filmov
tv
Digital Chemistry and Chemputation | by Prof. Lee Cronin
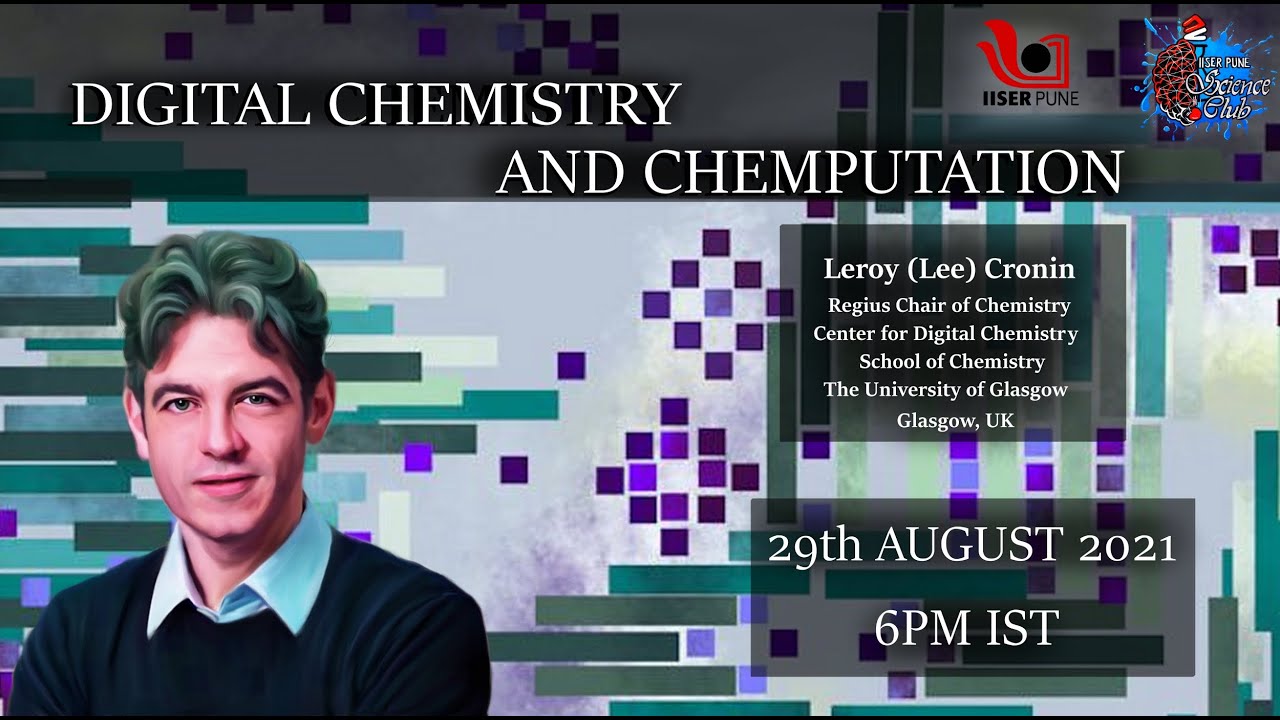
Показать описание
Today it is possible to design and synthesize many of the physically allowed molecules and materials conceivable if practical, yet paradoxically it is not possible to reproduce or rerun these successful procedures with high reliability. This is because many of the conditions devised for the manual or semi-manual synthesis are not uniformly recorded. The situation is even worse when the literature is investigated. For example, our preliminary studies reveal that more than 80% of all the published procedures fail to replicate without the help of an expert.
In this talk I will outline how we have solved this problem by devising a universal approach to chemical synthesis that allows us to translate all procedures, manual or automatic, to a new interchange format, XDL, that allows chemistry to be universally communicated. Furthermore, this new approach maps into a universal programming language for chemistry that is accessible to ALL synthetic chemists and will work on ALL robotic systems (subject to suitable specification). We demonstrate that the process is universal, and by analogy with computation, we call systems capable of universally turning code into reliable chemistry and materials processes Chemputation, see Figure.
Figure: Depiction of a chemical state machine (CSM) for synthesis that is capable of Chemputation. The input is a combination of reagents, process information and hardware addresses. The CSM organizes the reagents and the processes by using a scheduler that then gets executed in the hardware as a function of the available state until the product is formed.
I will also explain why our Chemical Synthesis Machine is really a Chemical State* Machine (CSM) and why you should care. This because our CSM can be used with AI and machine learning to search for new reactivity, reactions, materials, and molecules – that once discovered, can be reproduced using the CSM. This allows us to build the ultimate system that can both reproduce, search, discover, and update chemical knowledge autonomously in real time. I will show this working with real world examples from energy materials to small molecule drug candidates.
References:
[1] J. Granda, L. Donina, V. Dragone, D. –L. Long, L. Cronin 'Controlling an organic synthesis robot with machine learning to search for new reactivity', Nature, 2018, 559, 377-381.
[2] P. Kitson, G. Marie, J. –P. Francoia, S. Zalesskiy, R. Sigerson, J. S. Mathieson, L. Cronin 'Digitization of multistep organic synthesis in reactionware for on-demand pharmaceuticals', Science, 2018, 359, 314-319.
[3] S. Steiner, J. Wolf, S. Glatzel, A. Andreou, J. Granda, G. Keenan, T. Hinkley, G. Aragon-Camarasa, P. J. Kitson, D. Angelone, L. Cronin 'Organic synthesis in a modular robotic system driven by a chemical programming language', Science, 2019, 363, 144-152
[4] P. S. Gromski, A. Henson, J. Granda, L. Cronin 'How to explore chemical space using algorithms and automation', Nat Rev Chem., 2019, 3, 119-128.
[5] S. Hessam M. Mehr, M. Craven, A. Leonov, G. Keenan, L. Cronin 'A universal system for digitization and automatic execution of the chemical synthesis literature', Science, 2020, 370, 101-108.
In this talk I will outline how we have solved this problem by devising a universal approach to chemical synthesis that allows us to translate all procedures, manual or automatic, to a new interchange format, XDL, that allows chemistry to be universally communicated. Furthermore, this new approach maps into a universal programming language for chemistry that is accessible to ALL synthetic chemists and will work on ALL robotic systems (subject to suitable specification). We demonstrate that the process is universal, and by analogy with computation, we call systems capable of universally turning code into reliable chemistry and materials processes Chemputation, see Figure.
Figure: Depiction of a chemical state machine (CSM) for synthesis that is capable of Chemputation. The input is a combination of reagents, process information and hardware addresses. The CSM organizes the reagents and the processes by using a scheduler that then gets executed in the hardware as a function of the available state until the product is formed.
I will also explain why our Chemical Synthesis Machine is really a Chemical State* Machine (CSM) and why you should care. This because our CSM can be used with AI and machine learning to search for new reactivity, reactions, materials, and molecules – that once discovered, can be reproduced using the CSM. This allows us to build the ultimate system that can both reproduce, search, discover, and update chemical knowledge autonomously in real time. I will show this working with real world examples from energy materials to small molecule drug candidates.
References:
[1] J. Granda, L. Donina, V. Dragone, D. –L. Long, L. Cronin 'Controlling an organic synthesis robot with machine learning to search for new reactivity', Nature, 2018, 559, 377-381.
[2] P. Kitson, G. Marie, J. –P. Francoia, S. Zalesskiy, R. Sigerson, J. S. Mathieson, L. Cronin 'Digitization of multistep organic synthesis in reactionware for on-demand pharmaceuticals', Science, 2018, 359, 314-319.
[3] S. Steiner, J. Wolf, S. Glatzel, A. Andreou, J. Granda, G. Keenan, T. Hinkley, G. Aragon-Camarasa, P. J. Kitson, D. Angelone, L. Cronin 'Organic synthesis in a modular robotic system driven by a chemical programming language', Science, 2019, 363, 144-152
[4] P. S. Gromski, A. Henson, J. Granda, L. Cronin 'How to explore chemical space using algorithms and automation', Nat Rev Chem., 2019, 3, 119-128.
[5] S. Hessam M. Mehr, M. Craven, A. Leonov, G. Keenan, L. Cronin 'A universal system for digitization and automatic execution of the chemical synthesis literature', Science, 2020, 370, 101-108.