filmov
tv
MDS20 Minitutorial: ODE/PDE Neural Networks by Eldad Haber
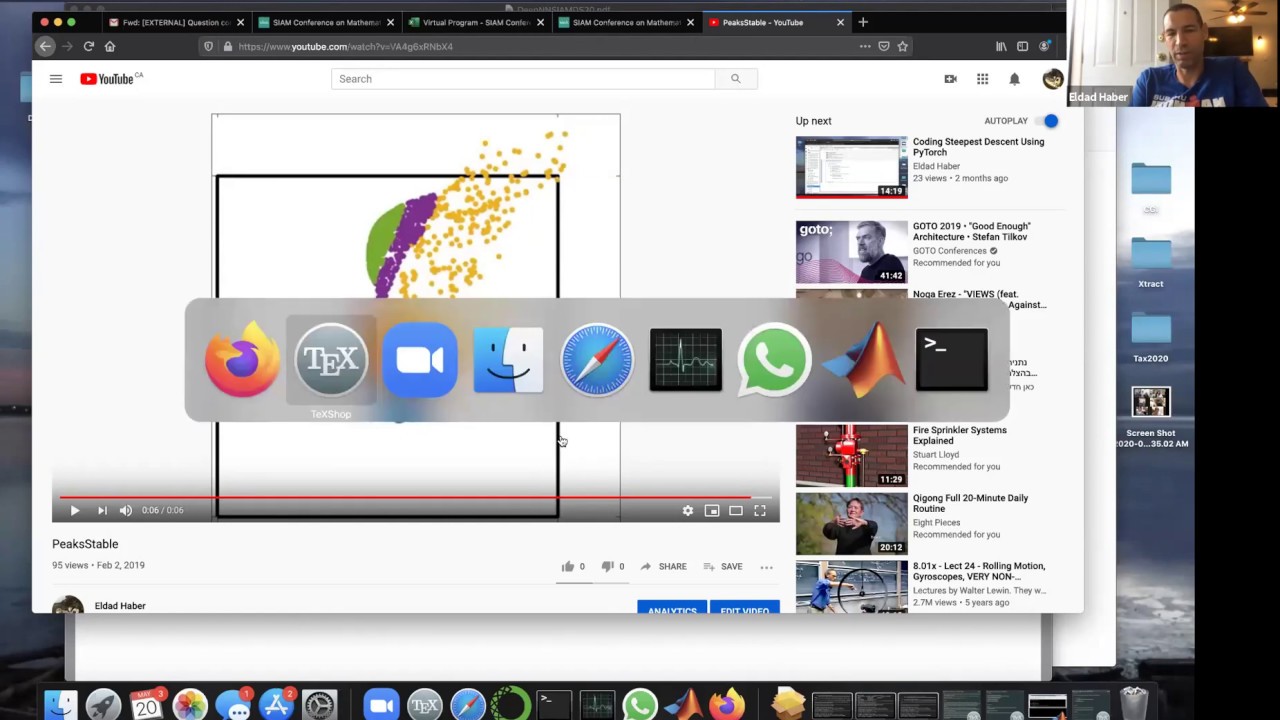
Показать описание
Abstract: Deep learning plays an increasing role in mathematical areas, like inverse problems and partial differential equations (PDEs). A factor behind this development is the idea of complementing physics-based models with data-driven components, the latter to introduce an adaptability to the data and make up for necessary modelling inaccuracies. Another benefit of deep learning is that once trained they are computationally fast to apply. Hence, this offers new ways to tackle computationally challenging problems, like the numerical solution of high-dimensional and non-linear PDEs. The potential for deep learning in enhancing these mathematical models through the aforementioned development has this far been supported by empirical evidence. Very little has been done in the context of mathematical theory that can support this empirical evidence. This tutorial brings to bare some of these rare and recent mathematical contributions for bringing numerical experiments closer to rigorous mathematical reasoning. The tutorial should serve as an inspiration for mathematicians to enter this field and provide their so needed contribution. To do so we propose a 2-part structure, with the first part being dedicated to deep learning for inverse problems, and the second to deep learning for PDEs.
Комментарии