filmov
tv
Chris Manning - Meaning and Intelligence in Language Models (COLM 2024)
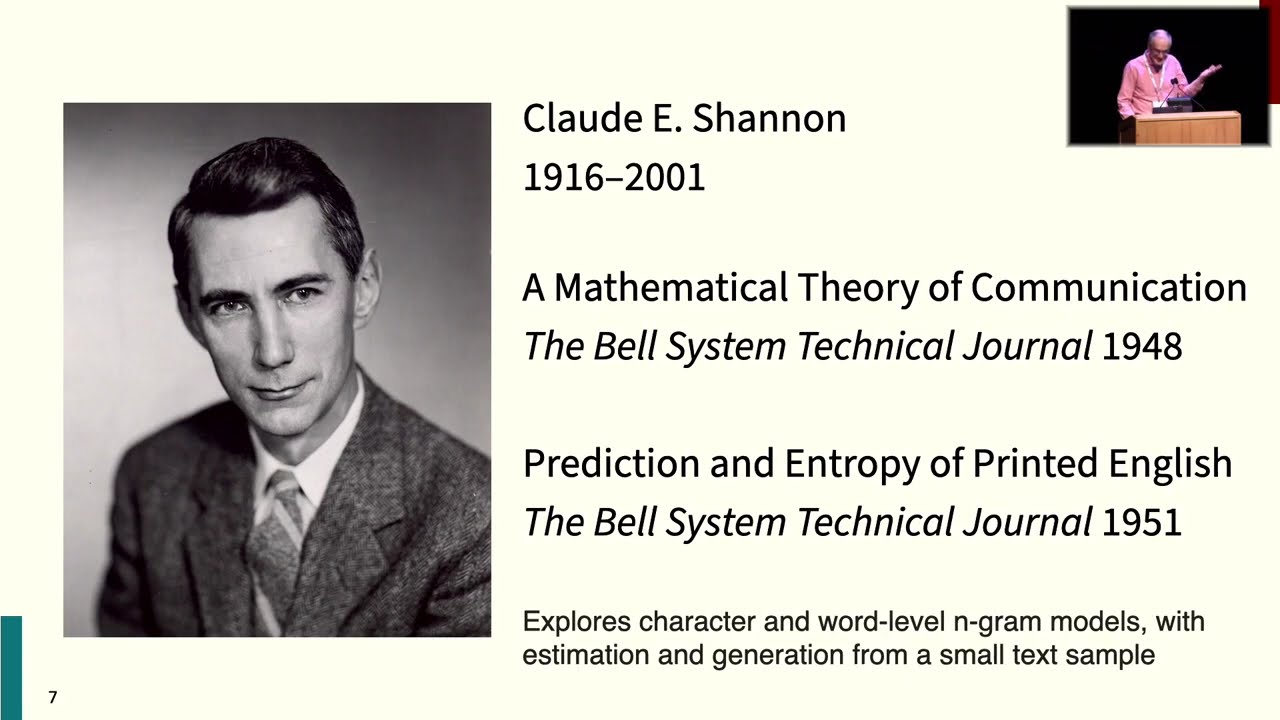
Показать описание
Meaning and Intelligence in Language Models: From Philosophy to Agents in a World
Language Models have been around for decades but have suddenly taken the world by storm. In a surprising third act for anyone doing NLP in the 70s, 80s, 90s, or 2000s, in much of the popular media, artificial intelligence is now synonymous with language models. In this talk, I want to take a look backward at where language models came from and why they were so slow to emerge, a look inward to give my thoughts on meaning, intelligence, and what language models understand and know, and a look forward at what we need to build intelligent language-using agents in a world. I will argue that material beyond language is not necessary to having meaning and understanding, but it is very useful in most cases, and that adaptability and learning are vital to intelligence, and so the current strategy of building from huge curated data will not truly get us there, even though LLMs have so many good uses. For a web agent, I look at how it can learn through interactions and make good use of the hierarchical structure of language to make exploration tractable. I will show recent work with Shikhar Murty about how an interaction-first learning approach for web agents can work very effectively, giving gains of 20 percent on MiniWoB++ over either a zero-shot language model agent or an instruction-first fine-tuned agent.
Language Models have been around for decades but have suddenly taken the world by storm. In a surprising third act for anyone doing NLP in the 70s, 80s, 90s, or 2000s, in much of the popular media, artificial intelligence is now synonymous with language models. In this talk, I want to take a look backward at where language models came from and why they were so slow to emerge, a look inward to give my thoughts on meaning, intelligence, and what language models understand and know, and a look forward at what we need to build intelligent language-using agents in a world. I will argue that material beyond language is not necessary to having meaning and understanding, but it is very useful in most cases, and that adaptability and learning are vital to intelligence, and so the current strategy of building from huge curated data will not truly get us there, even though LLMs have so many good uses. For a web agent, I look at how it can learn through interactions and make good use of the hierarchical structure of language to make exploration tractable. I will show recent work with Shikhar Murty about how an interaction-first learning approach for web agents can work very effectively, giving gains of 20 percent on MiniWoB++ over either a zero-shot language model agent or an instruction-first fine-tuned agent.
Комментарии