filmov
tv
Parallel Programming with (Py)OpenCL for Fun and Profit
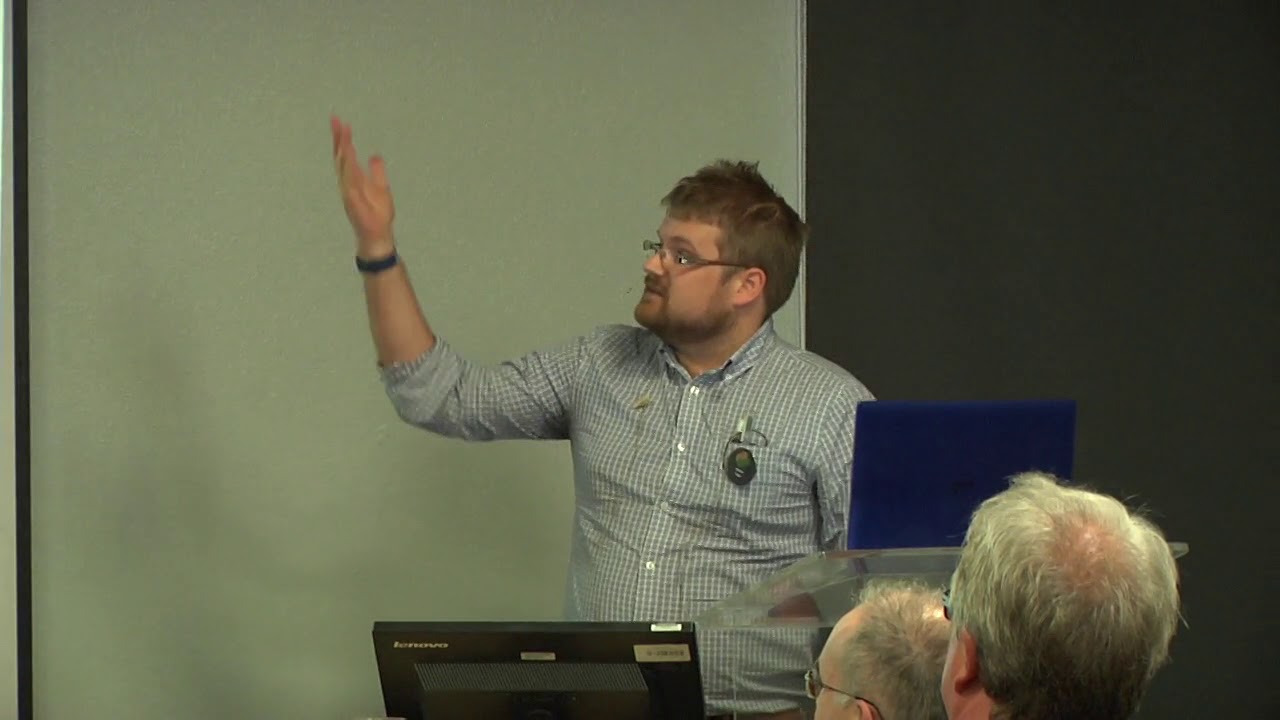
Показать описание
Gordon Inggs
## Overview
In this talk, I will introduce the basics of the OpenCL programming and runtime APIs, using examples run in Jupyter notebooks on a variety of devices. I will also help identify the situations where it makes sense to accelerate portions of a codebase.
## Audience
This talk is aimed at anyone who loves the expressiveness of Python, but has bumped into its performance limitations. I assume no background in HPC and/or heterogeneous computing, and will be using simple, yet hopefully relevant examples such as fundamental linear algebra and analysis applications.
By the end of the talk, provided it isn't a post-lunch slot, the audience should be ready to identify the hotspots in their code, and start accelerating using the CPUs, GPUs and FPGAs in their laptops and favourite public clouds such as AWS, Azure and GCE.
pyconza2018
python
## Overview
In this talk, I will introduce the basics of the OpenCL programming and runtime APIs, using examples run in Jupyter notebooks on a variety of devices. I will also help identify the situations where it makes sense to accelerate portions of a codebase.
## Audience
This talk is aimed at anyone who loves the expressiveness of Python, but has bumped into its performance limitations. I assume no background in HPC and/or heterogeneous computing, and will be using simple, yet hopefully relevant examples such as fundamental linear algebra and analysis applications.
By the end of the talk, provided it isn't a post-lunch slot, the audience should be ready to identify the hotspots in their code, and start accelerating using the CPUs, GPUs and FPGAs in their laptops and favourite public clouds such as AWS, Azure and GCE.
pyconza2018
python
Parallel Programming with (Py)OpenCL for Fun and Profit
CUDA Simply Explained - GPU vs CPU Parallel Computing for Beginners
Nvidia CUDA in 100 Seconds
What Is The Parallel Profits Program - Parallel Programming With (Py)Opencl For Fun And Profit
CUDA in your Python: Effective Parallel Programming on the GPU
CUDA in your Python Parallel Programming on the GPU - William Horton
William Horton: CUDA in Your Python: Effective Parallel Programming on the GPU
Intro to CUDA - An introduction, how-to, to NVIDIA's GPU parallel programming architecture
William Horton - CUDA in your Python: Effective Parallel Programming on the GPU - PyCon 2019
Hands-On GPU Computing with Python | 7. Working with ROCm and PyOpenCL
Episode 1: What is OpenCL™?
Local and Global Dimensions in OpenCL (3)
OpenCL for FPGA and Data Parallel Kernel
Purely Functional GPU Programming with Futhark
Pierre Glaser - Parallel computing in Python: Current state and recent advances
Programacao Paralela: OpenCL (demo)
CUDA Programming Course – High-Performance Computing with GPUs
ATI Stream OpenCL™ Technical Overview [Part 1] - What is OpenCL™?
Parallel Programming with Python (PyCUDA and Dask) - 2
High-Performance Computing with Python: Numba and GPUs
Python Parallel Programming Solutions [Video Course]
Directive Based Parallel programming on GPU
OpenCL OpenGL and Python
Parallel Programming with Python(PyCUDA and Dask)
Комментарии