filmov
tv
Exposome Data Challenge - 2 day: Expo. analytics, Topic 3 Biomed. sci. & ML, Topic 4 Causal modeling
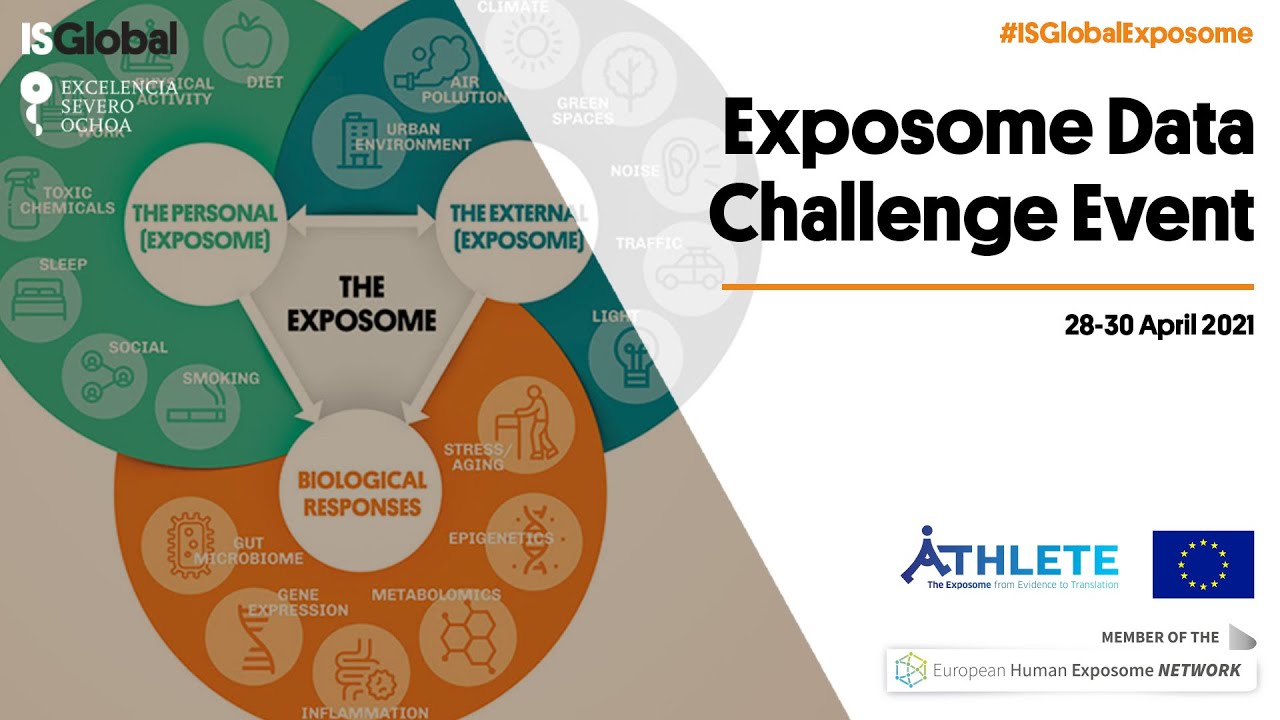
Показать описание
Keynote lecture
Exposome analytics: successes and current challenges
Marc Chadeau-Hyam, Imperial College London, United Kingdom
Topic 3: Biomedical data science & machine learning – Chair: Paula Petrone, ISGlobal
Clustering Exposure Trajectories to Classify Phenotypic Characteristics
Sejal Mistry, University of Utah
Exposure Continuum Mapping for predicting health and disease in exposome studies
John Pearce, Medical University of South Carolina
Deep-Exposome: A Predictive and Interpretative Deep Neural Network Ensemble for Exposome Data
Fei Zou, University of North Carolina, Chapel Hill
Leveraging machine learning and explainable AI to better understand exposomic data
Jean-Baptiste Guimbaud, LIRIS-ISGlobal
Using causal random forest to determine exposure environments with high sexual dimorphisms
Alejandro Caceres, ISGlobal
Searching for the risk factors for childhood overweight - A novel approach to identify the most relevant child BMI-associated exposures
Lucile Broséus and Paulina Jedynak, Université Grenoble Alpes
Discussion
Break
Topic 4: Causal modelling, mediation and triangulation – Chair: Léa Maitre, ISGlobal
Omics Modules for Exposome-Health Associations (OMEXA)
Carl Brunius, Chalmers University of Technology
Decoding unknown links between the exposome and health outcomes by multi-omics analysis
Xiaotao Shen, Stanford University
Meet-in-the-middle meets multi-omics: a strategy to identify molecular signatures of environmental drivers of childhood BMI
Congrong Wang, Hasselt University
Application of a novel method for mediation analysis in the exposome context
Daniela Zugna, University of Turin
Molecular Gatekeepers bridge the exposome and health
Miao Yu, Icahn School of Medicine at Mount Sinai
Discussion
Exposome analytics: successes and current challenges
Marc Chadeau-Hyam, Imperial College London, United Kingdom
Topic 3: Biomedical data science & machine learning – Chair: Paula Petrone, ISGlobal
Clustering Exposure Trajectories to Classify Phenotypic Characteristics
Sejal Mistry, University of Utah
Exposure Continuum Mapping for predicting health and disease in exposome studies
John Pearce, Medical University of South Carolina
Deep-Exposome: A Predictive and Interpretative Deep Neural Network Ensemble for Exposome Data
Fei Zou, University of North Carolina, Chapel Hill
Leveraging machine learning and explainable AI to better understand exposomic data
Jean-Baptiste Guimbaud, LIRIS-ISGlobal
Using causal random forest to determine exposure environments with high sexual dimorphisms
Alejandro Caceres, ISGlobal
Searching for the risk factors for childhood overweight - A novel approach to identify the most relevant child BMI-associated exposures
Lucile Broséus and Paulina Jedynak, Université Grenoble Alpes
Discussion
Break
Topic 4: Causal modelling, mediation and triangulation – Chair: Léa Maitre, ISGlobal
Omics Modules for Exposome-Health Associations (OMEXA)
Carl Brunius, Chalmers University of Technology
Decoding unknown links between the exposome and health outcomes by multi-omics analysis
Xiaotao Shen, Stanford University
Meet-in-the-middle meets multi-omics: a strategy to identify molecular signatures of environmental drivers of childhood BMI
Congrong Wang, Hasselt University
Application of a novel method for mediation analysis in the exposome context
Daniela Zugna, University of Turin
Molecular Gatekeepers bridge the exposome and health
Miao Yu, Icahn School of Medicine at Mount Sinai
Discussion