filmov
tv
Christine Solnon Talk - Time-Dependent and Stochastic Optimization for Smart and Sustainable Cities
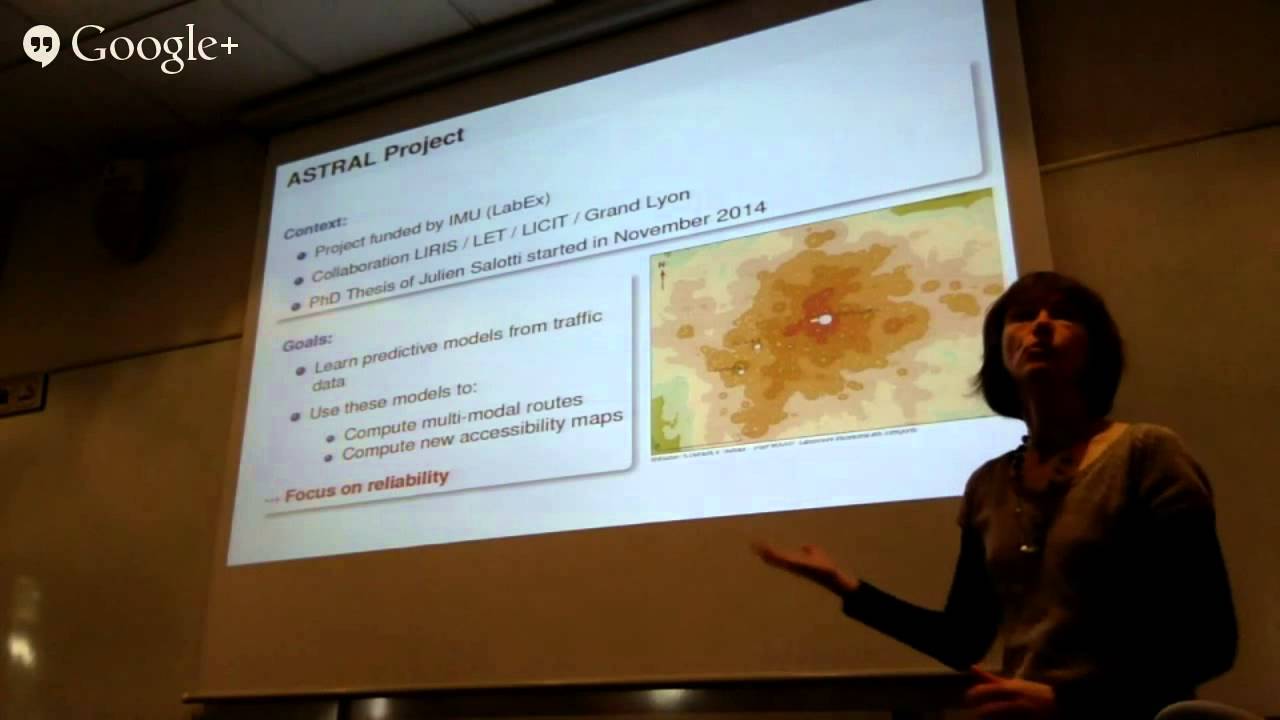
Показать описание
Smart cities are equipped with sensors which monitor many different physical conditions (such as temperature, sound, or traffic speed). These sensor networks generate huge data flows which may be leveraged to optimize resource consumption and, more generally, drive sustainable economic growth for citizens. This talk focuses on two challenging optimization problems that occur in this context.
Time-Dependent Optimization: Sensor networks generate data flows which may be used to design time-dependent predictive models. In the context of the Optimod’Lyon project, for example, we had access to real traffic data measured from 630 sensors for 6 years. Given this data, a time-dependent predictive model has been built, which predicts speeds on every street section, by 6-minute time steps. We use this time-dependent predictive model to optimize urban deliveries. We show that this allows us to compute better solutions. However, optimizing deliveries with time-dependent data is also more difficult to achieve and classical approaches do not scale well. Therefore, we introduce new optimization tools able to deal with time-dependent data. This work is done in collaboration with the IBM and the Grand Lyon.
Stochastic Optimization: Predicted data may simply be composed of mean values, estimated w.r.t. data flows monitored by sensors under similar conditions (e.g., same time window with similar weather conditions). However, in many real-world applications, we search not only for good solutions with respect to mean predicted data, but also for flexible ones, i.e., solutions that may be dynamically adapted when real data (revealed at real-time) is different from the predicted one. Therefore, we introduce new algorithms able to compute flexible solutions with respect to stochastic variables, and able to dynamically adapt these solutions when these stochastic variables are revealed (during the online execution of the solution). This work is done in collaboration with the Université Catholique de Louvain (Belgium).
Time-Dependent Optimization: Sensor networks generate data flows which may be used to design time-dependent predictive models. In the context of the Optimod’Lyon project, for example, we had access to real traffic data measured from 630 sensors for 6 years. Given this data, a time-dependent predictive model has been built, which predicts speeds on every street section, by 6-minute time steps. We use this time-dependent predictive model to optimize urban deliveries. We show that this allows us to compute better solutions. However, optimizing deliveries with time-dependent data is also more difficult to achieve and classical approaches do not scale well. Therefore, we introduce new optimization tools able to deal with time-dependent data. This work is done in collaboration with the IBM and the Grand Lyon.
Stochastic Optimization: Predicted data may simply be composed of mean values, estimated w.r.t. data flows monitored by sensors under similar conditions (e.g., same time window with similar weather conditions). However, in many real-world applications, we search not only for good solutions with respect to mean predicted data, but also for flexible ones, i.e., solutions that may be dynamically adapted when real data (revealed at real-time) is different from the predicted one. Therefore, we introduce new algorithms able to compute flexible solutions with respect to stochastic variables, and able to dynamically adapt these solutions when these stochastic variables are revealed (during the online execution of the solution). This work is done in collaboration with the Université Catholique de Louvain (Belgium).