filmov
tv
Reasoning about Uncertainty in High-dimensional Data Analysis
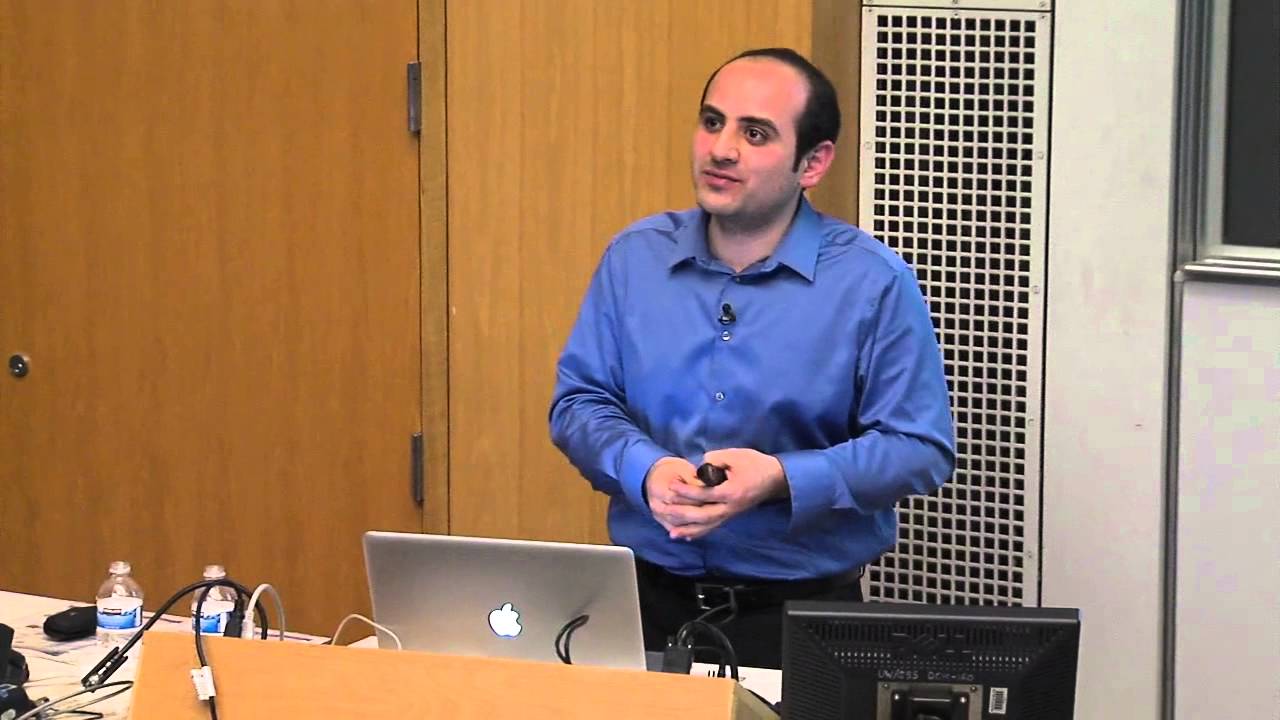
Показать описание
Modern technologies generate vast amounts of data at unprecedented speed. This ubiquitous technological trend is driving the need for increasingly sophisticated algorithms to find subtle statistical patterns in massive amounts of data, and extract actionable information. Examples of such problems arise in healthcare, social networks, and recommendation systems.
The most useful statistical models in this context are high-dimensional or over-parameterized: the number of parameters to estimate is far larger than the number of samples. Parameters are estimated using convex optimization or iterative algorithms. As a consequence, it is extremely challenging to quantify the uncertainty associated with a certain parameter estimate. Uncertainty assessment, however, is crucial whenever we intend to take actions on the basis of our statistical model of the data.
Over the last two years, this problem has attracted the attention of several groups. In this talk, I will propose an efficient method to construct confidence intervals for single regression coefficients. The resulting confidence intervals have nearly optimal size.
I will review applications to healthcare analytics, medical imaging and decision-making, and discuss future perspectives for this research area.
Adel Javanmard
Stanford University
02/13/2014
The most useful statistical models in this context are high-dimensional or over-parameterized: the number of parameters to estimate is far larger than the number of samples. Parameters are estimated using convex optimization or iterative algorithms. As a consequence, it is extremely challenging to quantify the uncertainty associated with a certain parameter estimate. Uncertainty assessment, however, is crucial whenever we intend to take actions on the basis of our statistical model of the data.
Over the last two years, this problem has attracted the attention of several groups. In this talk, I will propose an efficient method to construct confidence intervals for single regression coefficients. The resulting confidence intervals have nearly optimal size.
I will review applications to healthcare analytics, medical imaging and decision-making, and discuss future perspectives for this research area.
Adel Javanmard
Stanford University
02/13/2014
Комментарии