filmov
tv
MedAI #93: Toward Universal Medical Image Segmentation | Yunhe Gao
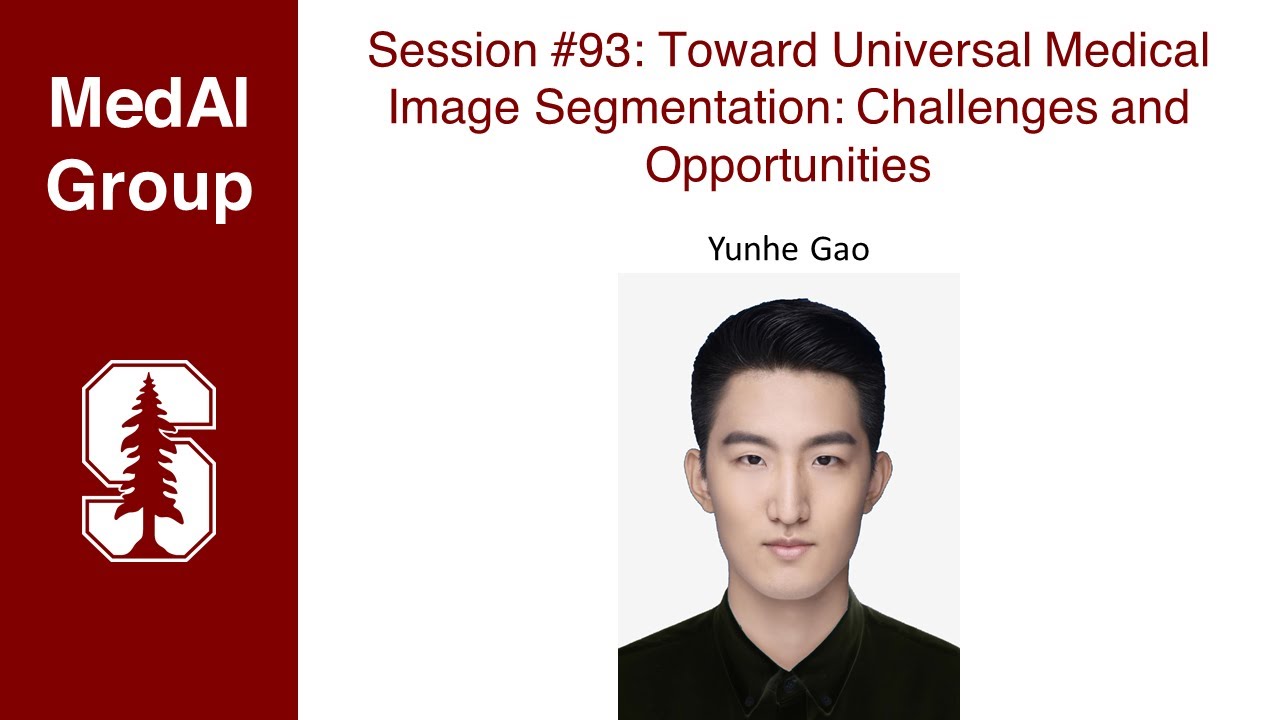
Показать описание
Title: Toward Universal Medical Image Segmentation: Challenges and Opportunities
Speaker: Yunhe Gao
Abstract:
A major enduring focus of clinical workflows is disease analytics and diagnosis, leading to medical imaging datasets where the modalities and annotations are strongly tied to specific clinical objectives. To date, the prevailing training paradigm for medical image segmentation revolves around developing separate models for specific medical objects (e.g., organs or tumors) and image modalities (e.g., CT or MR). This traditional paradigm can hinder the robustness and generalizability of these AI models, inflate costs when further scaling data volumes, and fail to exploit potential synergies among various medical imaging tasks. By observing the training program of radiology residency, we recognize that radiologists’ expertise arises from routine exposure to a diverse range of medical images across body regions, diseases, and imaging modalities. This observation motivates us to explore a new training paradigm, “universal medical image segmentation”, whose key goal is to learn from diverse medical imaging sources. In this talk, I’ll delve into challenges in the new paradigm including issues with partial labeling, conflicting class definitions, and significant data heterogeneity. I’ll also present our pioneering solution, Hermes, aimed at tackling these challenges. We demonstrate that our proposed universal paradigm not only offers enhanced performance and scalability, but also excels in transfer learning, incremental learning and generalization. This innovative approach opens up new perspectives for the construction of foundational models in a broad range of medical image analysis.
Speaker Bio:
Yunhe Gao is a fourth-year Ph.D. candidate in the Department of Computer Science at Rutgers University, advised by Distinguished Professor Dimitris Metaxas. His research focuses on computer vision and medical image analysis. He is also broadly interested in AI model robustness, data efficiency and their applications in the machine learning and healthcare domains. His research has been published in top-tier venues such as IEEE TMI, MedIA, ICCV, ECCV, IPMI and MICCAI.
------
The MedAI Group Exchange Sessions are a platform where we can critically examine key topics in AI and medicine, generate fresh ideas and discussion around their intersection and most importantly, learn from each other.
We will be having weekly sessions where invited speakers will give a talk presenting their work followed by an interactive discussion and Q&A. Our sessions are held every Thursday from 1pm-2pm PST.
Speaker: Yunhe Gao
Abstract:
A major enduring focus of clinical workflows is disease analytics and diagnosis, leading to medical imaging datasets where the modalities and annotations are strongly tied to specific clinical objectives. To date, the prevailing training paradigm for medical image segmentation revolves around developing separate models for specific medical objects (e.g., organs or tumors) and image modalities (e.g., CT or MR). This traditional paradigm can hinder the robustness and generalizability of these AI models, inflate costs when further scaling data volumes, and fail to exploit potential synergies among various medical imaging tasks. By observing the training program of radiology residency, we recognize that radiologists’ expertise arises from routine exposure to a diverse range of medical images across body regions, diseases, and imaging modalities. This observation motivates us to explore a new training paradigm, “universal medical image segmentation”, whose key goal is to learn from diverse medical imaging sources. In this talk, I’ll delve into challenges in the new paradigm including issues with partial labeling, conflicting class definitions, and significant data heterogeneity. I’ll also present our pioneering solution, Hermes, aimed at tackling these challenges. We demonstrate that our proposed universal paradigm not only offers enhanced performance and scalability, but also excels in transfer learning, incremental learning and generalization. This innovative approach opens up new perspectives for the construction of foundational models in a broad range of medical image analysis.
Speaker Bio:
Yunhe Gao is a fourth-year Ph.D. candidate in the Department of Computer Science at Rutgers University, advised by Distinguished Professor Dimitris Metaxas. His research focuses on computer vision and medical image analysis. He is also broadly interested in AI model robustness, data efficiency and their applications in the machine learning and healthcare domains. His research has been published in top-tier venues such as IEEE TMI, MedIA, ICCV, ECCV, IPMI and MICCAI.
------
The MedAI Group Exchange Sessions are a platform where we can critically examine key topics in AI and medicine, generate fresh ideas and discussion around their intersection and most importantly, learn from each other.
We will be having weekly sessions where invited speakers will give a talk presenting their work followed by an interactive discussion and Q&A. Our sessions are held every Thursday from 1pm-2pm PST.