filmov
tv
Filling Missing Climate Data Using Arithmetic mean method, Inverse Distance Weighting method MCMC
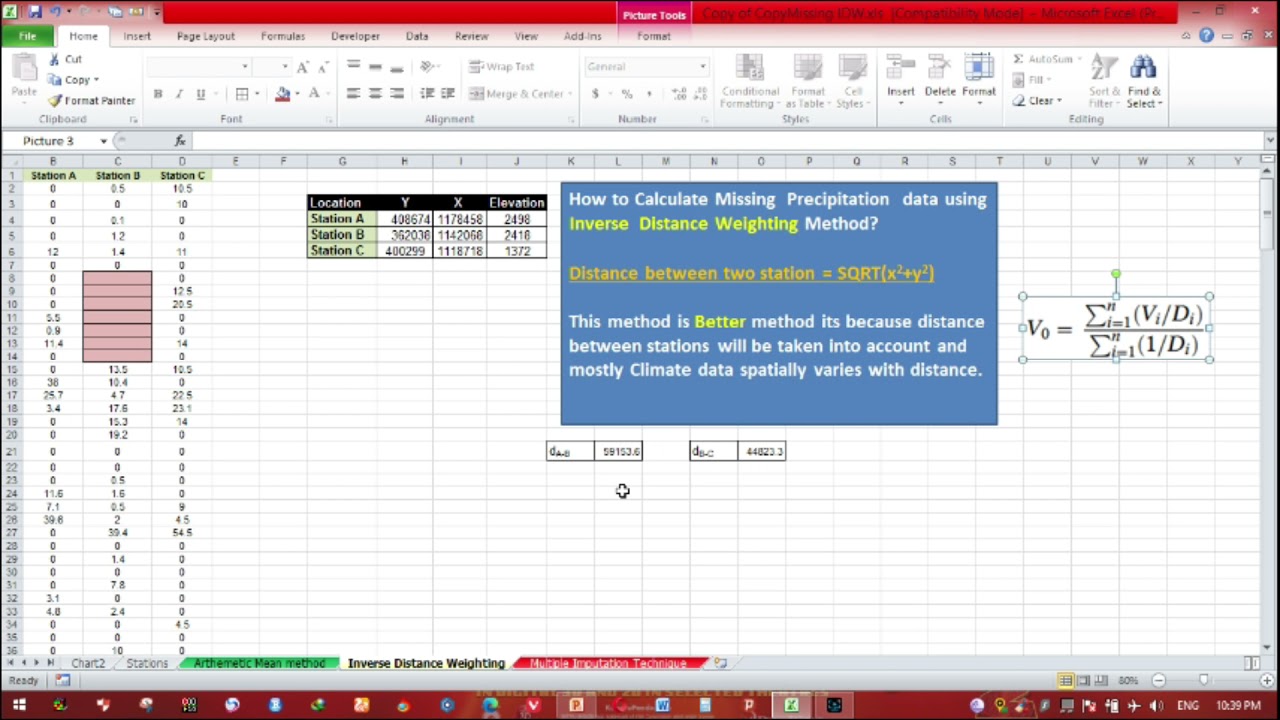
Показать описание
To know the different kind of methods used download the book using the link:
The arithmetic mean is the simplest and most widely used measure of a mean, or average. It simply involves taking the sum of a group of numbers, then dividing that sum by the count of the numbers used in the series.
Inverse distance weighting (IDW) is a type of deterministic method for multivariate interpolation with a known scattered set of points. The assigned values to unknown points are calculated with a weighted average of the values available at the known points.
Multiple Imputation is a Markov chain Monte Carlo technique developed to work out missing data problems.
Conclusion: The advantages of IDW are that it is simple, easy to understand, and efficient. Disadvantages are that it is sensitive to outliers and there is no indication of error.
The arithmetic mean is the simplest and most widely used measure of a mean, or average. It simply involves taking the sum of a group of numbers, then dividing that sum by the count of the numbers used in the series.
Inverse distance weighting (IDW) is a type of deterministic method for multivariate interpolation with a known scattered set of points. The assigned values to unknown points are calculated with a weighted average of the values available at the known points.
Multiple Imputation is a Markov chain Monte Carlo technique developed to work out missing data problems.
Conclusion: The advantages of IDW are that it is simple, easy to understand, and efficient. Disadvantages are that it is sensitive to outliers and there is no indication of error.
Filling Missing Climate Data Using Arithmetic mean method, Inverse Distance Weighting method MCMC
Fill the Gaps: Explore the Estimate Missing Climate Data Tool in Action
How to Filling Missing Values of Hydro Climatic Data Using Different Methods in XLSTAT
Checking filling of missing temperature data by Long Term average method
ESTIMATING MISSING PRECIPITATION DATA USING SIMPLE LINEAR REGRESSION DATA | HYRDROMETEOROLOGY
2. Filling Missed Data using XLSTAT Monte Carlo Multiple Imputation Technique
Climate Data (Temperature, Precipitation, Humidity) from 1981-2022
Linear Interpolation in Excel | Fill in Missing Values
Meteorological Data preparation part two
R-Instat: Climate Normals - Missing Values
Missing Data? No Problem!
Filling and handling missed data in R software
Intro to Webinar: Fill Missing Precipitation Data with Machine Learning in Python and Scikit-Learn
Meteorological Data preparation Part One
Fill Missing Precipitation Data with Machine Learning in Python and Scikit-Learn - Tutorial
Normal ratio method for filling missed data: meteorological data preparation part three
How to fill in missing data in Microsoft Excel
Missing Data Analysis | Xlstat Trial version|
Define missing values in XLSTAT - XLSTAT Tips
Download Historical and Future Climate Data (Temperature, Precipitation etc.)
R-Instat: Preparing Data - Missing Values Options
Estimation of Missing Data
Inverse distance method for filling missed data MDP part four
How to impute missing data using mice package in R programming
Комментарии