filmov
tv
Invariant Representations- Daniel Moyer
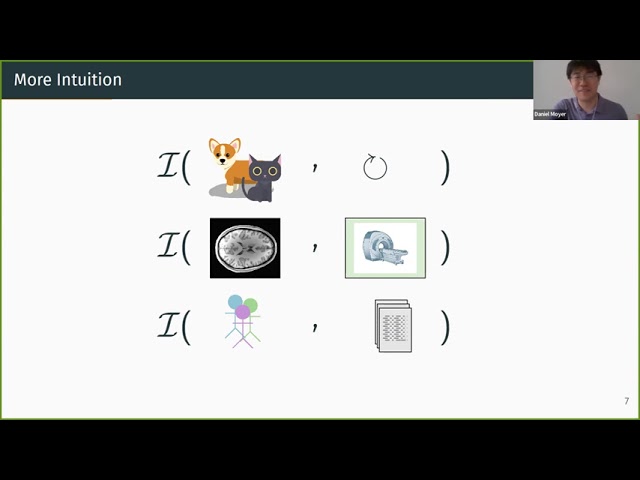
Показать описание
Abstract: The removal of unwanted information is a surprisingly common task. Removing potential biases in prediction problems, controlling the effects of covariates, and disentangling meaningful factors of variation all require the selective removal of information. In this talk, I will describe a method for constructing such representations by minimizing mutual information in a variational setting. This path also provides insight into adversarial methods and their training schema. We will then discuss applications and implications in multi-site MRI, style transfer, and fair representation.
Speaker Bio: Daniel Moyer will join the Computer Science Department at Vanderbilt University for the Fall 2022 semester as an Assistant Professor. Previously, he was a post-doc in CSAIL at MIT, working with Prof. Polina Golland on fetal MRI. He received his doctorate in 2019 from the University of Southern California under Paul Thompson and Greg Ver Steeg, where he worked on representation learning problems in diffusion MRI and neuroimaging.
Speaker Bio: Daniel Moyer will join the Computer Science Department at Vanderbilt University for the Fall 2022 semester as an Assistant Professor. Previously, he was a post-doc in CSAIL at MIT, working with Prof. Polina Golland on fetal MRI. He received his doctorate in 2019 from the University of Southern California under Paul Thompson and Greg Ver Steeg, where he worked on representation learning problems in diffusion MRI and neuroimaging.