filmov
tv
Causal Probabilistic Programming: Automating Reasoning In Simulation Models
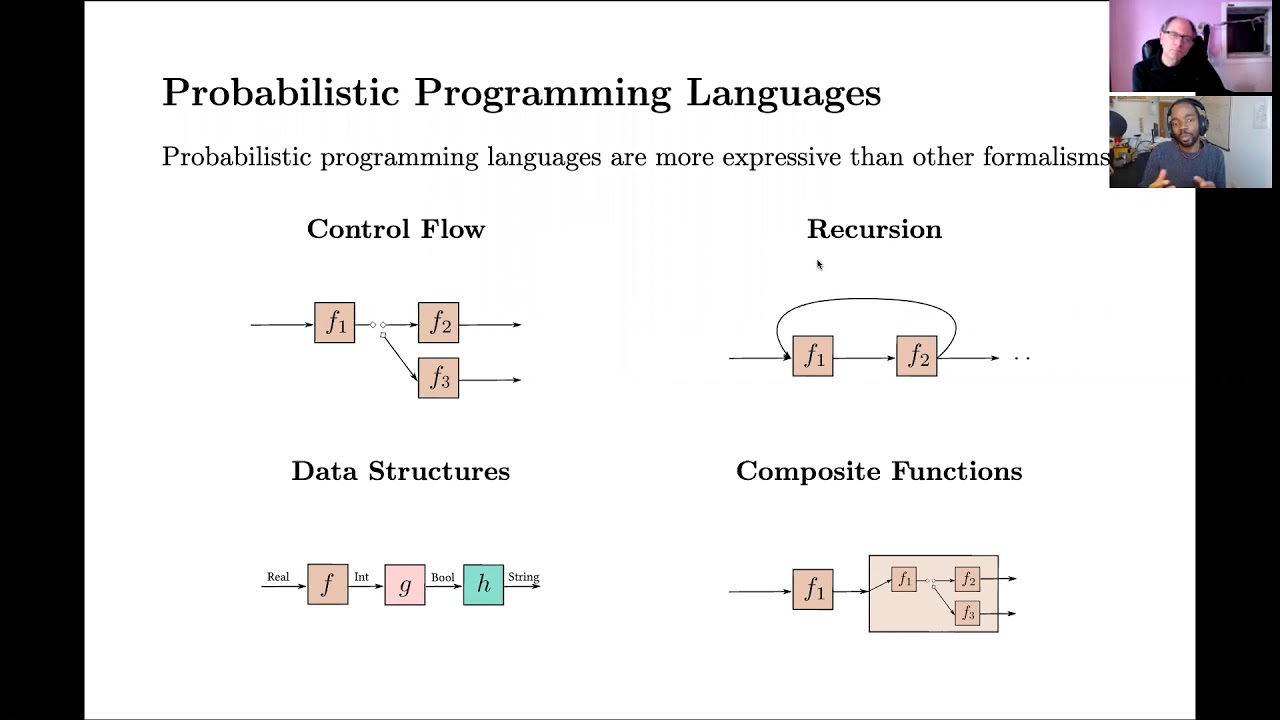
Показать описание
Causal Probabilistic Programming: Automating Reasoning In Simulation Models
Guest Speaker: Zenna Tavares, Postdoctoral Researcher, MIT
Abstract: Humans mentally model the causal structure of the world, and manipulate these models to reason: we infer plausible causes of events, construct explanations, and imagine worlds that could have been but never were. Humans build these models from very small amounts of data, both passively observed and actively sought through experimentation. In this talk, Zenna Tavares will present efforts to provide computational accounts of these remarkable feats of inference, and make the case that this offers a promising approach to address hard problems in data science and artificial intelligence.
The talk will propose that both tacit and explicit knowledge can be encoded as probabilistic programs, and that reasoning is performed by manipulating these programs. Tavares will present methods for probabilistic and causal inference within this framework, which allow us to answer what-if counterfactual queries in complex simulation models. Finally, the talk will sketch preliminary work on program induction — algorithms that infer the model structure itself from experimental and observational data.
Guest Speaker: Zenna Tavares, Postdoctoral Researcher, MIT
Abstract: Humans mentally model the causal structure of the world, and manipulate these models to reason: we infer plausible causes of events, construct explanations, and imagine worlds that could have been but never were. Humans build these models from very small amounts of data, both passively observed and actively sought through experimentation. In this talk, Zenna Tavares will present efforts to provide computational accounts of these remarkable feats of inference, and make the case that this offers a promising approach to address hard problems in data science and artificial intelligence.
The talk will propose that both tacit and explicit knowledge can be encoded as probabilistic programs, and that reasoning is performed by manipulating these programs. Tavares will present methods for probabilistic and causal inference within this framework, which allow us to answer what-if counterfactual queries in complex simulation models. Finally, the talk will sketch preliminary work on program induction — algorithms that infer the model structure itself from experimental and observational data.