filmov
tv
What's next in pgvector: Building AI-enabled apps with PostgreSQL - AWS Databases in 15
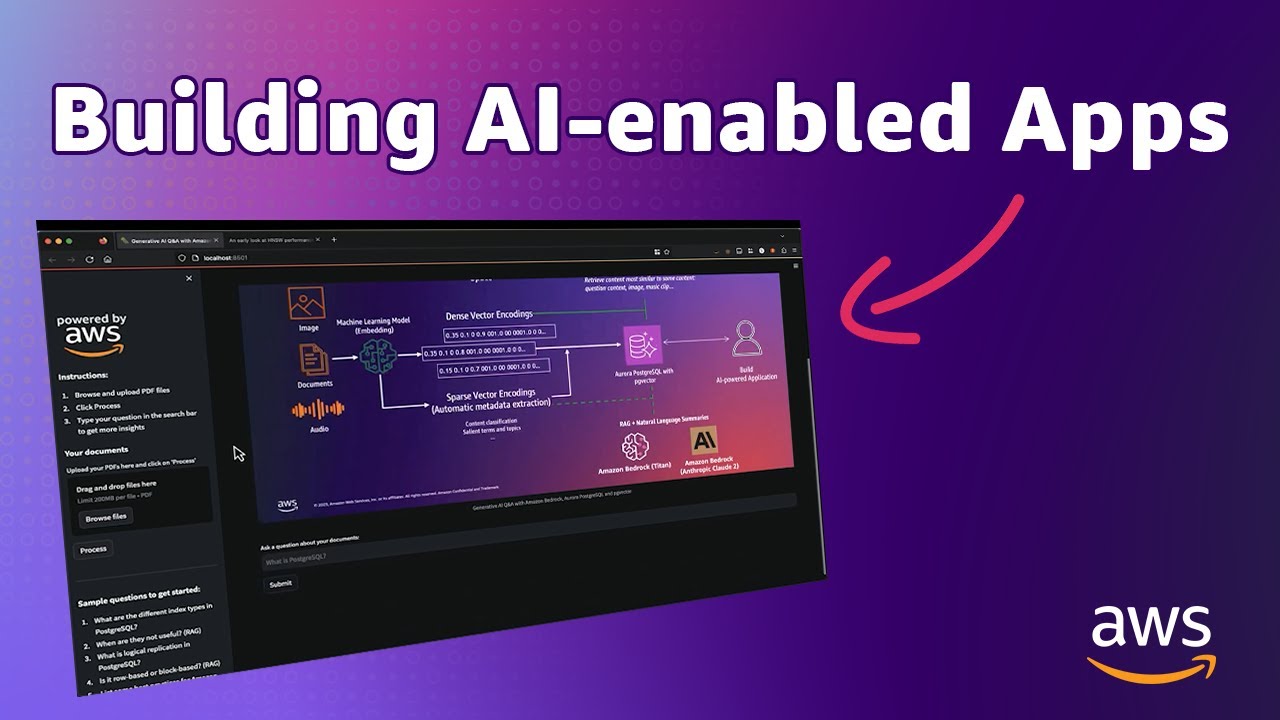
Показать описание
Generative AI and Large Language Models (LLMs) are powerful technologies for building applications with richer and more personalized user experiences. This year, Amazon Aurora and Amazon RDS announced support for pgvector, an extension that allows you to store embeddings from machine learning (ML) models in your database and to perform efficient similarity searches. In this session, we’ll briefly introduce you to pgvector, how it works, and why it’s important. AWS has had the opportunity to collaborate with the PostgreSQL community on this extension, we want to dive into some upcoming enhancements to pgvector. We will close by talking about how you can get started contributing and/or using this extension in your own workloads.
#AWS
#AWS
What's next in pgvector: Building AI-enabled apps with PostgreSQL - AWS Databases in 15
Vector databases are so hot right now. WTF are they?
Vector Databases simply explained! (Embeddings & Indexes)
PostgreSQL as VectorDB - Beginner Tutorial
OpenAI Embeddings and Vector Databases Crash Course
Build AI-powered apps on Google Cloud with pgvector, LangChain & LLMs
The missing pieces to your AI app (pgvector + RAG in prod)
Postgres pgvector Extension - Vector Database with PostgreSQL / Langchain Integration
Deep Dive: PostgreSQL(pgvector) vs MongoDB Vector Search for AI Applications
AWS re:Invent 2023 - Best practices for querying vector data for gen AI apps in PostgreSQL (DAT407)
How Postgres has adapted to AI and ML use cases using pgvector
A Beginner's Guide to Vector Embeddings
Jonathan S. Katz: Vectors are the new JSON (PGConf.EU 2023)
Spring AI With PostgreSQL pgvector: Building Generative AI Apps in Java
What is a Vector Database?
What is Retrieval-Augmented Generation (RAG)?
PostgreSQL as a Vector Database: Part 1, Getting Started With pgvector
Vector Database Explained | What is Vector Database?
Vector Search RAG Tutorial – Combine Your Data with LLMs with Advanced Search
pgvector for Python developers | POSETTE 2024
Supabase Vector: The Postgres Vector database: Paul Copplestone
Getting Started With pgvector and Amazon Aurora PostgreSQL
Why Build Enterprise RAG with Postgres?
How Does Rag Work? - Vector Database and LLMs #datascience #naturallanguageprocessing #llm #gpt
Комментарии