filmov
tv
DeepFT: Self-supervised fault tolerance (IEEE INFOCOM 2023)
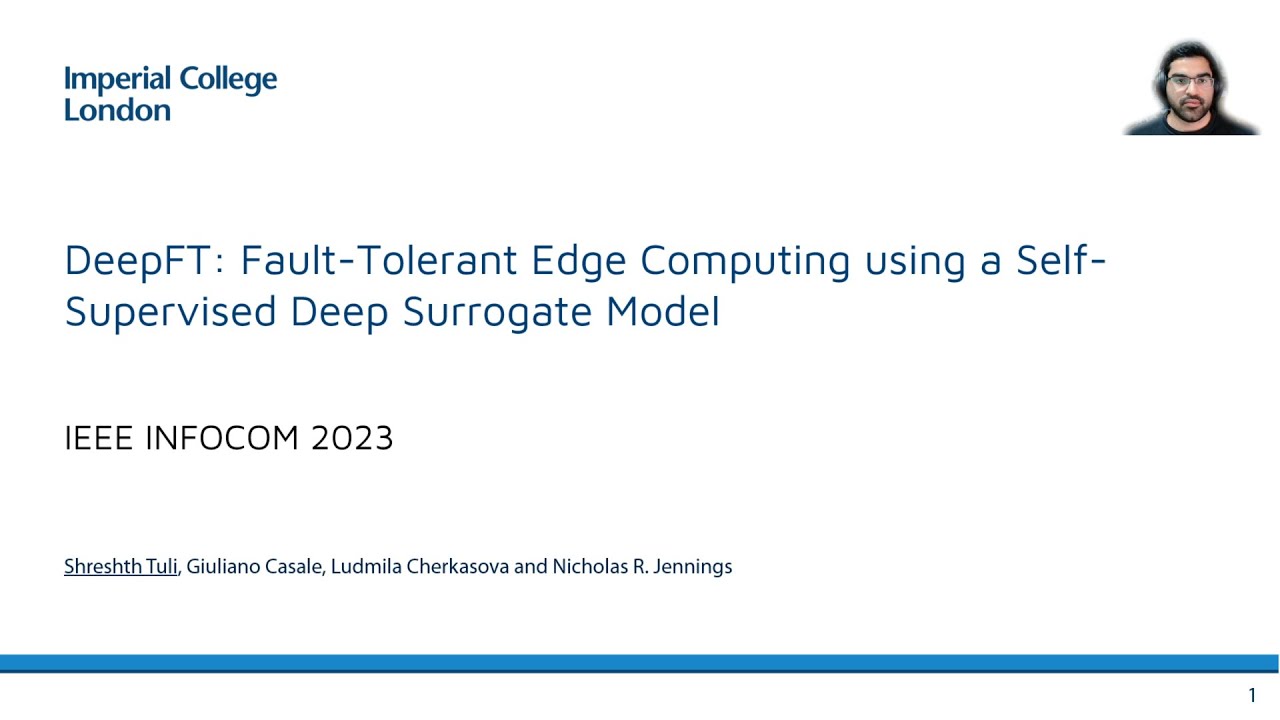
Показать описание
This video presents our work, namely DeepFT. This work has been accepted in IEEE INFOCOM 2023.
Abstract: The emergence of latency-critical AI applications has been supported by the evolution of the edge computing paradigm. However, edge solutions are typically resource-constrained, posing reliability challenges due to heightened contention for compute capacities and faulty application behavior in the presence of overload conditions. Although a large amount of generated log data can be mined for fault prediction, labeling this data for training is a manual process and thus a limiting factor for automation. Due to this, many companies resort to unsupervised fault-tolerance models. Yet, failure models of this kind can incur a loss of accuracy when they need to adapt to non-stationary workloads and diverse host characteristics. Thus, we propose a novel modeling approach, DeepFT, to proactively avoid system overloads and their adverse effects by optimizing the task scheduling decisions. DeepFT uses a deep-surrogate model to accurately predict and diagnose faults in the system and co-simulation based self-supervised learning to dynamically adapt the model in volatile settings. Experimentation on an edge cluster shows that DeepFT can outperform state-of-the-art methods in fault-detection and QoS metrics. Specifically, DeepFT gives the highest F1 scores for fault-detection, reducing service deadline violations by up to 37% while also improving response time by up to 9%.
Abstract: The emergence of latency-critical AI applications has been supported by the evolution of the edge computing paradigm. However, edge solutions are typically resource-constrained, posing reliability challenges due to heightened contention for compute capacities and faulty application behavior in the presence of overload conditions. Although a large amount of generated log data can be mined for fault prediction, labeling this data for training is a manual process and thus a limiting factor for automation. Due to this, many companies resort to unsupervised fault-tolerance models. Yet, failure models of this kind can incur a loss of accuracy when they need to adapt to non-stationary workloads and diverse host characteristics. Thus, we propose a novel modeling approach, DeepFT, to proactively avoid system overloads and their adverse effects by optimizing the task scheduling decisions. DeepFT uses a deep-surrogate model to accurately predict and diagnose faults in the system and co-simulation based self-supervised learning to dynamically adapt the model in volatile settings. Experimentation on an edge cluster shows that DeepFT can outperform state-of-the-art methods in fault-detection and QoS metrics. Specifically, DeepFT gives the highest F1 scores for fault-detection, reducing service deadline violations by up to 37% while also improving response time by up to 9%.
Комментарии