filmov
tv
Open the Black Box: an Introduction to Model Interpretability with LIME and SHAP - Kevin Lemagnen
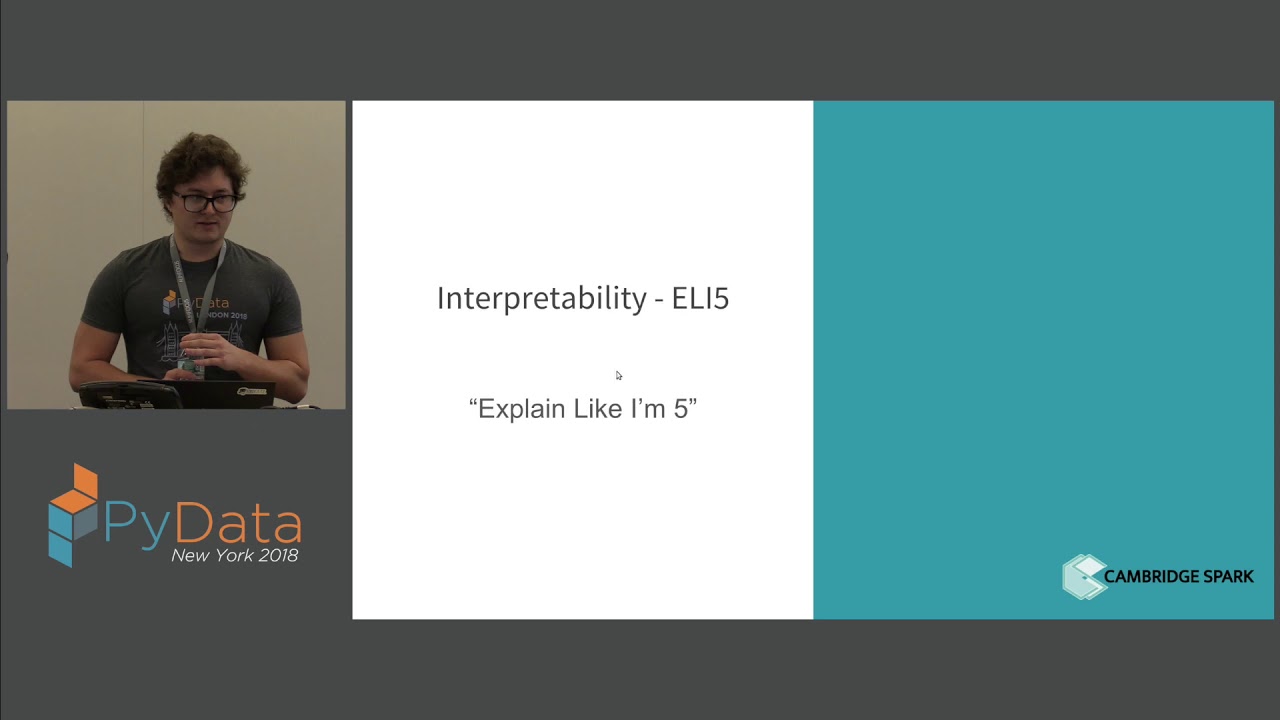
Показать описание
PyData NYC 2018
What's the use of sophisticated machine learning models if you can't interpret them? This workshop covers two recent model interpretability techniques that are essentials in your data scientist toolbox: LIME and SHAP. You will learn how to apply these techniques in Python on a real-world data science problem.
===
PyData is an educational program of NumFOCUS, a 501(c)3 non-profit organization in the United States. PyData provides a forum for the international community of users and developers of data analysis tools to share ideas and learn from each other. The global PyData network promotes discussion of best practices, new approaches, and emerging technologies for data management, processing, analytics, and visualization. PyData communities approach data science using many languages, including (but not limited to) Python, Julia, and R.
PyData conferences aim to be accessible and community-driven, with novice to advanced level presentations. PyData tutorials and talks bring attendees the latest project features along with cutting-edge use cases. 00:00 Welcome!
00:10 Help us add time stamps or captions to this video! See the description for details.
What's the use of sophisticated machine learning models if you can't interpret them? This workshop covers two recent model interpretability techniques that are essentials in your data scientist toolbox: LIME and SHAP. You will learn how to apply these techniques in Python on a real-world data science problem.
===
PyData is an educational program of NumFOCUS, a 501(c)3 non-profit organization in the United States. PyData provides a forum for the international community of users and developers of data analysis tools to share ideas and learn from each other. The global PyData network promotes discussion of best practices, new approaches, and emerging technologies for data management, processing, analytics, and visualization. PyData communities approach data science using many languages, including (but not limited to) Python, Julia, and R.
PyData conferences aim to be accessible and community-driven, with novice to advanced level presentations. PyData tutorials and talks bring attendees the latest project features along with cutting-edge use cases. 00:00 Welcome!
00:10 Help us add time stamps or captions to this video! See the description for details.
How to Open a Black Box (originally from MIT GAMBIT)
The Black Box Emergency | Javier Viaña | TEDxBoston
Russian experts open black box of downed jet
What's inside a Black Box?
What is Sukuna's Black Box? | Jujutsu Kaisen
Nier Automata Ver 1.1a Opening 2 Full - 'Black Box' by LiSA (Lyrics)
Black box on monitor fix
Jordan 12 Retro “Black Grey Wolf” Open Box 2024
Electronic Safe Opened in 10 seconds with Black Box | Mr. Locksmith Video
BetaFlight black box logging complete beginners setup guide. So easy a greasy FPV ninja can do it!
Open the Black Box: an Introduction to Model Interpretability with LIME and SHAP - Kevin Lemagnen
Opening up the black box | Nele Lea Uhlemann | TEDxHUBerlin
Open the Blackbox: Der Sound eines Elektroautos
SAS 4 | Opening a Black Box without using a key #3 (v 1.6.0)
WOT BLITZ the luckiest moment of my life ! ( BLACK BOX 10)
AI detectives are cracking open the black box of deep learning
Oren Matar: Opening the black box – an interpretable neural network architecture
EAGE Student E-Lecture: Cracking Open the Black Box by Jesper Sören Dramsch
Human Embryo Research: Opening the “Black Box”
Jessica Jean - Black Box (Official Music Video)
OpenAI o1 - the biggest black box of all. Let’s break it open.
BLACK BOX OPENING - WOT BLITZ #insane
Science and Technology Studies: Opening the Black Box
Guide to enable the Blackbox Code Autocomplete on VS Code
Комментарии