filmov
tv
Scaling Write-Intensive Key-Value Stores
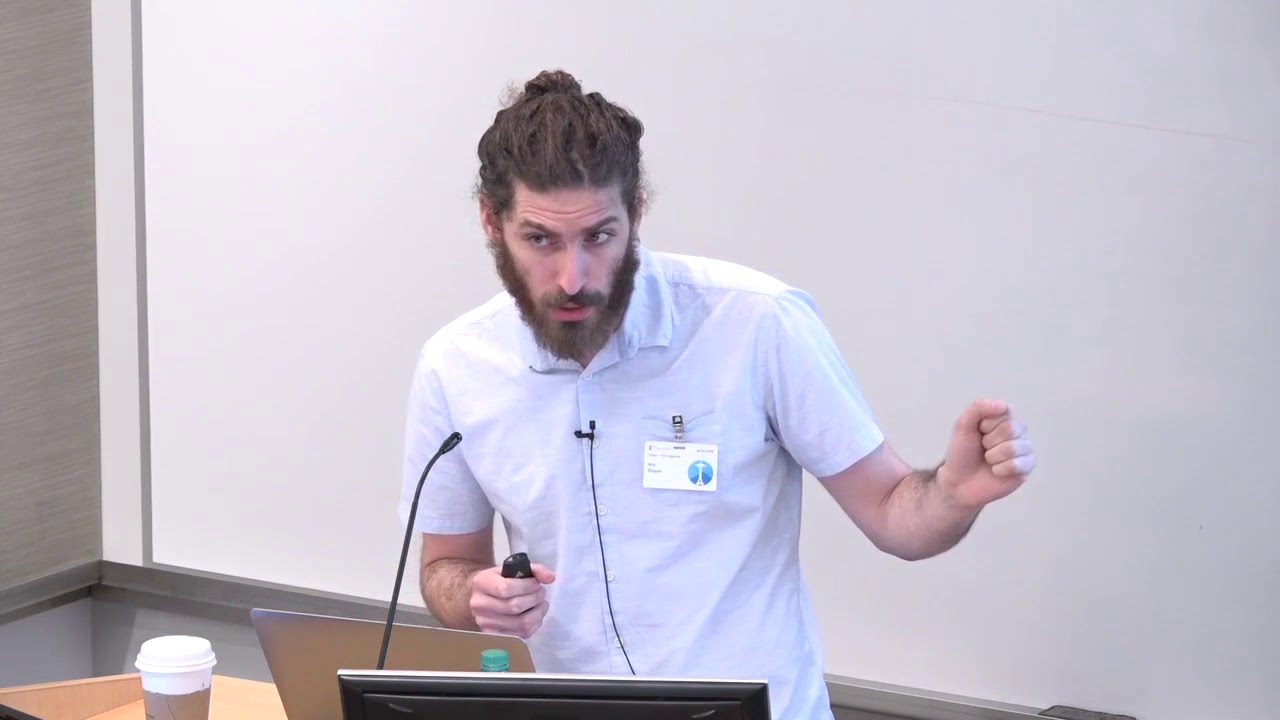
Показать описание
In recent years, the log-structured merge-tree (LSM-tree) has become the mainstream core data structure used by key-value stores to ingest and persist data quickly. LSM-tree enables fast writes by buffering incoming data in memory and flushing it as independent sorted batches to storage whenever the buffer is full. To enable fast reads, LSM-tree sort-merges batches in storage to restrict the number that reads have to search, and it also uses in-memory Bloom filters to enable point reads to probabilistically skip accessing batches that do not contain a target entry. In this talk, we show that such LSM-tree based designs do not scale well: as the data size increases, both reads and writes take increasingly long to execute. We pinpoint the problem to suboptimal core design: the Bloom filters have been attached to LSM-tree as an afterthought and are therefore not optimized to minimize the overall probability of access to storage. Point reads are therefore unnecessarily expensive. To compensate, more merging than necessary has to take place thereby making writes unnecessarily expensive.
As a part of the CrimsonDB project at the Harvard DasLab, we developed two insights to address this problem. Firstly, we show that the optimal approach for allocating Bloom filters given any amount of available memory resources is to assign significantly lower false positive rates to smaller data batches. This shaves a logarithmic factor from point read cost thereby allowing key-value stores to scale better in terms of reads. Secondly, having lower false positive rates for smaller batches allows to merge newer data more lazily without compromising point read cost. This allows eliminating most of the merge overheads of LSM-tree thereby improving the scalability of writes. We close by describing a higher-level lessons from our work: while data structure design up until today has focused on the cost balance between reads and writes, the inclusion of memory utilization as a direct additional optimization objective opens up new avenues for asymptotic improvements, which studying reads and writes in isolation could not have revealed.
As a part of the CrimsonDB project at the Harvard DasLab, we developed two insights to address this problem. Firstly, we show that the optimal approach for allocating Bloom filters given any amount of available memory resources is to assign significantly lower false positive rates to smaller data batches. This shaves a logarithmic factor from point read cost thereby allowing key-value stores to scale better in terms of reads. Secondly, having lower false positive rates for smaller batches allows to merge newer data more lazily without compromising point read cost. This allows eliminating most of the merge overheads of LSM-tree thereby improving the scalability of writes. We close by describing a higher-level lessons from our work: while data structure design up until today has focused on the cost balance between reads and writes, the inclusion of memory utilization as a direct additional optimization objective opens up new avenues for asymptotic improvements, which studying reads and writes in isolation could not have revealed.
Комментарии