filmov
tv
Data platform in a mesh architecture
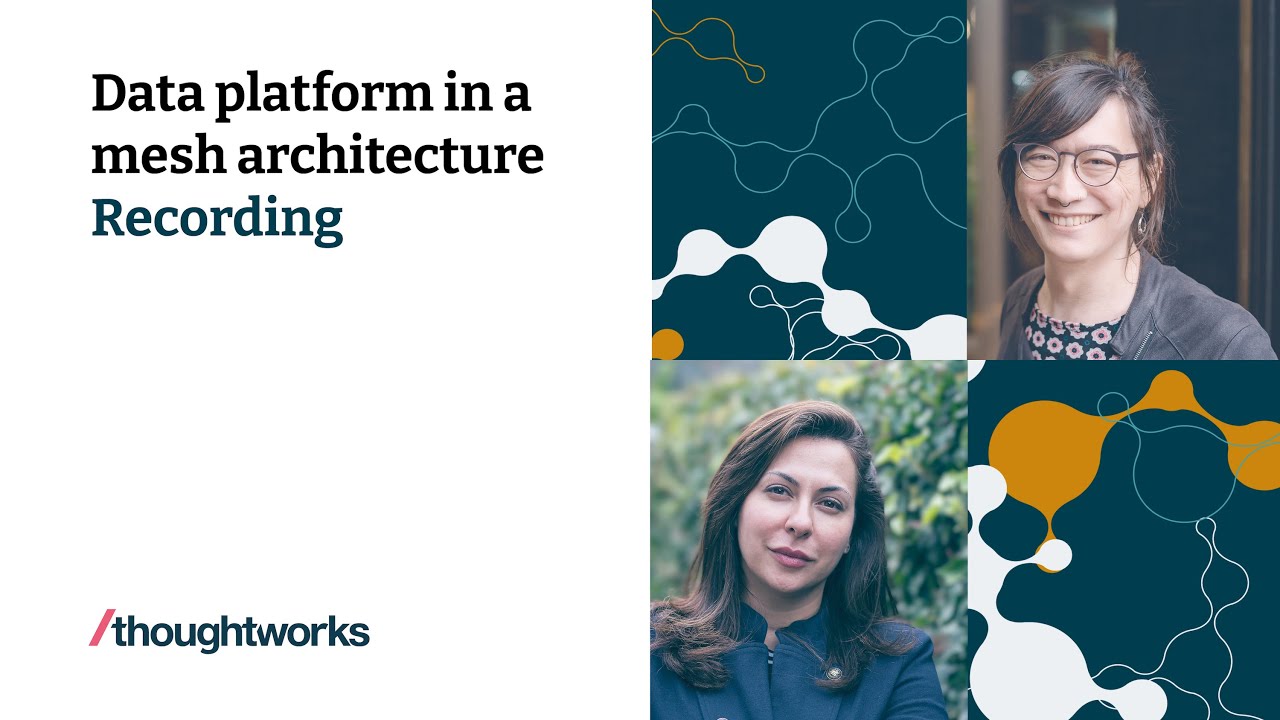
Показать описание
Data Mesh is a paradigm shift in big analytical data management that addresses some of the limitations of the past paradigms, data warehousing and data lake. Data Mesh is founded in four principles: "domain-driven ownership of data", "data as a product", "self-serve data platform" and a "federated computational governance".
In this talk Emily and Zhamak will talk about data platforms in a mesh architecture. They will cover topics such as what does it take to make data sets independently understandable? How do we empower individual domain teams to deliver data products, while lowering their cognitive load? How do we build a platform that enables data generalists and reduces the need for specialization?
In this talk Emily and Zhamak will talk about data platforms in a mesh architecture. They will cover topics such as what does it take to make data sets independently understandable? How do we empower individual domain teams to deliver data products, while lowering their cognitive load? How do we build a platform that enables data generalists and reduces the need for specialization?
Data platform in a mesh architecture
The role of self service platforms in data mesh – Pramod Sadalage
Data Mesh tutorial for beginners - What is Data Mesh ?
Data Mesh - The Fourth Generation Enterprise Data Platform
Modern Data Architectures | Data Mesh, Data Fabric, & Data Lakehouse
Data Mesh 101: What is Data Mesh?
Database vs Data Warehouse vs Data Lake | What is the Difference?
An End–to-End Data Mesh Platform for Innovation
Implement Data Mesh with a Self Serve Platform
Data Platform Modernization with a Data Mesh Architecture
Semantic Data Mesh: The Missing Links In Your Data Platform
Introduction to Data Mesh - Zhamak Dehghani
Data Mesh 101: Data Available Everywhere, Self-Service
Data Mesh Paradigm Shift in Data Platform Architecture - Arif Wider - DDD Europe 2020
AWS re:Invent 2022 - Building data mesh architectures on AWS (ANT336)
Showcase Data Mesh | Modern Data Platform at Vanderlande
Data Mesh TV: Building and maintaining the modern data platform
How to implement a Modern Data platform with Data Mesh Architecture Pattern
How Siemens AG Built an Enterprise-Wide Data Mesh Architecture on Snowflake
Platform Building for Data Mesh - Show me how it is done! by Tom De Wolf
Data Sharks #18: Kent Graziano from Snowflake on Data Mesh
Data Mesh Paradigm Shift in Data Platform Architecture
Data Mesh in Practice: How Europe’s Leading Online Platform for Fashion Goes Beyond the Data Lake
Build a Data Mesh on GCP with Dataplex
Комментарии