filmov
tv
Partial Dependence (PDPs) and Individual Conditional Expectation (ICE) Plots | Intuition and Math
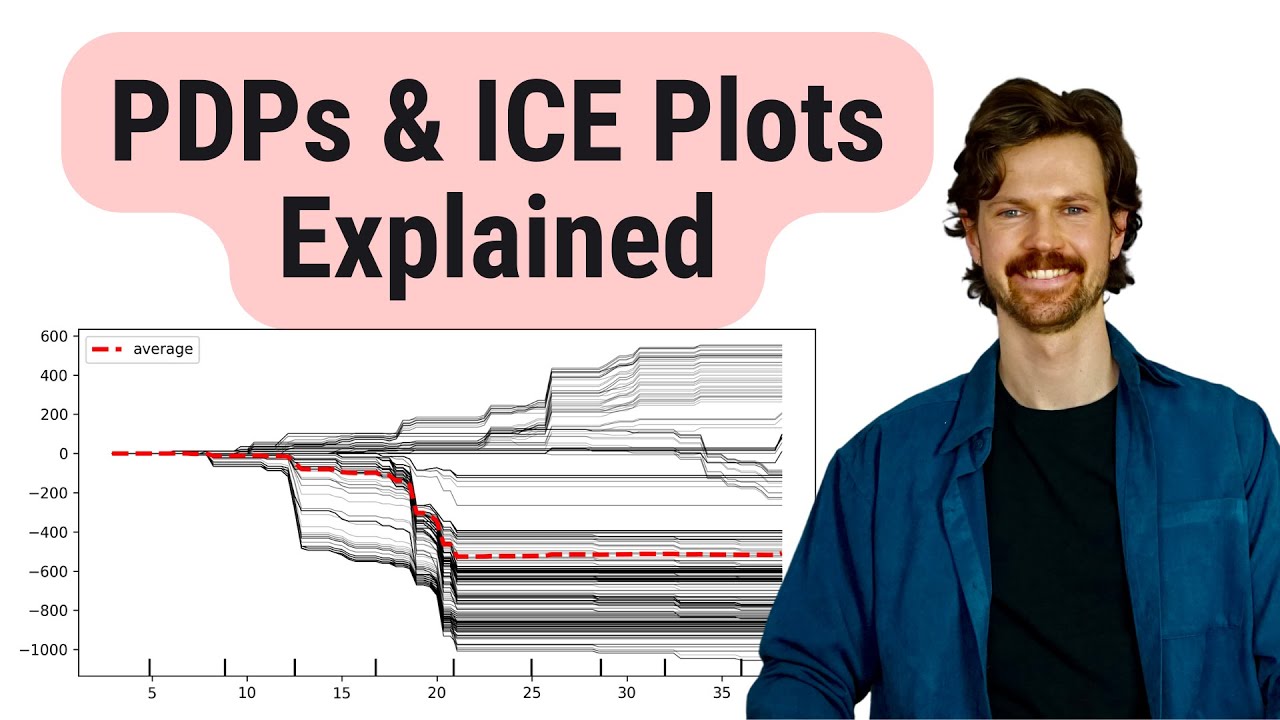
Показать описание
Both Partial Dependence (PDPs) and Individual Conditional Expectation (ICE) Plots are used to understand and explain machine learning models. PDPs can tell us if a relationship between a model feature and target variable is linear, non-linear or if there is no relationship. Similarly, ICE plots are used to visualise interactions. Now, at first glance, these plots may look complicated. But you will see, they are actually constructed in a fairly intuitive way.
In this video, we will:
- Take you step-by-step through how PDPs and ICE plots are created.
- Discuss what insight the explainable AI methods can give
- And we will end by explaining the mathematics behind PDPs.
🚀 Free Course 🚀
🚀 Companion article with link to code (no-paywall link): 🚀
🚀 Useful playlists 🚀
🚀 Get in touch 🚀
🚀 Chapters 🚀
00:00 Introduction
01:31 Understanding PDPs
04:20 Visualising relationships with PDPs
06:58 Understanding ICE Plots
06:26 The maths
In this video, we will:
- Take you step-by-step through how PDPs and ICE plots are created.
- Discuss what insight the explainable AI methods can give
- And we will end by explaining the mathematics behind PDPs.
🚀 Free Course 🚀
🚀 Companion article with link to code (no-paywall link): 🚀
🚀 Useful playlists 🚀
🚀 Get in touch 🚀
🚀 Chapters 🚀
00:00 Introduction
01:31 Understanding PDPs
04:20 Visualising relationships with PDPs
06:58 Understanding ICE Plots
06:26 The maths
Partial Dependence (PDPs) and Individual Conditional Expectation (ICE) Plots | Intuition and Math
Model Auditing: Partial Dependence Plot (PDP) and Individual Conditional Expectation (ICE)
Partial Dependence Plots (Opening the Black Box)
Partial Dependence Plot (PDP) in Python
(HSMA 6 Day 13) 4G Part 4/6 - Partial Dependence Plots and Individual Conditional Expectation Plots
Interpretable Machine Learning - Feature Effects - Individual Conditional Expectation (ICE) Plots
Use Classification Learner App to Interpret Machine Learning Models with Partial Dependence Plots
How to Build Shap Single Feature Partial Dependence Plot (PDP Plot)
Explain Machine-learning Models: Individual Conditional Expectation (ICE) in Python
Partial dependency plot: How feature impact model prediction?
How to Build 3D Shap Two Feature Partial Dependence Plot (PDP Plot)
SHAP values for beginners | What they mean and their applications
#128: Scikit-learn 122: Inspection
Partial dependence plots for Mario Kart world records
23. Partial Dependence Plot / DAI Starter Course
Explaining Hyperparameter Optimization via Partial Dependence Plots (NeurIPS'21)
How to Build Shap Two Feature Partial Dependance Plot (PDP Plot)
R : Partial dependence plot from an xgboost model in R
Explanatory Model Analysis: Partial-dependence Profiles (ema01 17)
PDPs and ICE Plots | Python Code | scikit-learn Package
Ensembles
Shapley Values : Data Science Concepts
Model Interpretability in MATLAB
SHAP with Python (Code and Explanations)
Комментарии