filmov
tv
Maximum Likelihood - Cramer Rao Lower Bound Intuition
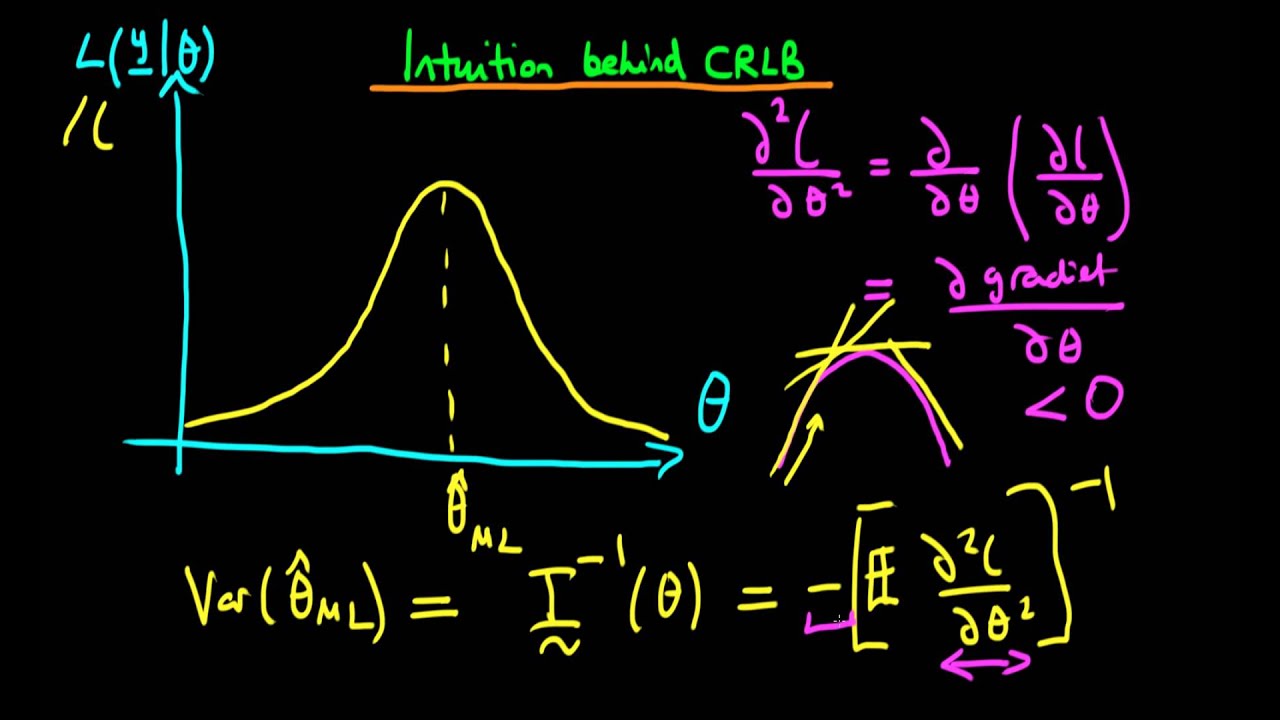
Показать описание
This video provides some intuition behind the idea that the Cramer-Rao Lower Bound is inversely related to the variance of a maximum likelihood estimator.
Maximum Likelihood - Cramer Rao Lower Bound Intuition
The Cramer-Rao Lower Bound: Inference in Maximum Likelihood Estimation
The Cramer-Rao Lower Bound ... MADE EASY!!!
Introduction to Cramer-Rao Lower Bound
The Fisher Information
cramer rao
MATH 341 Parameter Estimation (CI for MLE, efficiency, Cramer-Rao lower bound, and sufficiency)
6. Maximum Likelihood Estimation (cont.) and the Method of Moments
The Cramer-Rao Inequality
Quantum parameter estimation, Fisher information, and the Cramér-Rao bound
Fisher information explained in 5 minutes
Pillai: Cramer-Rao Bound (Version-2)
STATS 100B - Intro to Math Stat - Lecture 13 (Fisher information; Cramer-Rao inequality; Efficiency)
Visualizing the Cramer-Rao Lower Bound for MLEs of normal distributions
Maximum likelihood estimation for the beer example model
Find the Cramer Rao Lower Bound of the Bernoulli Distribution
Pillai: Examples on Cramer-Rao Bound
Cramer Rao Inequality
Pillai, Lect.8, April '14 'The Cramer Rao Bound for the Multi-Parameter case'
452 S21 Lecture 10 - Cramer-Rao Lower Bound
What is Fisher Information?
Maximum Likelihood estimation - an introduction part 1
Cramer-Rao lower bound demonstrated experimentally for the family of normal distributions
Unit 9 - Part 1 - Rao Cramer Lower Bound
Комментарии