filmov
tv
Summary: Bayesian vs Frequentist statistics
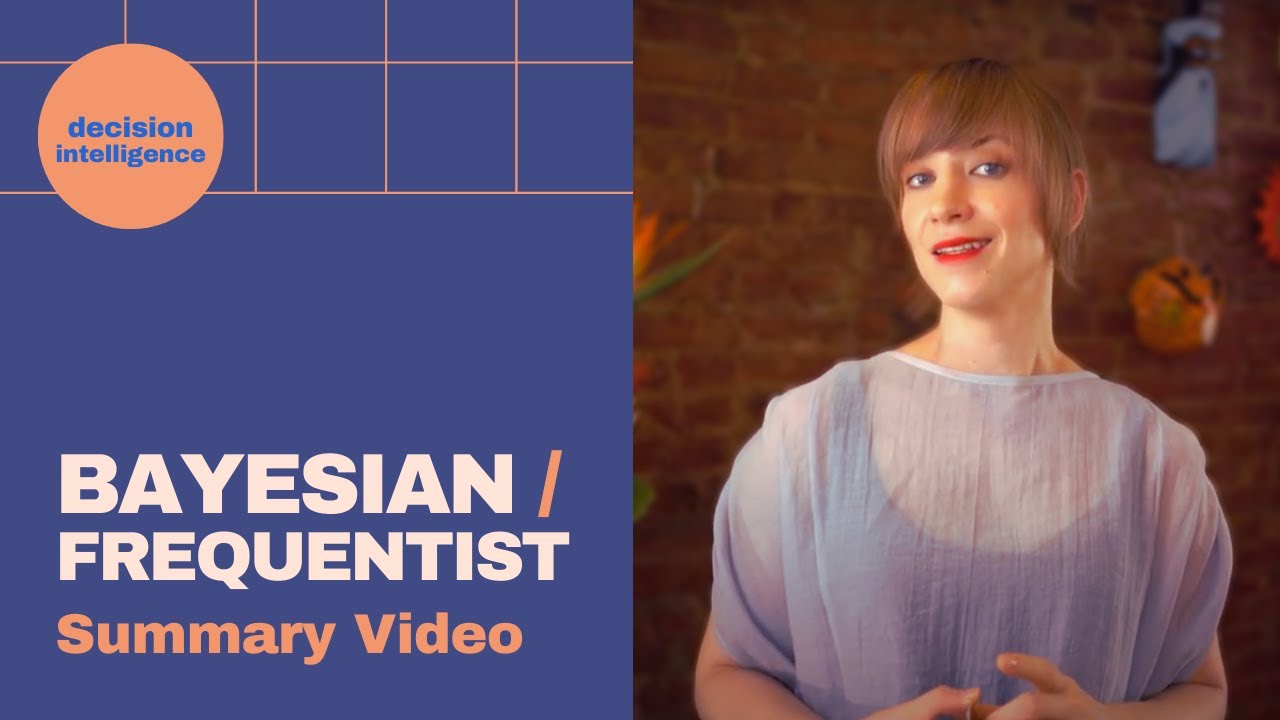
Показать описание
Statistics is the science of changing your mind. There are two equally reasonable schools of thought. The more popular one - Frequentist statistics - is all about checking whether you should leave your default action. Bayesian statistics is all about having a prior opinion and updating that opinion with data.
[Read the summary below after watching both videos.]
SUMMARY OF BOTH VIDEOS
It is only by insisting that the parameter may not be a random variable (Frequentist) that it makes any kind of sense to talk about your method's ability to deliver the right answer. As soon as you let the parameter be a random variable (Bayesian), there's no longer any notion of right and wrong.
BAYES VS FREQUENTIST... FIGHT!
What words tell you that we're wading through their territory?
Frequentist: confidence interval, p-value, power, significance
Bayesian: credible interval, prior, posterior
What are they using statistics to change their minds about?
Bayesian: opinions to have (prior belief)
What is the main difference?
Frequentist: the parameter is a fixed quantity (no probability about it) -- "The coin has landed. P(Heads) is 0% or 100%, I just don't know which one."
Bayesian: the parameter is random variable (no right answer) -- "For me, it's 50%, for you it's whatever it is for you."
What do you win if you choose this side?
Frequentist: it makes sense to talk about your method's quality and "getting the answer right"
Bayesian: intuitive definitions, e.g. credible intervals are what you wish confidence intervals were (but aren't!)
What do you lose if you choose this side?
Frequentist: the core objects make no sense to beginners (e.g. p-values and confidence intervals are hard to think about) and lazy thinkers make a hash out them frequent-ly.
Bayesian: you lose the ability to talk about any notion of "right answers" and "method quality" - there's no such thing as statistically significant or rejecting the null. There's only "more likely" and "less likely" ...from your perspective.
So, which one is better?
Wrong question! The right one to choose depends on how you want to approach your decision-making. For example, if you have no default action, the Frequentist approach is less practical than the Bayesian approach, unless you have other philosophical reasons for invoking the concept of TRUTH in your calculations.
Okay... so which one is more objective and "correct"?
The key difference is how they assist decision-making once the decision context has been framed.
Cassie, you're killing us here. Are you Bayesian or Frequentist?
Both! I choose based on how I'm framing my decision-making. It depends on whether the situation calls for choosing between actions or forming an evidence-based opinion.
So, does that mean I don't have to pick a side?
I'd advise against it (unless you've spent a few years thinking about the philosophy of statistics and you're willing to die on this hill). Honestly, it's a little silly to declare yourself as one of the other unless you've pondered them very deeply. Having had the pleasure of doing my graduate work at Duke University (which is to Bayesian statistics approximately what the Vatican is to Catholicism), I noticed that the loudest loudmouths about the superiority of Bayesian statistics aren't the profs... it's the newbie students who are relieved not to have to memorize the definition of the weird Frequentist confidence interval anymore (the Bayesian credible interval is so much easier). The profs understand that "better" depends on why you're trying to do. They spend a lot of time thinking in the Bayesian way because it fits the kind of decision approaches they're interested in.
So, my advice? Don't pick a side. See them as two different approaches that fit two different styles of decision-making and reasoning, then leave yourself the option of using whichever one suits the mindset/context you find yourself in.
[Read the summary below after watching both videos.]
SUMMARY OF BOTH VIDEOS
It is only by insisting that the parameter may not be a random variable (Frequentist) that it makes any kind of sense to talk about your method's ability to deliver the right answer. As soon as you let the parameter be a random variable (Bayesian), there's no longer any notion of right and wrong.
BAYES VS FREQUENTIST... FIGHT!
What words tell you that we're wading through their territory?
Frequentist: confidence interval, p-value, power, significance
Bayesian: credible interval, prior, posterior
What are they using statistics to change their minds about?
Bayesian: opinions to have (prior belief)
What is the main difference?
Frequentist: the parameter is a fixed quantity (no probability about it) -- "The coin has landed. P(Heads) is 0% or 100%, I just don't know which one."
Bayesian: the parameter is random variable (no right answer) -- "For me, it's 50%, for you it's whatever it is for you."
What do you win if you choose this side?
Frequentist: it makes sense to talk about your method's quality and "getting the answer right"
Bayesian: intuitive definitions, e.g. credible intervals are what you wish confidence intervals were (but aren't!)
What do you lose if you choose this side?
Frequentist: the core objects make no sense to beginners (e.g. p-values and confidence intervals are hard to think about) and lazy thinkers make a hash out them frequent-ly.
Bayesian: you lose the ability to talk about any notion of "right answers" and "method quality" - there's no such thing as statistically significant or rejecting the null. There's only "more likely" and "less likely" ...from your perspective.
So, which one is better?
Wrong question! The right one to choose depends on how you want to approach your decision-making. For example, if you have no default action, the Frequentist approach is less practical than the Bayesian approach, unless you have other philosophical reasons for invoking the concept of TRUTH in your calculations.
Okay... so which one is more objective and "correct"?
The key difference is how they assist decision-making once the decision context has been framed.
Cassie, you're killing us here. Are you Bayesian or Frequentist?
Both! I choose based on how I'm framing my decision-making. It depends on whether the situation calls for choosing between actions or forming an evidence-based opinion.
So, does that mean I don't have to pick a side?
I'd advise against it (unless you've spent a few years thinking about the philosophy of statistics and you're willing to die on this hill). Honestly, it's a little silly to declare yourself as one of the other unless you've pondered them very deeply. Having had the pleasure of doing my graduate work at Duke University (which is to Bayesian statistics approximately what the Vatican is to Catholicism), I noticed that the loudest loudmouths about the superiority of Bayesian statistics aren't the profs... it's the newbie students who are relieved not to have to memorize the definition of the weird Frequentist confidence interval anymore (the Bayesian credible interval is so much easier). The profs understand that "better" depends on why you're trying to do. They spend a lot of time thinking in the Bayesian way because it fits the kind of decision approaches they're interested in.
So, my advice? Don't pick a side. See them as two different approaches that fit two different styles of decision-making and reasoning, then leave yourself the option of using whichever one suits the mindset/context you find yourself in.
Комментарии