filmov
tv
Stéphane Mallat - Multiscale Models for Image Classification and Physics with Deep Networks
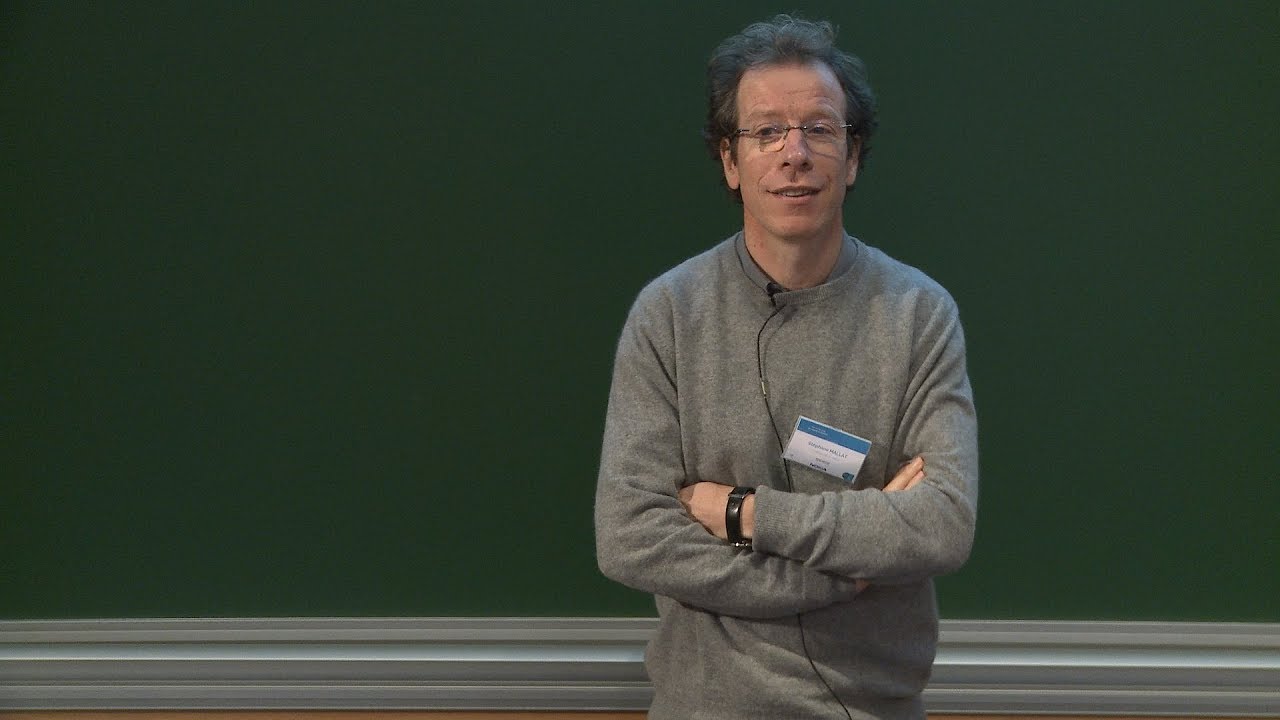
Показать описание
Abstract: Approximating high-dimensional functionals with low-dimensional models is a central issue of machine learning, image processing, physics and mathematics. Deep convolutional networks are able to approximate such functionals over a wide range of applications. This talk shows that these computational architectures take advantage of scale separation, symmetries and sparse representations. We introduce simplified architectures which can be anlalyzed mathematically. Scale separations is performed with wavelets and scale interactions are captured through phase coherence. We show applications to image classification and generation as well as regression of quantum molecular energies and modelization of turbulence flows.
––––––––––––––––––––––––––––––
Track: Wanderlust — CRASTEL [Audio Library Release]
Music provided by Audio Library Plus
––––––––––––––––––––––––––––––
––––––––––––––––––––––––––––––
Track: Wanderlust — CRASTEL [Audio Library Release]
Music provided by Audio Library Plus
––––––––––––––––––––––––––––––