filmov
tv
Quantum algorithms for approximate function loading [QCT21/22, Seminar #06]
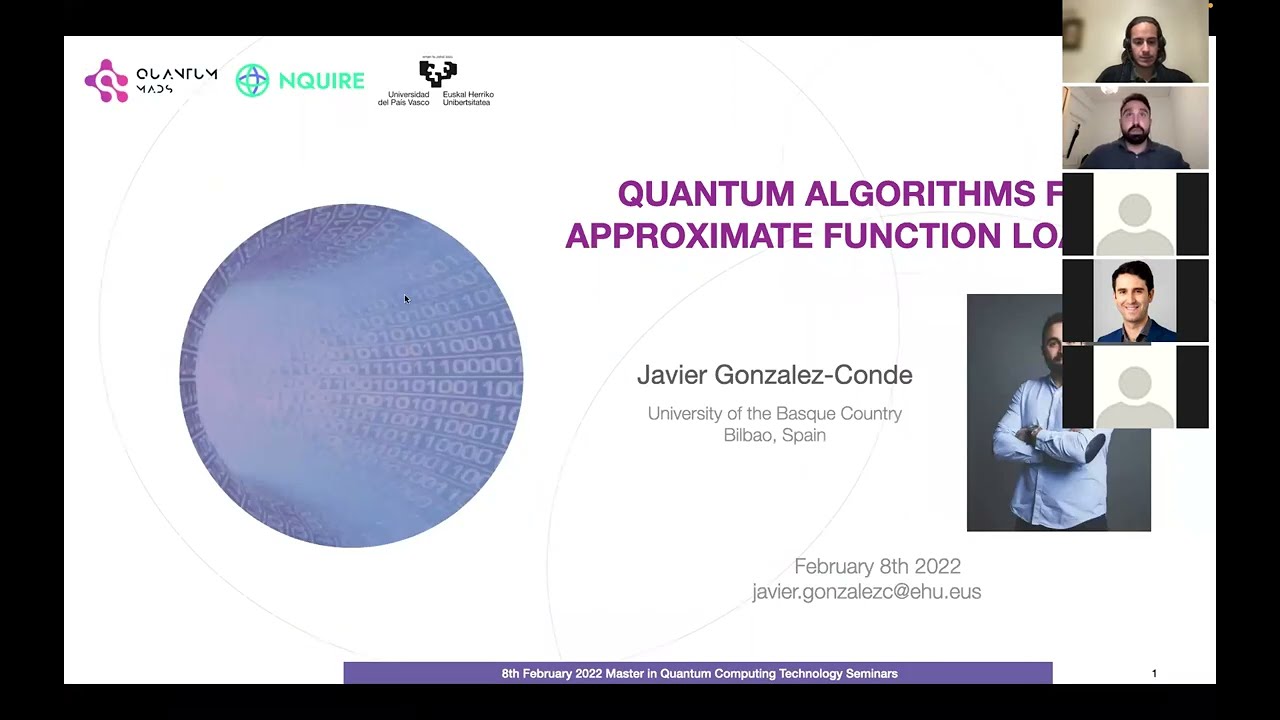
Показать описание
By Javier González Conde (Quantum Mads).
Loading classical data into quantum computers represents an essential stage in many relevant quantum algorithms, especially in the field of quantum machine learning. Therefore, the inefficiency of this loading process means a major bottleneck for the application of these algorithms. In this seminar, we introduce two approximate quantum-state preparation methods inspired by the Grover-Rudolph algorithm, which partially solve the problem of loading real functions. Indeed, by allowing for an infidelity ϵ and under certain smoothness conditions, we prove that the complexity of Grover-Rudolph algorithm can be reduced from O(2^n) to O(2^(k0(ϵ))), with n the number of qubits and k0(ϵ) asymptotically independent of n. This leads to a dramatic reduction in the number of required two-qubit gates. Aroused by this result, we also propose a variational algorithm capable of loading functions beyond the aforementioned smoothness conditions. Our variational ansatz is explicitly tailored to the landscape of the function, leading to a quasi-optimized number of hyperparameters. This allows us to achieve high fidelity in the loaded state with high speed convergence for the studied examples.
After acquiring a formation in Mathematics, Physics and the recently emerged techniques of Machine Learning and Big Data, Javier took the decision of orientating his professional career towards the quantum world. He is currently a junior researcher and PhD student at the University of the Basque Country, Bilbao, belonging to the group Quantum Computing and Architectures leaded by Dr. Mikel Sanz. He is also CEO of Quantum Mads, one of the top quantum computing Spanish startups. He has focused his doctoral thesis on studying a wide range of Differential Equations based on financial models (pricing, risk), fluids, etc. solved by applying different techniques based on quantum computation, such as quantum simulations and HHL algorithm applied to numerical methods.
Loading classical data into quantum computers represents an essential stage in many relevant quantum algorithms, especially in the field of quantum machine learning. Therefore, the inefficiency of this loading process means a major bottleneck for the application of these algorithms. In this seminar, we introduce two approximate quantum-state preparation methods inspired by the Grover-Rudolph algorithm, which partially solve the problem of loading real functions. Indeed, by allowing for an infidelity ϵ and under certain smoothness conditions, we prove that the complexity of Grover-Rudolph algorithm can be reduced from O(2^n) to O(2^(k0(ϵ))), with n the number of qubits and k0(ϵ) asymptotically independent of n. This leads to a dramatic reduction in the number of required two-qubit gates. Aroused by this result, we also propose a variational algorithm capable of loading functions beyond the aforementioned smoothness conditions. Our variational ansatz is explicitly tailored to the landscape of the function, leading to a quasi-optimized number of hyperparameters. This allows us to achieve high fidelity in the loaded state with high speed convergence for the studied examples.
After acquiring a formation in Mathematics, Physics and the recently emerged techniques of Machine Learning and Big Data, Javier took the decision of orientating his professional career towards the quantum world. He is currently a junior researcher and PhD student at the University of the Basque Country, Bilbao, belonging to the group Quantum Computing and Architectures leaded by Dr. Mikel Sanz. He is also CEO of Quantum Mads, one of the top quantum computing Spanish startups. He has focused his doctoral thesis on studying a wide range of Differential Equations based on financial models (pricing, risk), fluids, etc. solved by applying different techniques based on quantum computation, such as quantum simulations and HHL algorithm applied to numerical methods.