filmov
tv
AGU 2020: Evolutionary full-waveform inversion of the African Plate
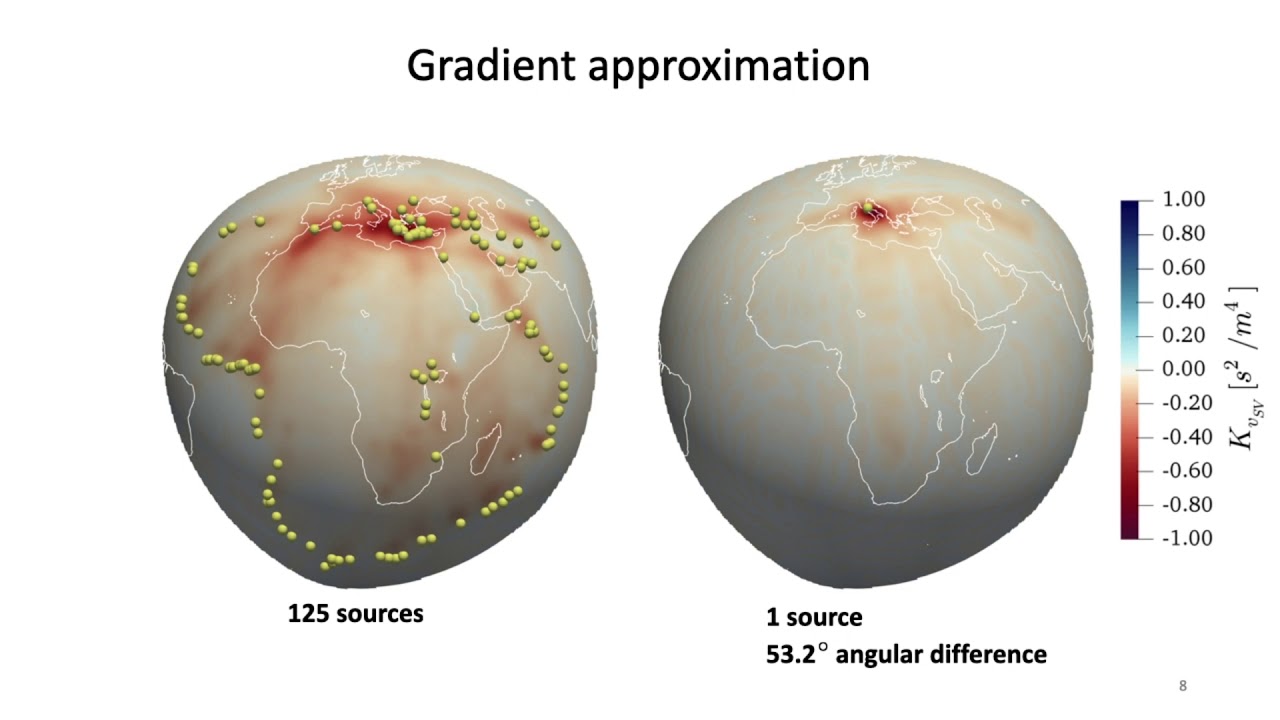
Показать описание
We present a new approach for the assimilation of new data in full-waveform inversion models. This evolutionary inversion is built on a form of stochastic L-BFGS that we refer to as dynamic mini-batch optimization. The motivation for this work is driven by the observation that seismic data volumes continue to grow roughly exponentially. In theory, all this new data should enable us to improve our models. In practice, however, most Earth models are currently built from a static dataset.
In contrast to conventional waveform inversion, our approach uses dynamically changing mini-batches (subsets of data) that approximate the gradient of the larger dataset at each iteration. This has three major advantages, (1) We exploit redundancies within the dataset, which results in a reduced computational cost for model updates, (2) The size of the complete dataset does not directly impact the computational cost of an iteration, thereby enabling us to work with larger datasets, and (3) The nature of the algorithm makes it trivial to assimilate new data, as the new data can simply be added to the complete dataset from which the mini-batches are sampled.
To show the utility of this approach, we apply it to invert for upper-mantle structure beneath the African Plate. Starting from a 1-D model and with data recorded until 1995, we sequentially grow the dataset by incorporating more and more recent data into this ongoing inversion. We then show how a consistent measure of misfit can be maintained and the model evolves over time as a function of data coverage.
In contrast to conventional waveform inversion, our approach uses dynamically changing mini-batches (subsets of data) that approximate the gradient of the larger dataset at each iteration. This has three major advantages, (1) We exploit redundancies within the dataset, which results in a reduced computational cost for model updates, (2) The size of the complete dataset does not directly impact the computational cost of an iteration, thereby enabling us to work with larger datasets, and (3) The nature of the algorithm makes it trivial to assimilate new data, as the new data can simply be added to the complete dataset from which the mini-batches are sampled.
To show the utility of this approach, we apply it to invert for upper-mantle structure beneath the African Plate. Starting from a 1-D model and with data recorded until 1995, we sequentially grow the dataset by incorporating more and more recent data into this ongoing inversion. We then show how a consistent measure of misfit can be maintained and the model evolves over time as a function of data coverage.