filmov
tv
Zhang-Wei Hong on Explore and Exploit Data in Reinforcement Learning | Toronto AIR Seminar
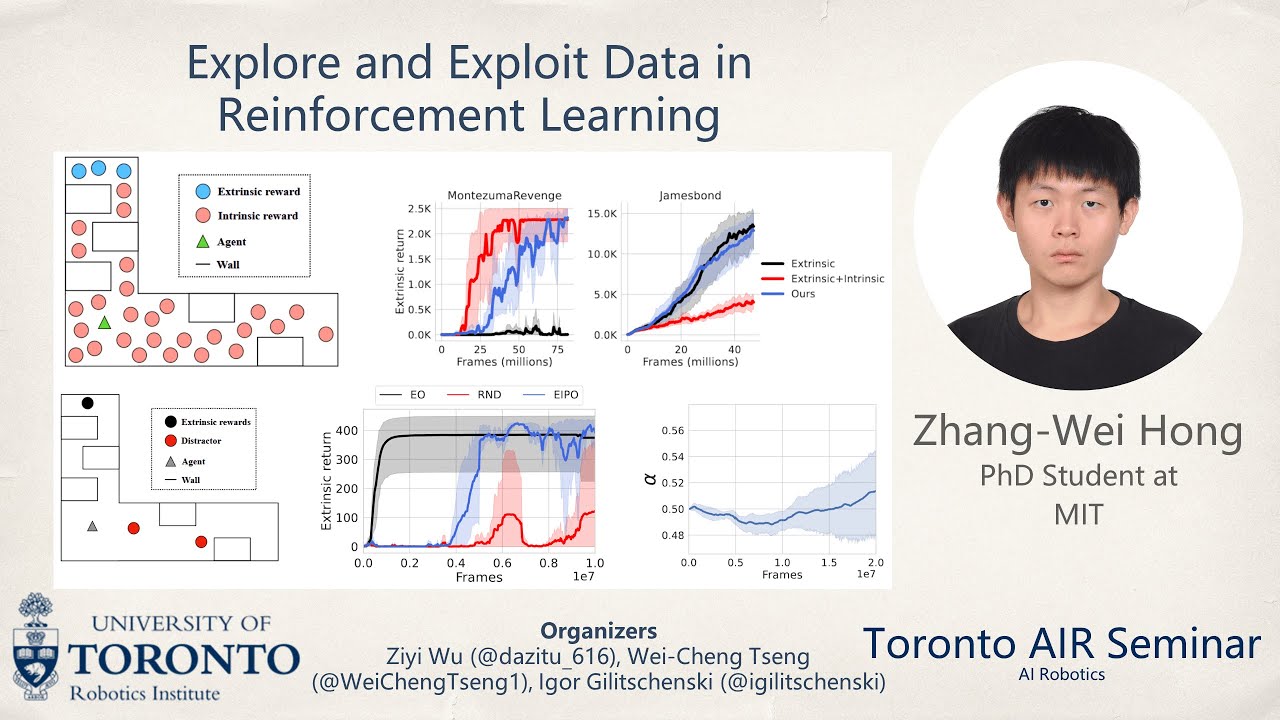
Показать описание
Abstract:
Reinforcement learning (RL) is a data-driven method for solving sequential decision-making problems from interaction experience with the environment. RL has shown to be able to learn non-trivial controllers in robot locomotion and manipulation that are challenging for model-based planning. However, the intensive data requirement prevents RL from being widely applied in robotics. Even training policies in simulators take several weeks to obtain a satisfactory policy. Prior works circumvent this data requirements using a curiosity-driven (a.k.a. exploration bonuses or intrinsic rewards) strategy to improve exploration (data collection) or learning from dataset (offline RL) curated by humans or pre-programmed controller. In this talk, I will illustrate the fallacy of curiosity-driven exploration strategy and sensitivity to data distribution of offline RL algorithms.
Paper:
Bio:
Zhang-Wei Hong is a Ph.D. candidate in the Department of Electrical Engineering and Computer Science at Massachusetts Institute of Technology (MIT). He received his B.S. and M.S. degrees from National Tsing Hua University in Taiwan and has conducted research internships at TU Darmstadt in Germany and Preferred Networks (PFN) in Japan. Zhang-Wei's research interests lie at the intersection of reinforcement learning and optimization, with a focus on developing principled algorithms to improve the usability of RL in real-world scenarios. His work has been published in top-tier conferences such as NeurIPS, ICLR, ICRA, and CoRL.
Toronto AIR Seminar:
The Toronto AI Robotics Seminar Series is a set of events featuring young robotics and AI experts. The talks are given by local as well as global speakers and organized by the Faculty and Students at University of Toronto’s Department of Computer Science. We welcome students, researchers and robotics enthusiasts from around the world to join us and interact with the Toronto Robotics Community.
Reinforcement learning (RL) is a data-driven method for solving sequential decision-making problems from interaction experience with the environment. RL has shown to be able to learn non-trivial controllers in robot locomotion and manipulation that are challenging for model-based planning. However, the intensive data requirement prevents RL from being widely applied in robotics. Even training policies in simulators take several weeks to obtain a satisfactory policy. Prior works circumvent this data requirements using a curiosity-driven (a.k.a. exploration bonuses or intrinsic rewards) strategy to improve exploration (data collection) or learning from dataset (offline RL) curated by humans or pre-programmed controller. In this talk, I will illustrate the fallacy of curiosity-driven exploration strategy and sensitivity to data distribution of offline RL algorithms.
Paper:
Bio:
Zhang-Wei Hong is a Ph.D. candidate in the Department of Electrical Engineering and Computer Science at Massachusetts Institute of Technology (MIT). He received his B.S. and M.S. degrees from National Tsing Hua University in Taiwan and has conducted research internships at TU Darmstadt in Germany and Preferred Networks (PFN) in Japan. Zhang-Wei's research interests lie at the intersection of reinforcement learning and optimization, with a focus on developing principled algorithms to improve the usability of RL in real-world scenarios. His work has been published in top-tier conferences such as NeurIPS, ICLR, ICRA, and CoRL.
Toronto AIR Seminar:
The Toronto AI Robotics Seminar Series is a set of events featuring young robotics and AI experts. The talks are given by local as well as global speakers and organized by the Faculty and Students at University of Toronto’s Department of Computer Science. We welcome students, researchers and robotics enthusiasts from around the world to join us and interact with the Toronto Robotics Community.