filmov
tv
Matthew Tancik: Neural Radiance Fields for View Synthesis
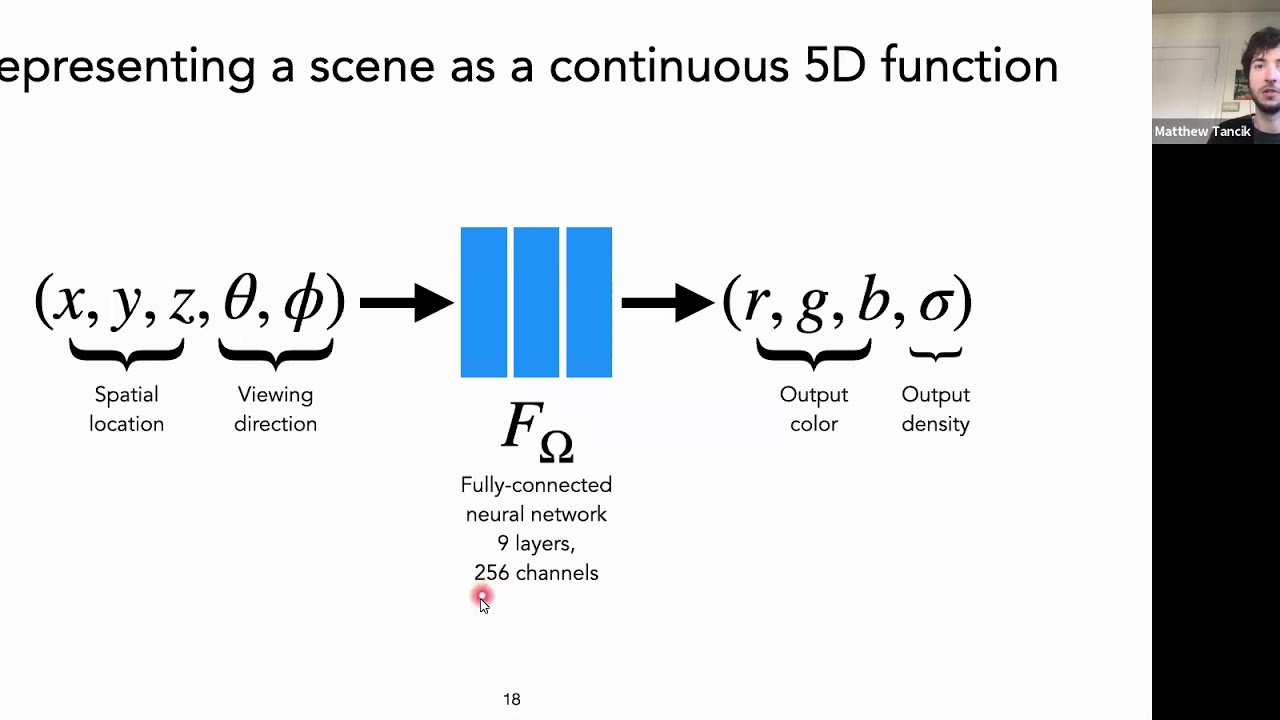
Показать описание
Talk @ Tübingen seminar series of the Autonomous Vision Group
Neural Radiance Fields for View Synthesis
Matthew Tancik (UC Berkeley)
Abstract: In this talk I will present our recent work on Neural Radiance Fields (NeRFs) for view synthesis. We are able to achieve state-of-the-art results for synthesizing novel views of scenes with complex geometry and view dependent effects from a sparse set of input views by optimizing an underlying continuous volumetric scene function parameterized as a fully-connected deep network. In this work we combine the recent advances in coordinate based neural representations with classic methods for volumetric rendering. In order to recover high frequency content in the scene, we find that it is necessary to map the input coordinates to a higher dimensional space using Fourier features before feeding them through the network. In our followup work we use Neural Tangent Kernel analysis to show that this is equivalent to transforming our network into a stationary kernel with tunable bandwidth.
Neural Radiance Fields for View Synthesis
Matthew Tancik (UC Berkeley)
Abstract: In this talk I will present our recent work on Neural Radiance Fields (NeRFs) for view synthesis. We are able to achieve state-of-the-art results for synthesizing novel views of scenes with complex geometry and view dependent effects from a sparse set of input views by optimizing an underlying continuous volumetric scene function parameterized as a fully-connected deep network. In this work we combine the recent advances in coordinate based neural representations with classic methods for volumetric rendering. In order to recover high frequency content in the scene, we find that it is necessary to map the input coordinates to a higher dimensional space using Fourier features before feeding them through the network. In our followup work we use Neural Tangent Kernel analysis to show that this is equivalent to transforming our network into a stationary kernel with tunable bandwidth.
Matthew Tancik: Neural Radiance Fields for View Synthesis
NeRF: Neural Radiance Fields
NeRF: Representing Scenes as Neural Radiance Fields for View Synthesis (ML Research Paper Explained)
Plenoxels vs. NeRF - Optimization Speed Comparison
Learned Initializations for Optimizing Coordinate-Based Neural Representations
The Unreasonable Power of Neural Radiance Fields (NeRFs)
Jon Barron - Understanding and Extending Neural Radiance Fields
Summary of the Plenoxels Approach
Plenoxels - Forward-facing Real Scenes
Neural Radiance Fields, Luma Ai.
Fourier Features Let Networks Learn High Frequency Functions in Low Dimensional Domains
[ECCV 2020] NeRF: Neural Radiance Fields (10 min talk)
KiloNeRF: Speeding up Neural Radiance Fields with Thousands of Tiny MLPs
Interacting with Neural Radiance Fields in Immersive Virtual Reality
MAS.S61 presents Matthew Tancik, Co-Author of NeRF and NeRF Studio
An Overview of Neural Radiance Fields [+Discussion]
Neural Radiance Field - filipino bangka
Plenoxels vs. NeRF - Dinosaur
Test using neural rendering (NERF) on outdoor scene, no drone involved
Plenoxels: Radiance Fields without Neural Networks
Baking Neural Radiance Fields for Real-Time View Synthesis
Plenoxels vs. NeRF - Synthetic Ship
Unlocking NeRF: Synthesizing Photorealistic 3D Scenes Simplified!
Hallucinated Neural Radiance Fields in the Wild
Комментарии