filmov
tv
Stable Diffusion XL (SDXL) Locally On Your PC - 8GB VRAM - Easy Tutorial With Automatic Installer
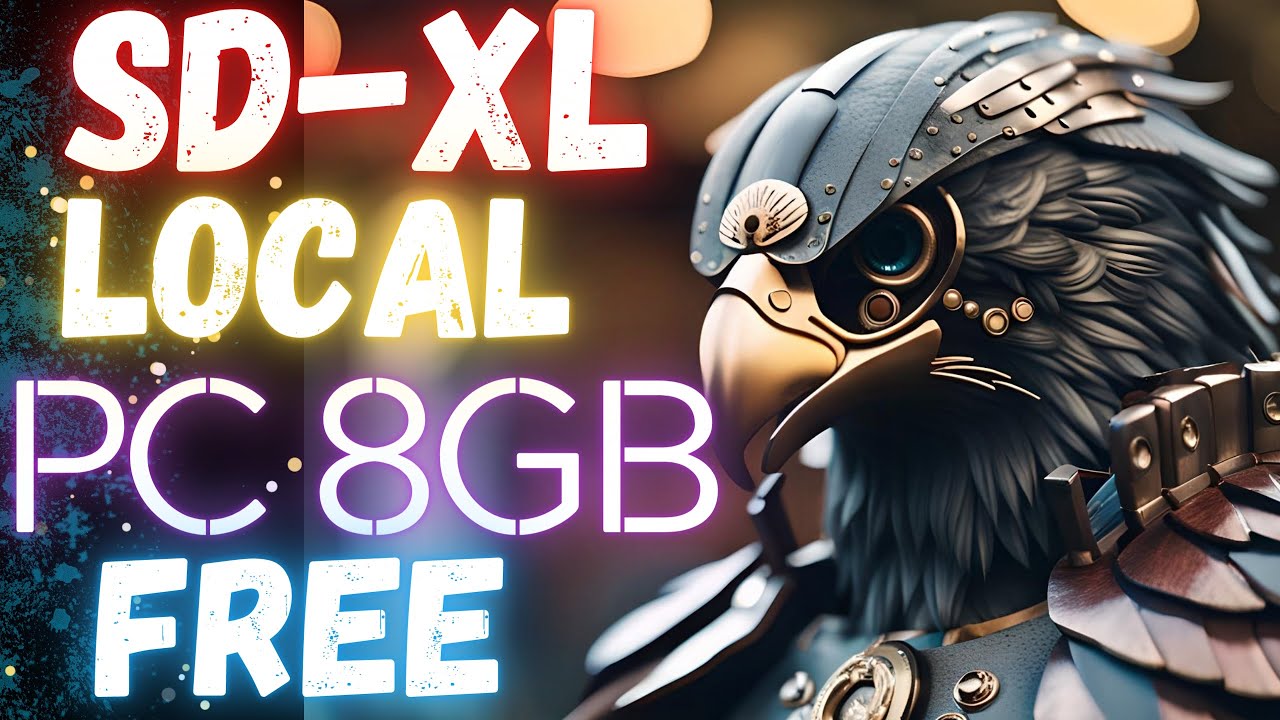
Показать описание
#SDXL is currently in beta and in this video I will show you how to use it install it on your PC. This tutorial should work on all devices including Windows, Unix, Mac even may work with AMD but I couldn't test it. I also have shown settings for 8GB VRAM so don't forget to watch that chapter.
Source GitHub Readme File ⤵️
Automatic Installer Script File ⤵️
Our Discord server ⤵️
If I have been of assistance to you and you would like to show your support for my work, please consider becoming a patron on 🥰 ⤵️
Technology & Science: News, Tips, Tutorials, Tricks, Best Applications, Guides, Reviews ⤵️
Playlist of #StableDiffusion Tutorials, Automatic1111 and Google Colab Guides, DreamBooth, Textual Inversion / Embedding, LoRA, AI Upscaling, Pix2Pix, Img2Img ⤵️
0:00 How to use SDXL locally on your PC
1:01 How to install via Automatic installer script
1:35 Beginning manual installation
1:47 How to accept terms and conditions to access SDXL weights and model files (instantly approved)
2:08 How agreement page looks like and how to fill form for instant access
2:38 How to generate Hugging Face access token
2:53 Continuing the manual installation
3:36 Automatic installation is completed. How to start using SDXL
4:00 How to add your Hugging Face token so that Gradio will work
4:45 Continuing the manual installation
5:19 Manual installation is completed. How to start using SDXL
6:17 How to delete cached model and weight files
6:44 How the app will download weight files showing live
7:20 Advanced settings of the Gradio APP of SDXL
8:11 Speed of image generation with RTX 3090 TI
8:39 Where are the generated images are saved
9:44 8 GB VRAM settings - min VRAM settings for SDXL
10:06 How to see file extensions on Windows
SDXL: Improving Latent Diffusion Models for High-Resolution Image Synthesis
Abstract
We present SDXL, a latent diffusion model for text-to-image synthesis. Compared
to previous versions of Stable Diffusion, SDXL leverages a three times larger UNet
backbone: The increase of model parameters is mainly due to more attention blocks
and a larger cross-attention context as SDXL uses a second text encoder. We design
multiple novel conditioning schemes and train SDXL on multiple aspect ratios.
We also introduce a refinement model which is used to improve the visual fidelity
of samples generated by SDXL using a post-hoc image-to-image technique. We
demonstrate that SDXL shows drastically improved performance compared the
previous versions of Stable Diffusion and achieves results competitive with those
of black-box state-of-the-art image generators. In the spirit of promoting open
research and fostering transparency in large model training and evaluation, we
provide access to code and model weights.
The last year has brought enormous leaps in deep generative modeling across various data domains,
such as natural language [50], audio [17], and visual media [38, 37, 40, 44, 15, 3, 7]. In this report,
we focus on the latter and unveil SDXL, a drastically improved version of Stable Diffusion. Stable
Diffusion is a latent text-to-image diffusion model (DM), which serves as the foundation for an
array of recent advancements in, e.g., 3D classification [43], controllable image editing [54], image
personalization [10], synthetic data augmentation [48], graphical user interface prototyping [51], etc.
Remarkably, the scope of applications has been extraordinarily extensive, encompassing fields as
diverse as music generation [9] and reconstructing images from fMRI brain scans [49].
User studies demonstrate that SDXL consistently surpasses all previous versions of Stable Diffusion
by a significant margin (see Fig. 1). In this report, we present the design choices which lead to this
boost in performance encompassing i) a 3× larger UNet-backbone compared to previous Stable
Diffusion models (Sec. 2.1), ii) two simple yet effective additional conditioning techniques (Sec. 2.2)
which do not require any form of additional supervision, and iii) a separate diffusion-based refinement
model which applies a noising-denoising process [28] to the latents produced by SDXL to improve
the visual quality of its samples (Sec. 2.5).
A major concern in the field of visual media creation is that while black-box-models are often
recognized as state-of-the-art, the opacity of their architecture prevents faithfully assessing and
validating their performance.
thumb photo taken from twitter : stonekaiju
Source GitHub Readme File ⤵️
Automatic Installer Script File ⤵️
Our Discord server ⤵️
If I have been of assistance to you and you would like to show your support for my work, please consider becoming a patron on 🥰 ⤵️
Technology & Science: News, Tips, Tutorials, Tricks, Best Applications, Guides, Reviews ⤵️
Playlist of #StableDiffusion Tutorials, Automatic1111 and Google Colab Guides, DreamBooth, Textual Inversion / Embedding, LoRA, AI Upscaling, Pix2Pix, Img2Img ⤵️
0:00 How to use SDXL locally on your PC
1:01 How to install via Automatic installer script
1:35 Beginning manual installation
1:47 How to accept terms and conditions to access SDXL weights and model files (instantly approved)
2:08 How agreement page looks like and how to fill form for instant access
2:38 How to generate Hugging Face access token
2:53 Continuing the manual installation
3:36 Automatic installation is completed. How to start using SDXL
4:00 How to add your Hugging Face token so that Gradio will work
4:45 Continuing the manual installation
5:19 Manual installation is completed. How to start using SDXL
6:17 How to delete cached model and weight files
6:44 How the app will download weight files showing live
7:20 Advanced settings of the Gradio APP of SDXL
8:11 Speed of image generation with RTX 3090 TI
8:39 Where are the generated images are saved
9:44 8 GB VRAM settings - min VRAM settings for SDXL
10:06 How to see file extensions on Windows
SDXL: Improving Latent Diffusion Models for High-Resolution Image Synthesis
Abstract
We present SDXL, a latent diffusion model for text-to-image synthesis. Compared
to previous versions of Stable Diffusion, SDXL leverages a three times larger UNet
backbone: The increase of model parameters is mainly due to more attention blocks
and a larger cross-attention context as SDXL uses a second text encoder. We design
multiple novel conditioning schemes and train SDXL on multiple aspect ratios.
We also introduce a refinement model which is used to improve the visual fidelity
of samples generated by SDXL using a post-hoc image-to-image technique. We
demonstrate that SDXL shows drastically improved performance compared the
previous versions of Stable Diffusion and achieves results competitive with those
of black-box state-of-the-art image generators. In the spirit of promoting open
research and fostering transparency in large model training and evaluation, we
provide access to code and model weights.
The last year has brought enormous leaps in deep generative modeling across various data domains,
such as natural language [50], audio [17], and visual media [38, 37, 40, 44, 15, 3, 7]. In this report,
we focus on the latter and unveil SDXL, a drastically improved version of Stable Diffusion. Stable
Diffusion is a latent text-to-image diffusion model (DM), which serves as the foundation for an
array of recent advancements in, e.g., 3D classification [43], controllable image editing [54], image
personalization [10], synthetic data augmentation [48], graphical user interface prototyping [51], etc.
Remarkably, the scope of applications has been extraordinarily extensive, encompassing fields as
diverse as music generation [9] and reconstructing images from fMRI brain scans [49].
User studies demonstrate that SDXL consistently surpasses all previous versions of Stable Diffusion
by a significant margin (see Fig. 1). In this report, we present the design choices which lead to this
boost in performance encompassing i) a 3× larger UNet-backbone compared to previous Stable
Diffusion models (Sec. 2.1), ii) two simple yet effective additional conditioning techniques (Sec. 2.2)
which do not require any form of additional supervision, and iii) a separate diffusion-based refinement
model which applies a noising-denoising process [28] to the latents produced by SDXL to improve
the visual quality of its samples (Sec. 2.5).
A major concern in the field of visual media creation is that while black-box-models are often
recognized as state-of-the-art, the opacity of their architecture prevents faithfully assessing and
validating their performance.
thumb photo taken from twitter : stonekaiju
Комментарии