filmov
tv
Yu Cheng: Towards data efficient vision-language (VL) models
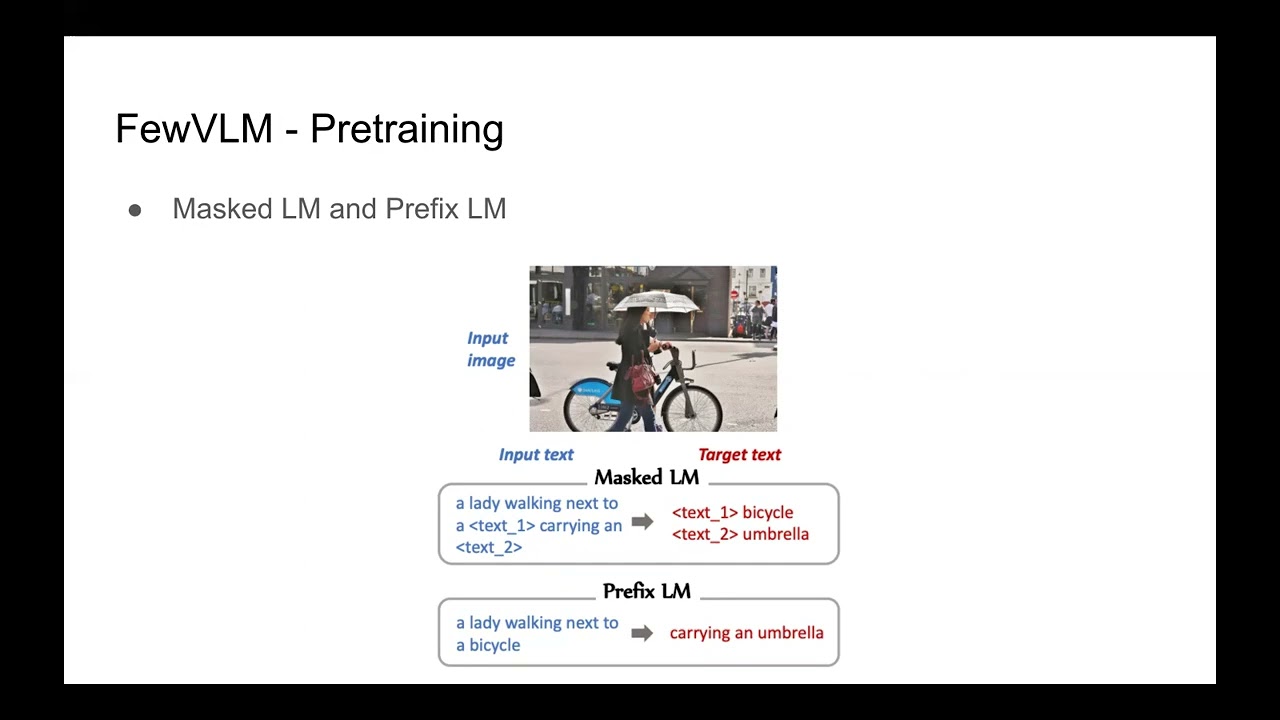
Показать описание
Abstract: Language transformers have shown remarkable performance on natural language understanding tasks. However, these gigantic VL models are hard to deploy for real-world applications due to their impractically huge model size and the requirement for downstream fine-tuning data. In this talk, I will first present FewVLM, a few-shot prompt-based learner on vision-language tasks. FewVLM is trained with both prefix language modeling and masked language modeling and utilizes simple prompts to improve zero/few-shot performance on VQA and image captioning. Then I will introduce Grounded-FewVLM, a new version that learns object grounding and localization in pre-training and can adapt to diverse grounding tasks. The models have been evaluated on various zero-/few-shot VL tasks and the results show that they consistently surpass the state-of-the-art few-shot methods.
Bio: Yu Cheng is a Principal Researcher at Microsoft Research. Before joining Microsoft, he was a Research Staff Member at IBM Research & MIT-IBM Watson AI Lab. He got a Ph.D. degree from Northwestern University in 2015 and a bachelor’s degree from Tsinghua University in 2010. His research covers deep learning in general, with specific interests in model compression and efficiency, deep generative models, and adversarial robustness. Currently, he focuses on productionizing these techniques to solve challenging problems in CV, NLP, and Multimodal. Yu is serving (or, has served) as an area chair for CVPR, NeurIPS, AAAI, IJCAI, ACMMM, WACV, and ECCV.
Bio: Yu Cheng is a Principal Researcher at Microsoft Research. Before joining Microsoft, he was a Research Staff Member at IBM Research & MIT-IBM Watson AI Lab. He got a Ph.D. degree from Northwestern University in 2015 and a bachelor’s degree from Tsinghua University in 2010. His research covers deep learning in general, with specific interests in model compression and efficiency, deep generative models, and adversarial robustness. Currently, he focuses on productionizing these techniques to solve challenging problems in CV, NLP, and Multimodal. Yu is serving (or, has served) as an area chair for CVPR, NeurIPS, AAAI, IJCAI, ACMMM, WACV, and ECCV.