filmov
tv
Neural ODEs in Hydrology | Marvin Höge | SciMLCon 2022
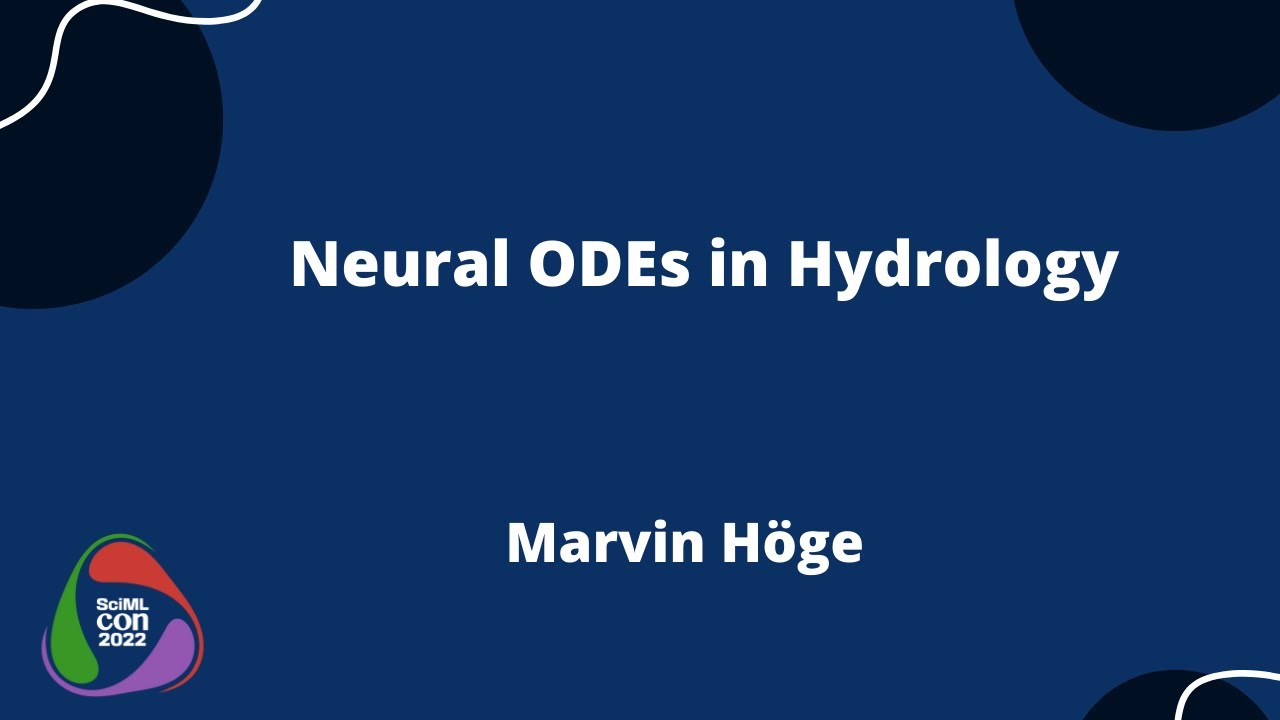
Показать описание
Neural ODEs in Hydrology | Marvin Höge | SciMLCon 2022
Abstract:
For stream flow predictions in hydrology commonly two types of models are used: deep learning models (high predictive performance) and ODE-based conceptual hydrologic models (fully interpretable, encoding scientific assumptions). We introduce hydrologic Neural ODE models that fuse both approaches and have all benefits: We obtain state-of the-art predictive performance and gain insights into the dynamics of model processes and states. We demonstrate the approach on a large real-world data set.
Description:
Authors: Marvin Höge, Andreas Scheidegger, Marco Baity-Jesi, Carlo Albert, and Fabrizio Fenicia
Affiliation: Department of Systems Analysis, Integrated Assessment and Modelling, Eawag, Dübendorf, Switzerland
Deep learning models, in particular long-short-term-memory (LSTM) neural networks, have outperformed traditionally-used conceptual hydrologic models in streamflow prediction over recent years. Conceptual models based on ordinary differential equations (ODEs) which makes them fully interpretable. Since system understanding is just as important in hydrology, this has always been considered to be an asset of this model type. Conceptual hydrologic models provide direct interpretability of model states and processes and allow for physical inter- and extrapolations, e.g., over temperature ranges. For example, this is particularly important for investigating hydrologic implications of climate change and extreme conditions.
Despite their high predictive accuracy, LSTMs show limitations that are particularly relevant in hydrology: First, although progress in investigating the internals of LSTMs is being made, they remain hardly traceable and difficult to interpret. Second, physical principles like time continuity and mass balance are not given as they are with ODE-based models. Third, prior knowledge like river catchment-specific conditions cannot be easily included. Therefore, conceptual models often remain the first choice in the hydrologic community despite having lower predictive accuracy.
We introduce hydrologic Neural ODE models that show similar or better predictive performance compared to state-of-the-art deep learning method while not having the shortcomings of other deep learning methods: They are as easily interpretable as conceptual hydrologic models, they are naturally continuous in time and account for mass balance, and they allow to include specific knowledge into the model. Therefore, the NeuralODE approach offers a way to fuse deep learning with mechanistic modelling that alleviates the deficits of both approaches.
We have built our models using a simple conceptual hydrologic model as baseline. From there, we developed two Neural ODE models: One in which only a few processes are substituted by neural networks, and one in which all processes are substituted. For comparison, we evaluate all three models in direct conjunction.
We demonstrate the high predictive accuracy of hydrologic Neural ODE models for real-word data from a public large-sample hydrologic data set. The data set covers several hundred catchments in the continental USA and comprises of the stream flow data as well as meteorological input forcings like precipitation and temperature. Our models are trained and tested individually on each considered catchment and rated by metrics like Nash-Sutcliffe efficiency that are commonly used in hydrology.
Further, we give insight to what the model-internal neural networks have learned about the model processes for selected catchments. We analyze the processes on discharge generation, evapotranspiration and melting and compare them to the dynamics of the hard-coded processes of the baseline conceptual model.
00:00 Welcome!
00:10 Help us add time stamps or captions to this video! See the description for details.
Комментарии