filmov
tv
Data Science, Financial Services, Insurance, and Innovation (CXOTalk
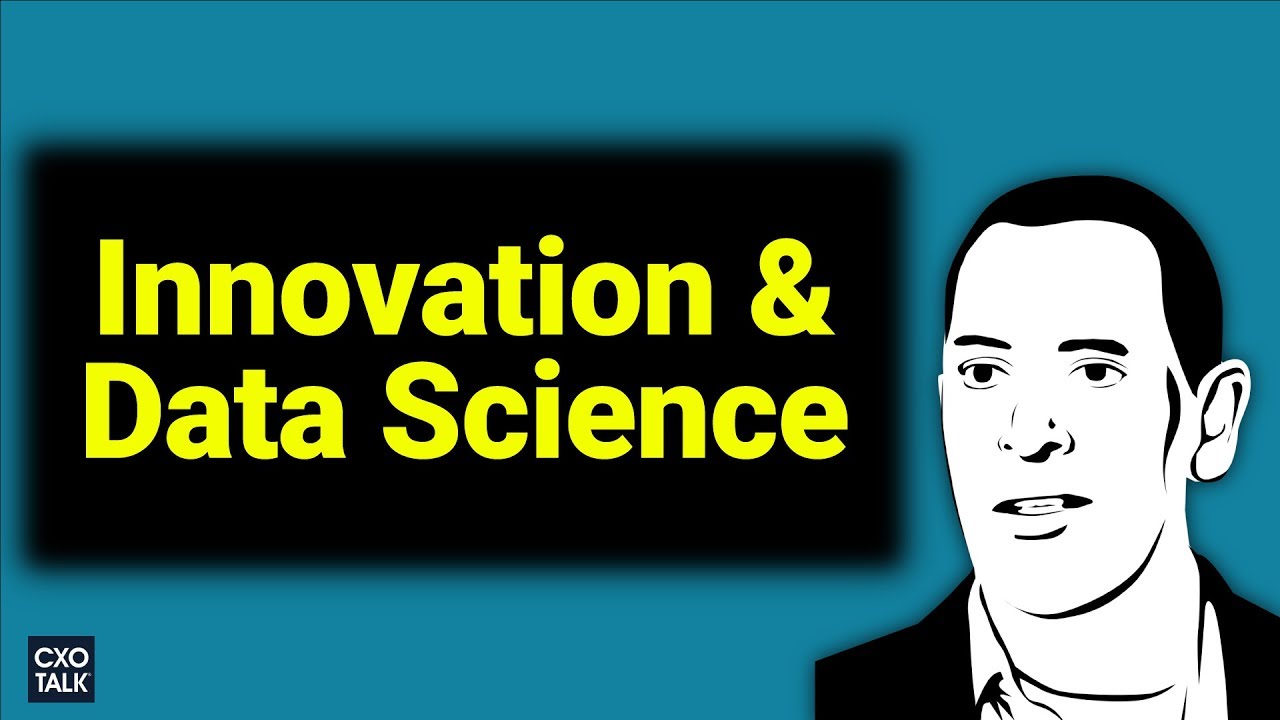
Показать описание
Pervasive data science is becoming a core enabler of business innovation and competition. Given this importance, it's worth examining the context of data science to consider its trajectory and future value.
Murli Buluswar, former Chief Science Officer at AIG, speaks with CXOTalk co-hosts Michael Li, CEO of The Data Incubator, and Michael Krigsman
Primary challenges around data science for business leaders come from three sources:
1. Business people do not always understand the power and implications of what's possible with data science and machine learning. The impact on business models, operations, and customers can be profound.
2. Lack of available data to feed the machine learning beast. Without historical or other data, machine learning has little value. Aggregating useful data can be expensive and time-consuming.
3. Insufficient talent and resources to create models and set up analyses that can best serve users and customers. Making effective use of data science demands a different kind of thought than traditional analytics; even a culture shift, so it's hard for established organizations to adapt quickly.
From the transcript:
Murli Buluswar: To build on what you just said, Michael, if you were to contextualize that to insurance, I see the big leap in innovation happening in the next two to three years around this notion of making more granular, real-time decisions with machine learning.
By defining data not just in the traditional internal structured terms, but thinking of it in four quadrants: internal / external on one dimension and structured / unstructured on the other dimension.
The ability to build machine learning algorithms on these platforms will reshape what humans do with decision-making and judgment and where models harmonize or balance human judgment with machine intelligence.
Often, people think of it as an either/or. But if you re-paraphrase machine intelligence as nothing but the collective experience of the institution manifested through data, it brings consistency and granularity to decision-making.
That's not to say that it obviates the role of human judgment completely, but it is to say that that balance, that harmony should and will look dramatically different two years, three years from now than it has for the last decade and before that.
The next big step-change that I see for this sector as a whole is evolving from a predictor of risk to an actual risk partner that can mitigate outcomes through the power of real-time insights.
The most obvious example of that is the role that sensors can play in providing real-time feedback to drivers of vehicles in a way that hopefully reduces risky driving and mitigates the likelihood of accidents. To me, that is the true power of data science in insurance.
Not only does it mitigate accidents from happening, or adverse events from happening, but reduces the cost of insurance and expands the reach of protection to a much broader population, both in the developed and developing worlds. To me, that's a beautiful thing if you think about society having a much higher level of financial protection across every aspect of our lives.
Michael Li: Think about what's new in data science, that is, why is data science different from or how does data science expand upon things like the actuarial tradition, like statisticians, the quants of yore.
We're no longer just using structured data, so it's not just SQL queries anymore. It's now semi-structured and unstructured data. How do you start handling things when they don't come in nice tables that you can load into Excel or that you can put into SQL?
We are also in a world where data is much larger. You mentioned telematics. If you took a reading from every car every second, that's a lot of numbers you've got to store, and that's a very different paradigm for computation. You have to think about storing this data. How do you deal with data now that it's stored across multiple computers? How do you think about computation in that context?
Then, of course, the last thing is real-time data. Analytics has historically been -- you might call it -- a batch process. Run it once; generate a report; show it to people; you're done.
Now it's a continuous process. You run it; you have to instantly find the latest trends; put that into production so you can intelligently adapt; and then do it again the next hour, the next minute. That's where competition is driving you.
If you look at what Silicon Valley has been doing, it is very much your server is constantly learning from user behavior and adjusts how it interacts with users in a way that -- to borrow an expression -- delights the user. I think that we see that.
Traditional companies, non-tech-based companies, have to emulate that kind of level of customer service and satisfaction. A lot of that comes down to big data and having a team capable of understanding new and different kinds of data in a world that's rapidly evolving.
Murli Buluswar, former Chief Science Officer at AIG, speaks with CXOTalk co-hosts Michael Li, CEO of The Data Incubator, and Michael Krigsman
Primary challenges around data science for business leaders come from three sources:
1. Business people do not always understand the power and implications of what's possible with data science and machine learning. The impact on business models, operations, and customers can be profound.
2. Lack of available data to feed the machine learning beast. Without historical or other data, machine learning has little value. Aggregating useful data can be expensive and time-consuming.
3. Insufficient talent and resources to create models and set up analyses that can best serve users and customers. Making effective use of data science demands a different kind of thought than traditional analytics; even a culture shift, so it's hard for established organizations to adapt quickly.
From the transcript:
Murli Buluswar: To build on what you just said, Michael, if you were to contextualize that to insurance, I see the big leap in innovation happening in the next two to three years around this notion of making more granular, real-time decisions with machine learning.
By defining data not just in the traditional internal structured terms, but thinking of it in four quadrants: internal / external on one dimension and structured / unstructured on the other dimension.
The ability to build machine learning algorithms on these platforms will reshape what humans do with decision-making and judgment and where models harmonize or balance human judgment with machine intelligence.
Often, people think of it as an either/or. But if you re-paraphrase machine intelligence as nothing but the collective experience of the institution manifested through data, it brings consistency and granularity to decision-making.
That's not to say that it obviates the role of human judgment completely, but it is to say that that balance, that harmony should and will look dramatically different two years, three years from now than it has for the last decade and before that.
The next big step-change that I see for this sector as a whole is evolving from a predictor of risk to an actual risk partner that can mitigate outcomes through the power of real-time insights.
The most obvious example of that is the role that sensors can play in providing real-time feedback to drivers of vehicles in a way that hopefully reduces risky driving and mitigates the likelihood of accidents. To me, that is the true power of data science in insurance.
Not only does it mitigate accidents from happening, or adverse events from happening, but reduces the cost of insurance and expands the reach of protection to a much broader population, both in the developed and developing worlds. To me, that's a beautiful thing if you think about society having a much higher level of financial protection across every aspect of our lives.
Michael Li: Think about what's new in data science, that is, why is data science different from or how does data science expand upon things like the actuarial tradition, like statisticians, the quants of yore.
We're no longer just using structured data, so it's not just SQL queries anymore. It's now semi-structured and unstructured data. How do you start handling things when they don't come in nice tables that you can load into Excel or that you can put into SQL?
We are also in a world where data is much larger. You mentioned telematics. If you took a reading from every car every second, that's a lot of numbers you've got to store, and that's a very different paradigm for computation. You have to think about storing this data. How do you deal with data now that it's stored across multiple computers? How do you think about computation in that context?
Then, of course, the last thing is real-time data. Analytics has historically been -- you might call it -- a batch process. Run it once; generate a report; show it to people; you're done.
Now it's a continuous process. You run it; you have to instantly find the latest trends; put that into production so you can intelligently adapt; and then do it again the next hour, the next minute. That's where competition is driving you.
If you look at what Silicon Valley has been doing, it is very much your server is constantly learning from user behavior and adjusts how it interacts with users in a way that -- to borrow an expression -- delights the user. I think that we see that.
Traditional companies, non-tech-based companies, have to emulate that kind of level of customer service and satisfaction. A lot of that comes down to big data and having a team capable of understanding new and different kinds of data in a world that's rapidly evolving.