filmov
tv
Importance of Activation Functions in Neural Networks | Deep Learning basics
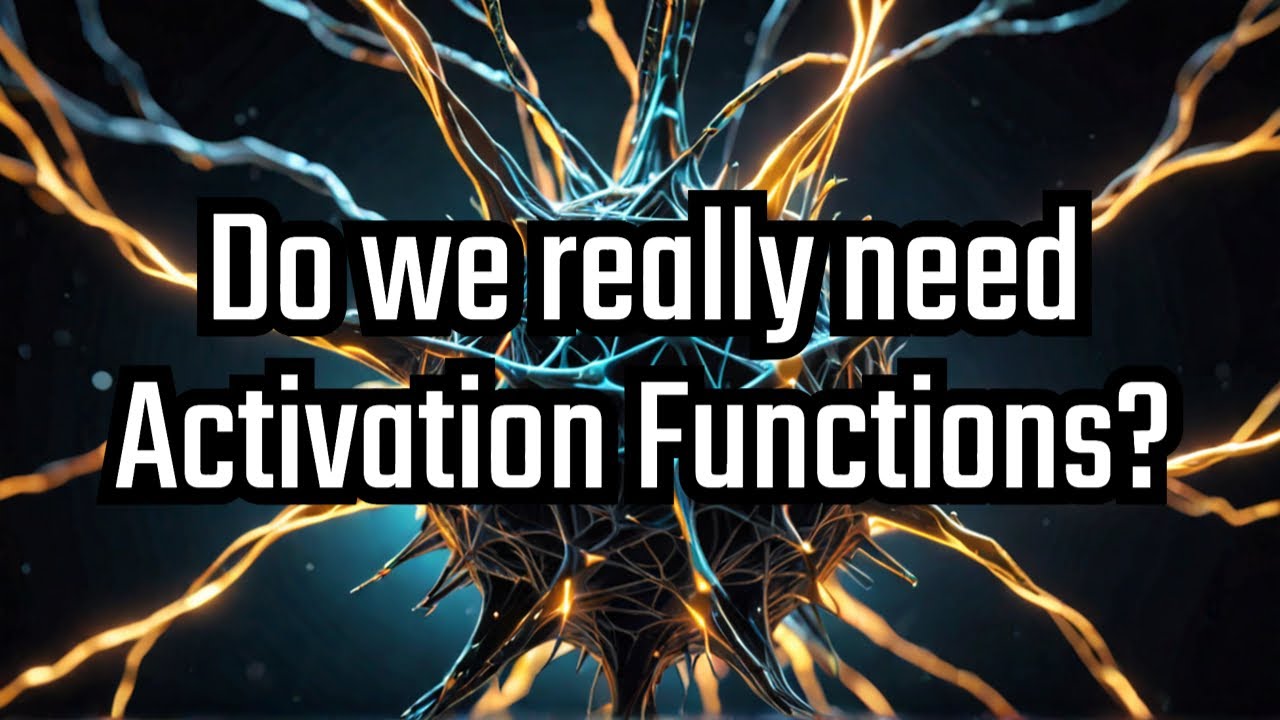
Показать описание
🔍 Have you ever wondered why we use activation functions in neural networks? In this video, we'll explain it in the simplest possible way with a relatable example.
🤔 Envision, a simple neural network with two input neurons, three hidden neurons, and a lone output neuron. In this example, the activation functions have been deliberately omitted from both the hidden and output layers. 😲
🔌 Through step-by-step methodical scrutiny, we will explain the calculations at each juncture, right from the input neurons to the outpus neuron. 💡
💡 We'll take a closer look at how the connections between neurons work, assigning weights to each one. By doing some math, we'll see how signals combine at each neuron. It's like putting together puzzle pieces!
Surprisingly, we'll find out that we can get the same result using just one neuron, but with the right weights. So, why bother with all those extra layers? 🤷♂️
🚫 Well, here's the catch: that single neuron can only handle simple data patterns. When things get more complex, it struggles. Think of it like trying to solve a really hard puzzle with only a few pieces!.
💥 But don't worry! Non-linear activation functions come to the rescue! They help us deal with all kinds of complex data patterns, making our neural network super powerful.
There's just one exception: the output layer. For certain problems, like figuring out trends in data, a simpler approach works fine.
So, get ready as we'll show you how activation functions work their magic, unlocking the mysteries of this fascinating technology. Let's go! 🚀
🤔 Envision, a simple neural network with two input neurons, three hidden neurons, and a lone output neuron. In this example, the activation functions have been deliberately omitted from both the hidden and output layers. 😲
🔌 Through step-by-step methodical scrutiny, we will explain the calculations at each juncture, right from the input neurons to the outpus neuron. 💡
💡 We'll take a closer look at how the connections between neurons work, assigning weights to each one. By doing some math, we'll see how signals combine at each neuron. It's like putting together puzzle pieces!
Surprisingly, we'll find out that we can get the same result using just one neuron, but with the right weights. So, why bother with all those extra layers? 🤷♂️
🚫 Well, here's the catch: that single neuron can only handle simple data patterns. When things get more complex, it struggles. Think of it like trying to solve a really hard puzzle with only a few pieces!.
💥 But don't worry! Non-linear activation functions come to the rescue! They help us deal with all kinds of complex data patterns, making our neural network super powerful.
There's just one exception: the output layer. For certain problems, like figuring out trends in data, a simpler approach works fine.
So, get ready as we'll show you how activation functions work their magic, unlocking the mysteries of this fascinating technology. Let's go! 🚀
Комментарии