filmov
tv
Artificial Intelligence for Geometry Processing (Rana Hanocka, Tel Aviv University)
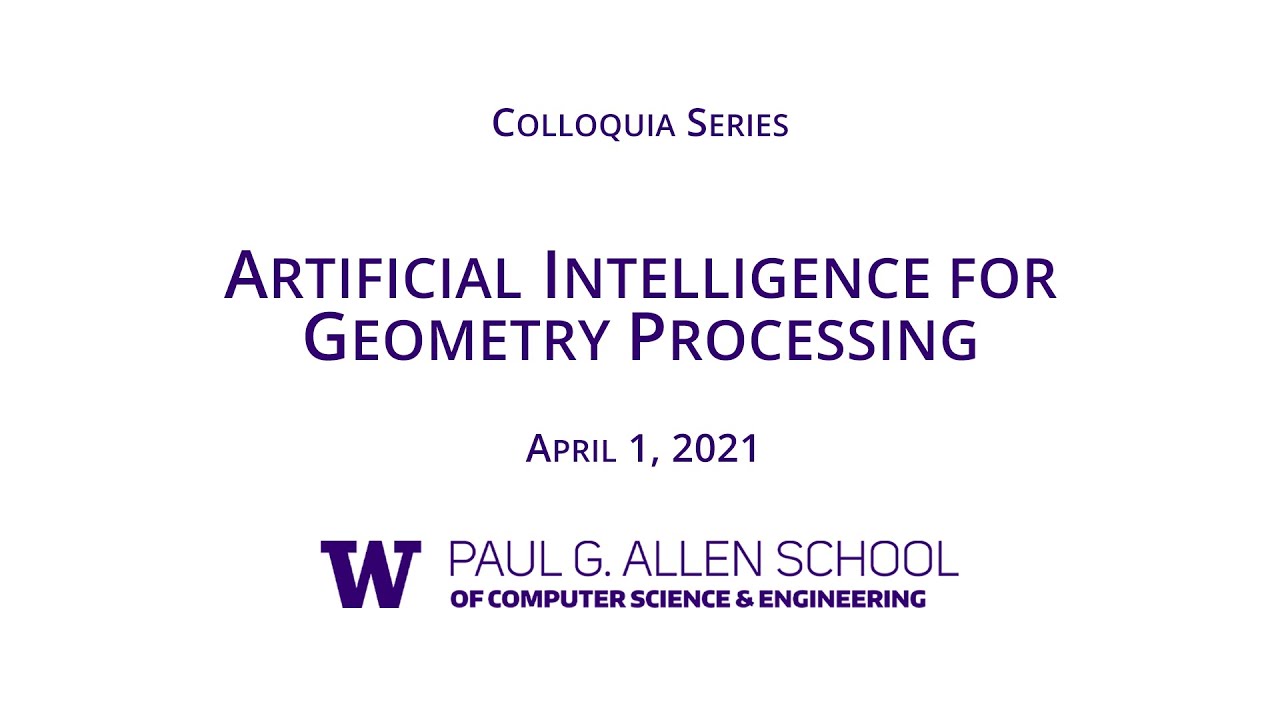
Показать описание
Spring 2021 Allen School Colloquium
Demand for geometry processing is higher than ever, given the continuously and exponentially growing amount of captured 3D data (with depth-sensing cameras now prevalent in smartphones, robots, drones, and cars). Yet, in practice, current geometry processing techniques struggle to automatically and robustly analyze real-world data, even in small volumes. Deep learning, the most popular form of artificial intelligence, has been remarkably effective in extracting patterns from voluminous data, thus generating significant scientific interest in its applicability to 3D geometric data. However, despite the inspiring success of deep learning on large sets of Euclidean data (such as text, images, and video), extending deep neural networks to non-Euclidean, irregular 3D data has proven to be both ambiguous and highly challenging. This talk will present my research into developing deep learning techniques that enable effective operation on irregular geometric data. I will demonstrate how we can leverage the representational power of neural networks to solve complex geometry processing problems, including surface reconstruction and geometric modeling/synthesis. I will conclude by highlighting open research directions aligned with my focus on designing 3D machine learning techniques that can both facilitate the robust processing of real-world geometric data and improve ease-of-use in downstream applications.
This lecture was recorded on April 1, 2021 and is closed captioned.
Demand for geometry processing is higher than ever, given the continuously and exponentially growing amount of captured 3D data (with depth-sensing cameras now prevalent in smartphones, robots, drones, and cars). Yet, in practice, current geometry processing techniques struggle to automatically and robustly analyze real-world data, even in small volumes. Deep learning, the most popular form of artificial intelligence, has been remarkably effective in extracting patterns from voluminous data, thus generating significant scientific interest in its applicability to 3D geometric data. However, despite the inspiring success of deep learning on large sets of Euclidean data (such as text, images, and video), extending deep neural networks to non-Euclidean, irregular 3D data has proven to be both ambiguous and highly challenging. This talk will present my research into developing deep learning techniques that enable effective operation on irregular geometric data. I will demonstrate how we can leverage the representational power of neural networks to solve complex geometry processing problems, including surface reconstruction and geometric modeling/synthesis. I will conclude by highlighting open research directions aligned with my focus on designing 3D machine learning techniques that can both facilitate the robust processing of real-world geometric data and improve ease-of-use in downstream applications.
This lecture was recorded on April 1, 2021 and is closed captioned.