filmov
tv
121. Databricks | Pyspark| AutoLoader: Incremental Data Load
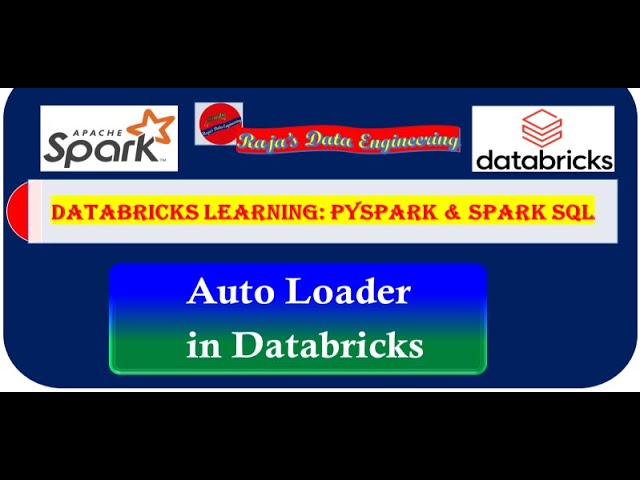
Показать описание
Azure Databricks Learning: Databricks and Pyspark: AutoLoader: Incremental Data Load
=====================================================================================
AutoLoader in Databricks is a crucial feature that streamlines the process of ingesting and processing large volumes of data efficiently. This automated data loading mechanism is instrumental for real-time or near-real-time data pipelines, allowing organizations to keep their data lakes up-to-date with minimal manual intervention. By automatically detecting and loading new or modified files from cloud storage, AutoLoader enhances data engineers' productivity, reduces latency in data availability, and ensures data accuracy. It plays a pivotal role in enabling timely insights and analytics, making it an indispensable component in modern data architectures.
To get more understanding, watch this video
#Databricks #AutoLoader #DataIngestion #DataEngineering #DataPipeline #BigData #DataIntegration #RealTimeData #DataAutomation #DataLake #Analytics #CloudComputing #DataProcessing #TechInnovation #DataEfficiency #DigitalTransformation #DataManagement #ETL #DataAccuracy #DataInsights #TechnologyTrends #DataAutomationBenefits #ApacheSpark #DataScience #ModernDataArchitecture #DataOps #InnovationInTech #PysparkforBeginners, #PysparkfromScratch, #SparkforBeginners, #SparkfromScratch,#DatabricksfromScratch, #DatabricksforBeginners, #AzureDatabricksTutorial,#DatabricksTutorialforBeginners,#DatabricksHandsonTutorial,#DataEngineeringProjectUsingPyspark, #PysparkAdvancedTutorial,#BestPysparkTutorial, #BestDatabricksTutorial, #BestSparkTutorial, #DatabricksETLPipeline, #AzureDatabricksPipeline, #AWSDatabricks, #GCPDatabricks
=====================================================================================
AutoLoader in Databricks is a crucial feature that streamlines the process of ingesting and processing large volumes of data efficiently. This automated data loading mechanism is instrumental for real-time or near-real-time data pipelines, allowing organizations to keep their data lakes up-to-date with minimal manual intervention. By automatically detecting and loading new or modified files from cloud storage, AutoLoader enhances data engineers' productivity, reduces latency in data availability, and ensures data accuracy. It plays a pivotal role in enabling timely insights and analytics, making it an indispensable component in modern data architectures.
To get more understanding, watch this video
#Databricks #AutoLoader #DataIngestion #DataEngineering #DataPipeline #BigData #DataIntegration #RealTimeData #DataAutomation #DataLake #Analytics #CloudComputing #DataProcessing #TechInnovation #DataEfficiency #DigitalTransformation #DataManagement #ETL #DataAccuracy #DataInsights #TechnologyTrends #DataAutomationBenefits #ApacheSpark #DataScience #ModernDataArchitecture #DataOps #InnovationInTech #PysparkforBeginners, #PysparkfromScratch, #SparkforBeginners, #SparkfromScratch,#DatabricksfromScratch, #DatabricksforBeginners, #AzureDatabricksTutorial,#DatabricksTutorialforBeginners,#DatabricksHandsonTutorial,#DataEngineeringProjectUsingPyspark, #PysparkAdvancedTutorial,#BestPysparkTutorial, #BestDatabricksTutorial, #BestSparkTutorial, #DatabricksETLPipeline, #AzureDatabricksPipeline, #AWSDatabricks, #GCPDatabricks
Комментарии