filmov
tv
Meta Llama 3 Fine tuning, RAG, and Prompt Engineering for Drug Discovery
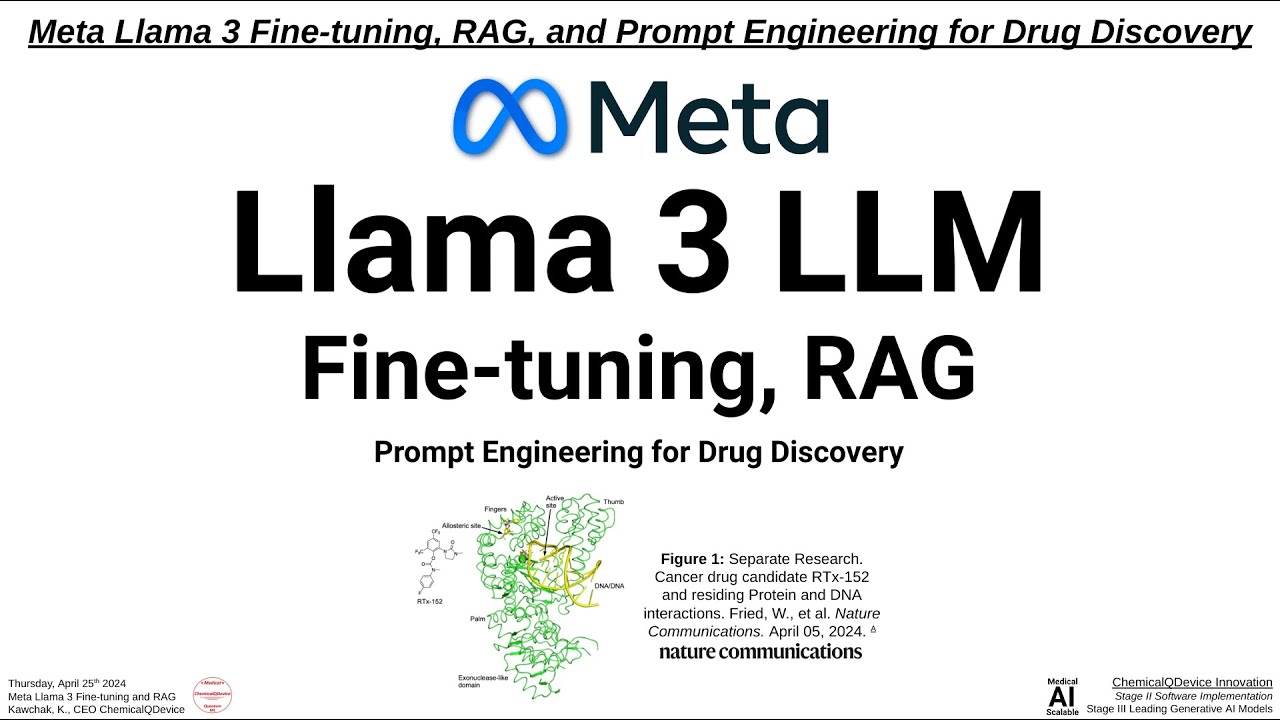
Показать описание
Large language models such as Meta's newly released Llama 3 have demonstrated state-of-the-art performance on standard benchmarks and real-world scenarios. (1) To further improve domain-specific generative AI answers, Fine-tuning on a different dataset, Prompt Engineering, and Retrieval Augmented Generation (RAG) are utilized to improve Llama 3 utility.
For enhanced usability, Llama 3 text-generations may need additional modifications, provide additional context, or use a specialized vocabulary. Fine-tuning is the process of further training the original pre-trained Llama 3 using domain-specific dataset(s). Prompt engineering doesn’t involve re-training Llama 3, but is the process of "designing and refining the input given to a model to guide and influence the kind of output you want." RAG "combines prompt engineering with context retrieval from external data sources to improve the performance and relevance of LLMs." (2)
The seminar will detail how to use Drug Discovery related datasets with the three LLM techniques mentioned above. The cover image depicts cancer drug candidate RTx-152 and residing Protein and DNA interactions, in Separate research. Fried, W., et al. Nature Communications. April 05, 2024. (A)
-CEO Kevin Kawchak
For enhanced usability, Llama 3 text-generations may need additional modifications, provide additional context, or use a specialized vocabulary. Fine-tuning is the process of further training the original pre-trained Llama 3 using domain-specific dataset(s). Prompt engineering doesn’t involve re-training Llama 3, but is the process of "designing and refining the input given to a model to guide and influence the kind of output you want." RAG "combines prompt engineering with context retrieval from external data sources to improve the performance and relevance of LLMs." (2)
The seminar will detail how to use Drug Discovery related datasets with the three LLM techniques mentioned above. The cover image depicts cancer drug candidate RTx-152 and residing Protein and DNA interactions, in Separate research. Fried, W., et al. Nature Communications. April 05, 2024. (A)
-CEO Kevin Kawchak
Комментарии