filmov
tv
RSS Journal webinar 22 January 2020
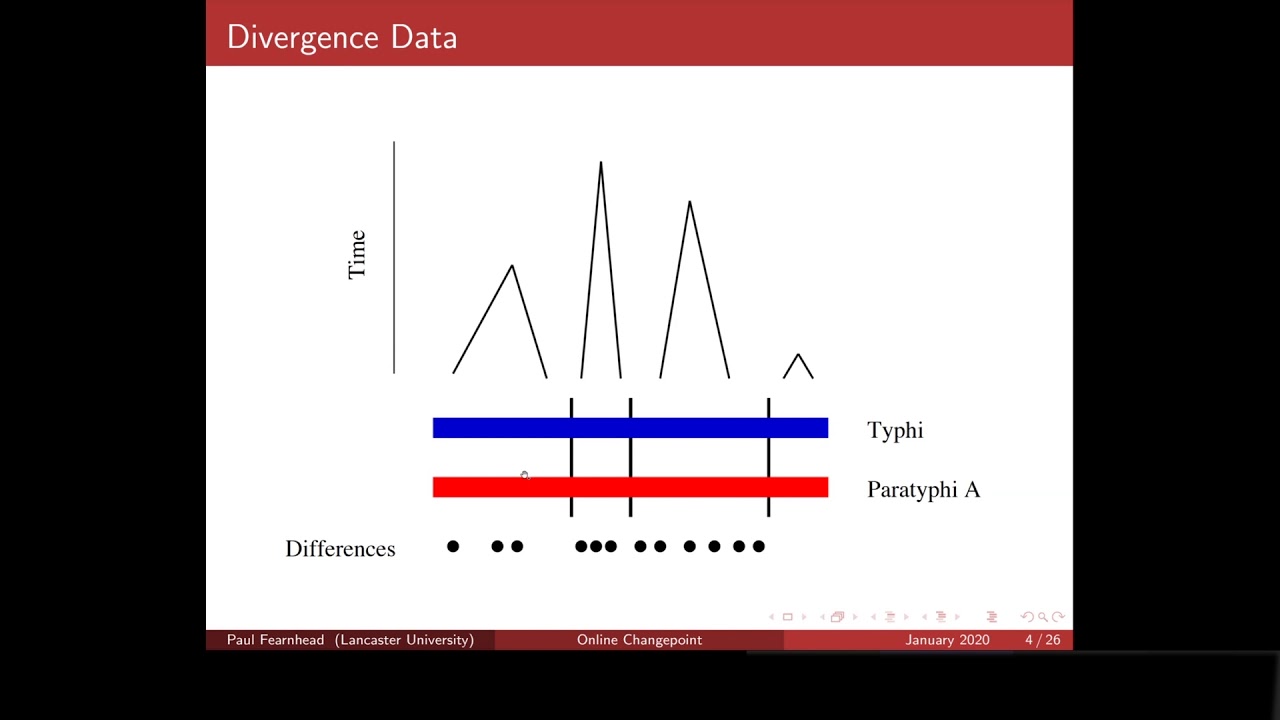
Показать описание
Paper 1: Online inference for multiple changepoint problems’ by Fearnhead & Liu.
It was published in Series B, Volume 69, Issue 4, September 2007
Presenter 1: Paul Fearnhead.
Abstract: We propose an on?line algorithm for exact filtering of multiple changepoint problems. This algorithm enables simulation from the true joint posterior distribution of the number and position of the changepoints for a class of changepoint models. The computational cost of this exact algorithm is quadratic in the number of observations. We further show how resampling ideas from particle filters can be used to reduce the computational cost to linear in the number of observations, at the expense of introducing small errors, and we propose two new, optimum resampling algorithms for this problem. One, a version of rejection control, allows the particle filter to choose the number of particles that are required at each time step automatically. The new resampling algorithms substantially outperform standard resampling algorithms on examples that we consider; and we demonstrate how the resulting particle filter is practicable for segmentation of human G+C content.
Paper 2: High dimensional change point estimation via sparse projection’ by Wang & Samworth.
It was published in Series B, Volume 80, Issue1 in January 2018
Presenter 2: Tengyao Wang
Abstract: Change points are a very common feature of ‘big data’ that arrive in the form of a data stream. We study high dimensional time series in which, at certain time points, the mean structure changes in a sparse subset of the co?ordinates. The challenge is to borrow strength across the co?ordinates to detect smaller changes than could be observed in any individual component series. We propose a two?stage procedure called inspect for estimation of the change points: first, we argue that a good projection direction can be obtained as the leading left singular vector of the matrix that solves a convex optimization problem derived from the cumulative sum transformation of the time series. We then apply an existing univariate change point estimation algorithm to the projected series. Our theory provides strong guarantees on both the number of estimated change points and the rates of convergence of their locations, and our numerical studies validate its highly competitive empirical performance for a wide range of data?generating mechanisms. Software implementing the methodology is available in the R package InspectChangepoint.
Chair: Yi Yu, University of Warwick
Discussant: Claudia Kirch, Otto von Guericke University Magdeburg
It was published in Series B, Volume 69, Issue 4, September 2007
Presenter 1: Paul Fearnhead.
Abstract: We propose an on?line algorithm for exact filtering of multiple changepoint problems. This algorithm enables simulation from the true joint posterior distribution of the number and position of the changepoints for a class of changepoint models. The computational cost of this exact algorithm is quadratic in the number of observations. We further show how resampling ideas from particle filters can be used to reduce the computational cost to linear in the number of observations, at the expense of introducing small errors, and we propose two new, optimum resampling algorithms for this problem. One, a version of rejection control, allows the particle filter to choose the number of particles that are required at each time step automatically. The new resampling algorithms substantially outperform standard resampling algorithms on examples that we consider; and we demonstrate how the resulting particle filter is practicable for segmentation of human G+C content.
Paper 2: High dimensional change point estimation via sparse projection’ by Wang & Samworth.
It was published in Series B, Volume 80, Issue1 in January 2018
Presenter 2: Tengyao Wang
Abstract: Change points are a very common feature of ‘big data’ that arrive in the form of a data stream. We study high dimensional time series in which, at certain time points, the mean structure changes in a sparse subset of the co?ordinates. The challenge is to borrow strength across the co?ordinates to detect smaller changes than could be observed in any individual component series. We propose a two?stage procedure called inspect for estimation of the change points: first, we argue that a good projection direction can be obtained as the leading left singular vector of the matrix that solves a convex optimization problem derived from the cumulative sum transformation of the time series. We then apply an existing univariate change point estimation algorithm to the projected series. Our theory provides strong guarantees on both the number of estimated change points and the rates of convergence of their locations, and our numerical studies validate its highly competitive empirical performance for a wide range of data?generating mechanisms. Software implementing the methodology is available in the R package InspectChangepoint.
Chair: Yi Yu, University of Warwick
Discussant: Claudia Kirch, Otto von Guericke University Magdeburg