filmov
tv
Magdalena Rybicka: End-to-End Neural Speaker Diarization with Non-Autoregressive Attractors
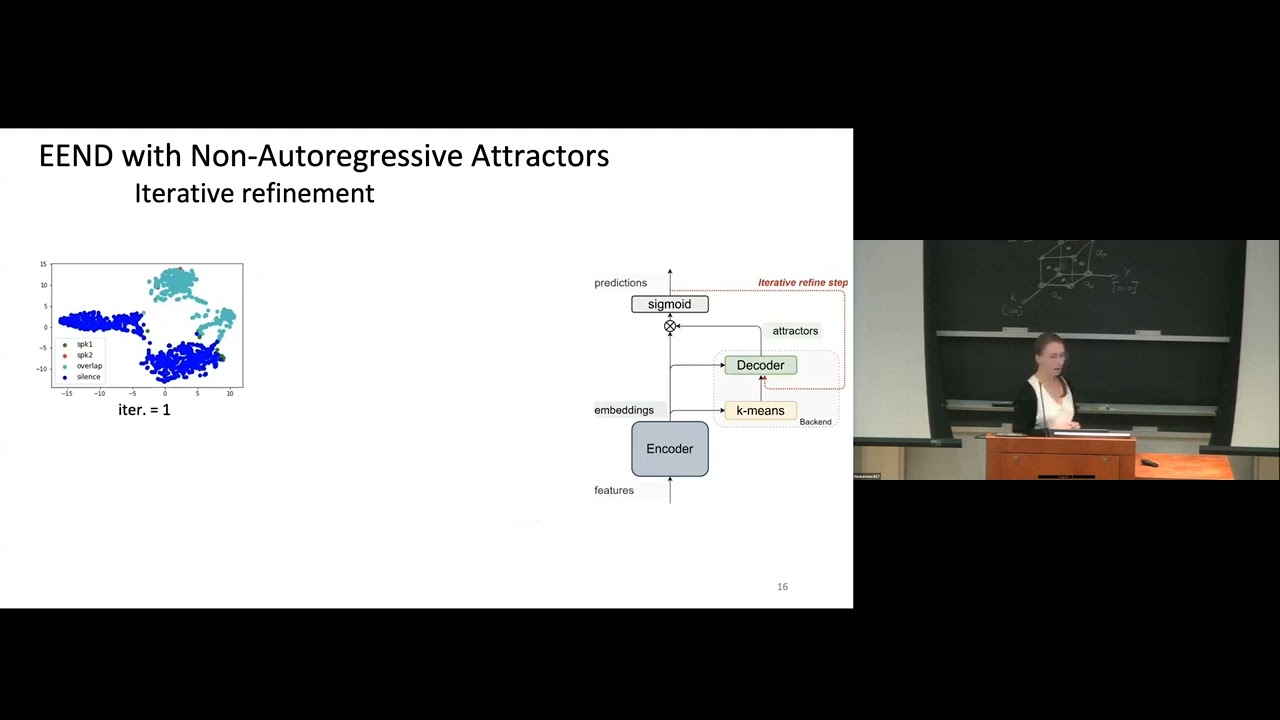
Показать описание
Abstract
Despite many recent developments in speaker diarization, it remains a challenge and an active area of research to make diarization robust and effective in real-life scenarios. End-to-end neural speaker diarization (EEND) systems are considered the next stepping stone in pursuing high-performance diarization. Next, the appearance of EEND with encoder-decoder-based attractors (EEND-EDA) enabled us to deal with recordings that contain a flexible number of speakers thanks to an LSTM-based EDA module. In this talk, I will describe our work on EEND with Non-Autoregressive Attractors (EEND-NAA) approach and recent further improvements to the EEND-NAA architecture, which can handle recordings containing speech of a variable and unknown number of speakers. Our proposed system uses a clustering approach but follows the EEND-EDA framework and end-to-end pipeline, where the autoregressive LSTM-based backend is replaced with non-autoregressive attractor estimation. Our proposal allows to make the process of attractor generation explainable, while the LSTM-based is more obscure.
Speaker bio:
Magdalena Rybicka received the B.Eng. and M.Sc. degrees in Electronics and Telecommunications from AGH University of Science and Technology in Krakow in 2018 and 2019. Her Master thesis focused on speaker recognition in adverse acoustic conditions. Currently, she is a PhD candidate advised by Konrad Kowalczyk. Her research interests are related to application of machine learning in speaker recognition task.
Despite many recent developments in speaker diarization, it remains a challenge and an active area of research to make diarization robust and effective in real-life scenarios. End-to-end neural speaker diarization (EEND) systems are considered the next stepping stone in pursuing high-performance diarization. Next, the appearance of EEND with encoder-decoder-based attractors (EEND-EDA) enabled us to deal with recordings that contain a flexible number of speakers thanks to an LSTM-based EDA module. In this talk, I will describe our work on EEND with Non-Autoregressive Attractors (EEND-NAA) approach and recent further improvements to the EEND-NAA architecture, which can handle recordings containing speech of a variable and unknown number of speakers. Our proposed system uses a clustering approach but follows the EEND-EDA framework and end-to-end pipeline, where the autoregressive LSTM-based backend is replaced with non-autoregressive attractor estimation. Our proposal allows to make the process of attractor generation explainable, while the LSTM-based is more obscure.
Speaker bio:
Magdalena Rybicka received the B.Eng. and M.Sc. degrees in Electronics and Telecommunications from AGH University of Science and Technology in Krakow in 2018 and 2019. Her Master thesis focused on speaker recognition in adverse acoustic conditions. Currently, she is a PhD candidate advised by Konrad Kowalczyk. Her research interests are related to application of machine learning in speaker recognition task.