuous efforts to develop computationally efficient generative diffusion models for high-resolution and high-dimensional data including 3D and dynamic medical images. This research opens the door to leverage generative diffusion models in tackling complex real-world data for addressing various crucial problems in many scientific disciplines.