filmov
tv
Paper Review Call 019 - UMAP
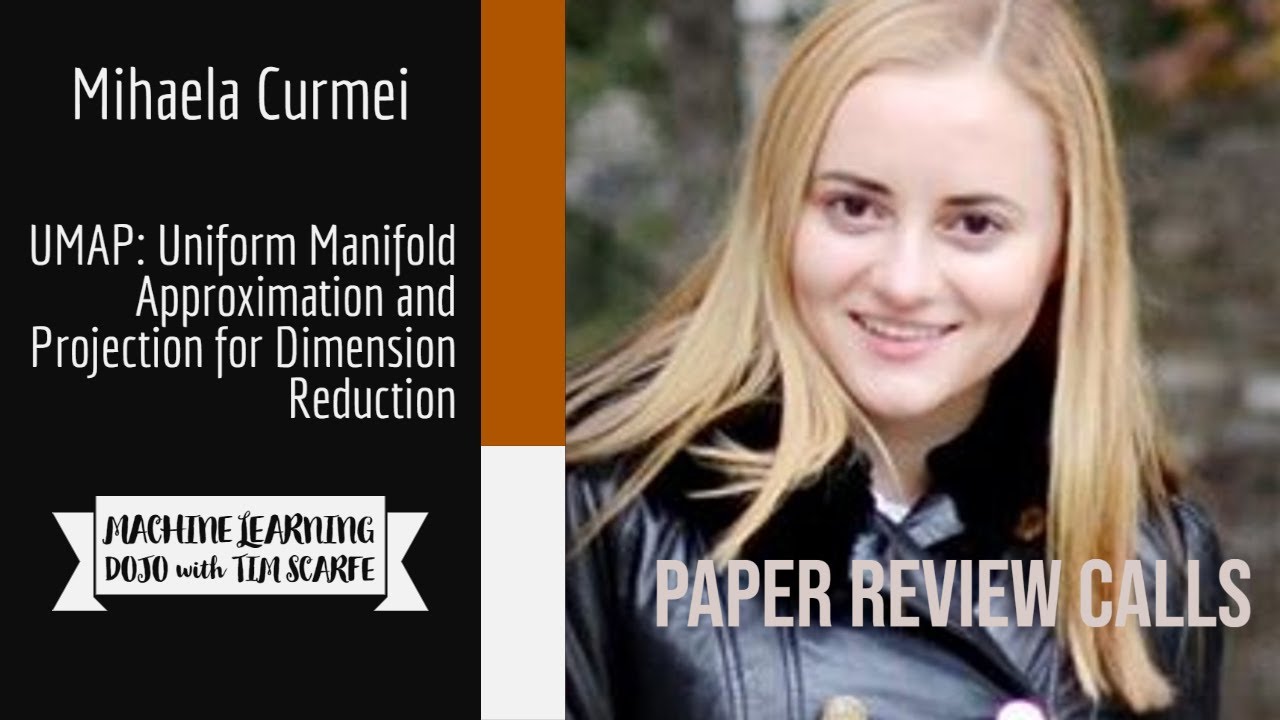
Показать описание
Paper Review Call 21: UMAP: Uniform Manifold Approximation and Projection for Dimension Reduction
COMPLIANCE STATEMENT:
* THIS IS NOT A MICROSOFT YOUTUBE CHANNEL
* WE ARE NOT A SPOKESPEOPLE FOR MICROSOFT I.E. OUR OPINIONS
* THERE IS NO MICROSOFT-CONFIDENTIAL INFO IN THIS VIDEO, IT'S AN EDUCATIONAL VIDEO ON MACHINE LEARNING, ALL INFO IS IN PUBLIC DOMAIN!
* EVERYONE IN THE VIDEO HAS GIVEN THEIR EXPLICIT PERMISSION FOR PUBLICATION ON YOUTUBE AND WAS AWARE IT WAS CREATED FOR THIS PURPOSE
* I WORKED FOR MICROSOFT AT THE TIME THIS CONTENT WAS CREATED
Interested in dimensionality reduction? TSNE is so last century, these days it’s all about UMAP!
UMAP (Uniform Manifold Approximation and Projection) is a novel manifold learning technique for dimension reduction. UMAP is constructed from a theoretical framework based in Riemannian geometry and algebraic topology. The result is a practical scalable algorithm that applies to real world data. The UMAP algorithm is competitive with t-SNE for visualization quality, and arguably preserves more of the global structure with superior run time performance. Furthermore, UMAP has no computational restrictions on embedding dimension, making it viable as a general purpose dimension reduction technique for machine learning.
Mihaela Curmei is going to expertly dissect this paper in detail as well as offer some practical engineering advice around dimensionality reduction in general and explain the pros and cons of TSNE vs UMAP. Having just done a dry run with Mihaela, I can promise you that this is NOT ONE TO MISS!
@article{mcinnes2018umap,
title={Umap: Uniform manifold approximation and projection for dimension reduction},
author={McInnes, Leland and Healy, John and Melville, James},
journal={arXiv preprint arXiv:1802.03426},
year={2018}
}
COMPLIANCE STATEMENT:
* THIS IS NOT A MICROSOFT YOUTUBE CHANNEL
* WE ARE NOT A SPOKESPEOPLE FOR MICROSOFT I.E. OUR OPINIONS
* THERE IS NO MICROSOFT-CONFIDENTIAL INFO IN THIS VIDEO, IT'S AN EDUCATIONAL VIDEO ON MACHINE LEARNING, ALL INFO IS IN PUBLIC DOMAIN!
* EVERYONE IN THE VIDEO HAS GIVEN THEIR EXPLICIT PERMISSION FOR PUBLICATION ON YOUTUBE AND WAS AWARE IT WAS CREATED FOR THIS PURPOSE
* I WORKED FOR MICROSOFT AT THE TIME THIS CONTENT WAS CREATED
Interested in dimensionality reduction? TSNE is so last century, these days it’s all about UMAP!
UMAP (Uniform Manifold Approximation and Projection) is a novel manifold learning technique for dimension reduction. UMAP is constructed from a theoretical framework based in Riemannian geometry and algebraic topology. The result is a practical scalable algorithm that applies to real world data. The UMAP algorithm is competitive with t-SNE for visualization quality, and arguably preserves more of the global structure with superior run time performance. Furthermore, UMAP has no computational restrictions on embedding dimension, making it viable as a general purpose dimension reduction technique for machine learning.
Mihaela Curmei is going to expertly dissect this paper in detail as well as offer some practical engineering advice around dimensionality reduction in general and explain the pros and cons of TSNE vs UMAP. Having just done a dry run with Mihaela, I can promise you that this is NOT ONE TO MISS!
@article{mcinnes2018umap,
title={Umap: Uniform manifold approximation and projection for dimension reduction},
author={McInnes, Leland and Healy, John and Melville, James},
journal={arXiv preprint arXiv:1802.03426},
year={2018}
}
Комментарии