filmov
tv
Explaining emergence in NN with model systems analysis - Ekdeep Singh Lubana (PIBBSS Speaker Series)
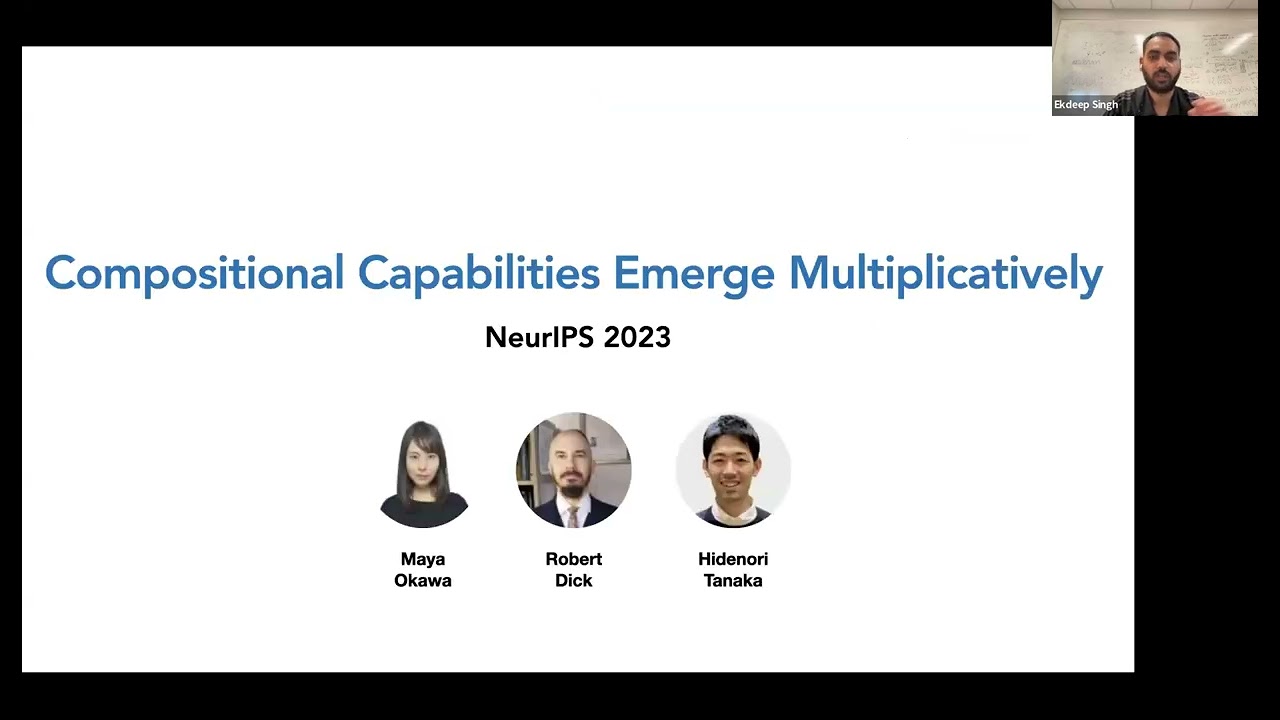
Показать описание
Ekdeep Singh Lubana is a postdoc at Center for Brain Science, Harvard University. Broadly, his research is focused on model systems for identifying novel challenges and better understanding existing challenges in alignment of AI systems. His recent work has revolved around developing mechanistic explanations for emergent capabilities in neural networks and demonstrating the brittleness of fine-tuning based approaches (e.g., RLHF) for alignment.
Explaining emergence in NN with model systems analysis
A fascinating phenomenon often seen in modern neural networks’ training is the sudden emergence of certain capabilities with scale. Specifically, such capabilities seem to be inexistent in the model until a critical amount of compute, data, or model size is reached, showing consistently and controllably thereafter. Since most policy frameworks for AI regulation are grounded in risk regulation, emergent capabilities are a big hurdle for such frameworks: regulating models for capabilities that are not yet present seems likely to be challenging (if not impossible). In this talk, we borrow the approach of model systems analysis from natural sciences to develop mechanistic hypotheses for what leads to the sudden emergence of capabilities in neural networks, identifying several unrelated mechanisms for this effect. These mechanisms have characteristic signatures that indicate preemptive estimation of the scale at which said capabilities will be learned may in fact be feasible.
This talk was recorded in August 2024. For more talks, check out our channel. For information on our talks, subscribe to the mailing list, and see speaker lineups on our website:
Explaining emergence in NN with model systems analysis
A fascinating phenomenon often seen in modern neural networks’ training is the sudden emergence of certain capabilities with scale. Specifically, such capabilities seem to be inexistent in the model until a critical amount of compute, data, or model size is reached, showing consistently and controllably thereafter. Since most policy frameworks for AI regulation are grounded in risk regulation, emergent capabilities are a big hurdle for such frameworks: regulating models for capabilities that are not yet present seems likely to be challenging (if not impossible). In this talk, we borrow the approach of model systems analysis from natural sciences to develop mechanistic hypotheses for what leads to the sudden emergence of capabilities in neural networks, identifying several unrelated mechanisms for this effect. These mechanisms have characteristic signatures that indicate preemptive estimation of the scale at which said capabilities will be learned may in fact be feasible.
This talk was recorded in August 2024. For more talks, check out our channel. For information on our talks, subscribe to the mailing list, and see speaker lineups on our website: