filmov
tv
Neural Networks are Decision Trees (w/ Alexander Mattick)
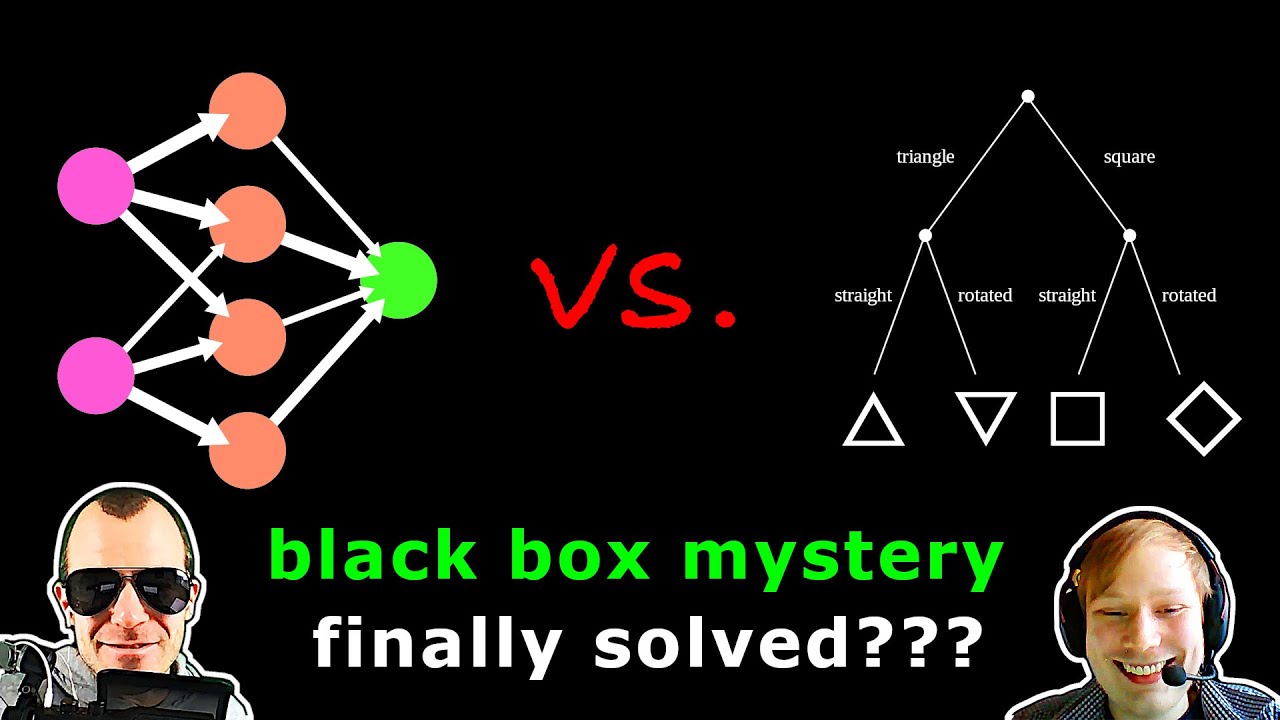
Показать описание
#neuralnetworks #machinelearning #ai
Alexander Mattick joins me to discuss the paper "Neural Networks are Decision Trees", which has generated a lot of hype on social media. We ask the question: Has this paper solved one of the large mysteries of deep learning and opened the black-box neural networks up to interpretability?
OUTLINE:
0:00 - Introduction
2:20 - Aren't Neural Networks non-linear?
5:20 - What does it all mean?
8:00 - How large do these trees get?
11:50 - Decision Trees vs Neural Networks
17:15 - Is this paper new?
22:20 - Experimental results
27:30 - Can Trees and Networks work together?
Abstract:
In this manuscript, we show that any feedforward neural network having piece-wise linear activation functions can be represented as a decision tree. The representation is equivalence and not an approximation, thus keeping the accuracy of the neural network exactly as is. We believe that this work paves the way to tackle the black-box nature of neural networks. We share equivalent trees of some neural networks and show that besides providing interpretability, tree representation can also achieve some computational advantages. The analysis holds both for fully connected and convolutional networks, which may or may not also include skip connections and/or normalizations.
Author: Caglar Aytekin
Links:
If you want to support me, the best thing to do is to share out the content :)
If you want to support me financially (completely optional and voluntary, but a lot of people have asked for this):
Bitcoin (BTC): bc1q49lsw3q325tr58ygf8sudx2dqfguclvngvy2cq
Ethereum (ETH): 0x7ad3513E3B8f66799f507Aa7874b1B0eBC7F85e2
Litecoin (LTC): LQW2TRyKYetVC8WjFkhpPhtpbDM4Vw7r9m
Monero (XMR): 4ACL8AGrEo5hAir8A9CeVrW8pEauWvnp1WnSDZxW7tziCDLhZAGsgzhRQABDnFy8yuM9fWJDviJPHKRjV4FWt19CJZN9D4n
Alexander Mattick joins me to discuss the paper "Neural Networks are Decision Trees", which has generated a lot of hype on social media. We ask the question: Has this paper solved one of the large mysteries of deep learning and opened the black-box neural networks up to interpretability?
OUTLINE:
0:00 - Introduction
2:20 - Aren't Neural Networks non-linear?
5:20 - What does it all mean?
8:00 - How large do these trees get?
11:50 - Decision Trees vs Neural Networks
17:15 - Is this paper new?
22:20 - Experimental results
27:30 - Can Trees and Networks work together?
Abstract:
In this manuscript, we show that any feedforward neural network having piece-wise linear activation functions can be represented as a decision tree. The representation is equivalence and not an approximation, thus keeping the accuracy of the neural network exactly as is. We believe that this work paves the way to tackle the black-box nature of neural networks. We share equivalent trees of some neural networks and show that besides providing interpretability, tree representation can also achieve some computational advantages. The analysis holds both for fully connected and convolutional networks, which may or may not also include skip connections and/or normalizations.
Author: Caglar Aytekin
Links:
If you want to support me, the best thing to do is to share out the content :)
If you want to support me financially (completely optional and voluntary, but a lot of people have asked for this):
Bitcoin (BTC): bc1q49lsw3q325tr58ygf8sudx2dqfguclvngvy2cq
Ethereum (ETH): 0x7ad3513E3B8f66799f507Aa7874b1B0eBC7F85e2
Litecoin (LTC): LQW2TRyKYetVC8WjFkhpPhtpbDM4Vw7r9m
Monero (XMR): 4ACL8AGrEo5hAir8A9CeVrW8pEauWvnp1WnSDZxW7tziCDLhZAGsgzhRQABDnFy8yuM9fWJDviJPHKRjV4FWt19CJZN9D4n
Комментарии