filmov
tv
Machine Learning Batch Size
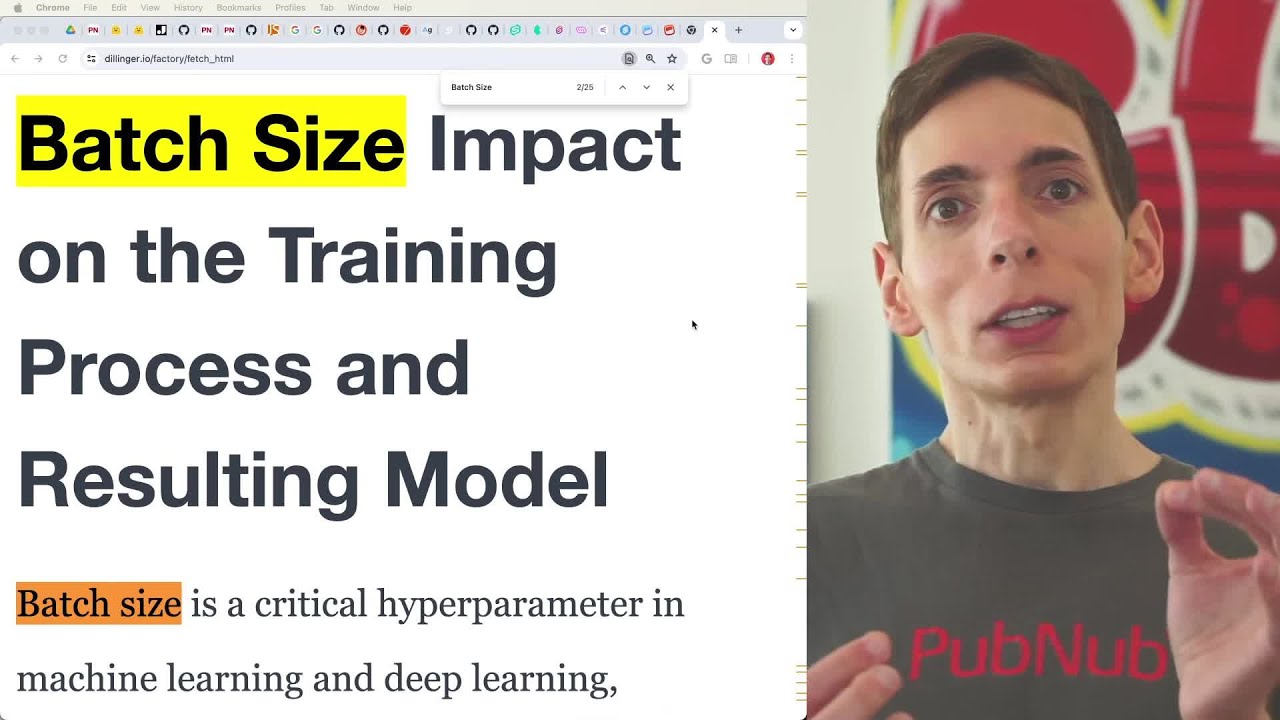
Показать описание
The batch size you use has a big impact on the machine learning model you're training and its final output. A small batch size means longer training times because it doesn't fully use your hardware. Large batch sizes, on the other hand, can quickly process data in parallel using the GPU, leading to faster training but updating the model less frequently.
This can sometimes cause over-generalization or underfitting. Smaller batches may provide noisier gradient estimates which help avoid local minima, potentially leading to better model performance but can also cause overfitting. Using smaller GPUs can work well with smaller batches, but the training will take longer.
Large batches tend to give smoother, more stable gradients and faster convergence, as they process and update with larger data chunks. this can lead to missing the benefits of the noisier gradients that smaller batches offer. Small batches can act as a form of regularization, preventing overfitting, while large batches can overfit or underfit more easily. Adjusting the batch size can help with overfitting or underfitting issues. It mostly depends on your data.
To balance training efficiency and model accuracy, you may want to slightly lean towards smaller batch sizes, even though it means longer training times.
This can sometimes cause over-generalization or underfitting. Smaller batches may provide noisier gradient estimates which help avoid local minima, potentially leading to better model performance but can also cause overfitting. Using smaller GPUs can work well with smaller batches, but the training will take longer.
Large batches tend to give smoother, more stable gradients and faster convergence, as they process and update with larger data chunks. this can lead to missing the benefits of the noisier gradients that smaller batches offer. Small batches can act as a form of regularization, preventing overfitting, while large batches can overfit or underfit more easily. Adjusting the batch size can help with overfitting or underfitting issues. It mostly depends on your data.
To balance training efficiency and model accuracy, you may want to slightly lean towards smaller batch sizes, even though it means longer training times.
Комментарии