filmov
tv
Do Statistical Models Understand the World? - Ian Goodfellow, Research Scientist, Google
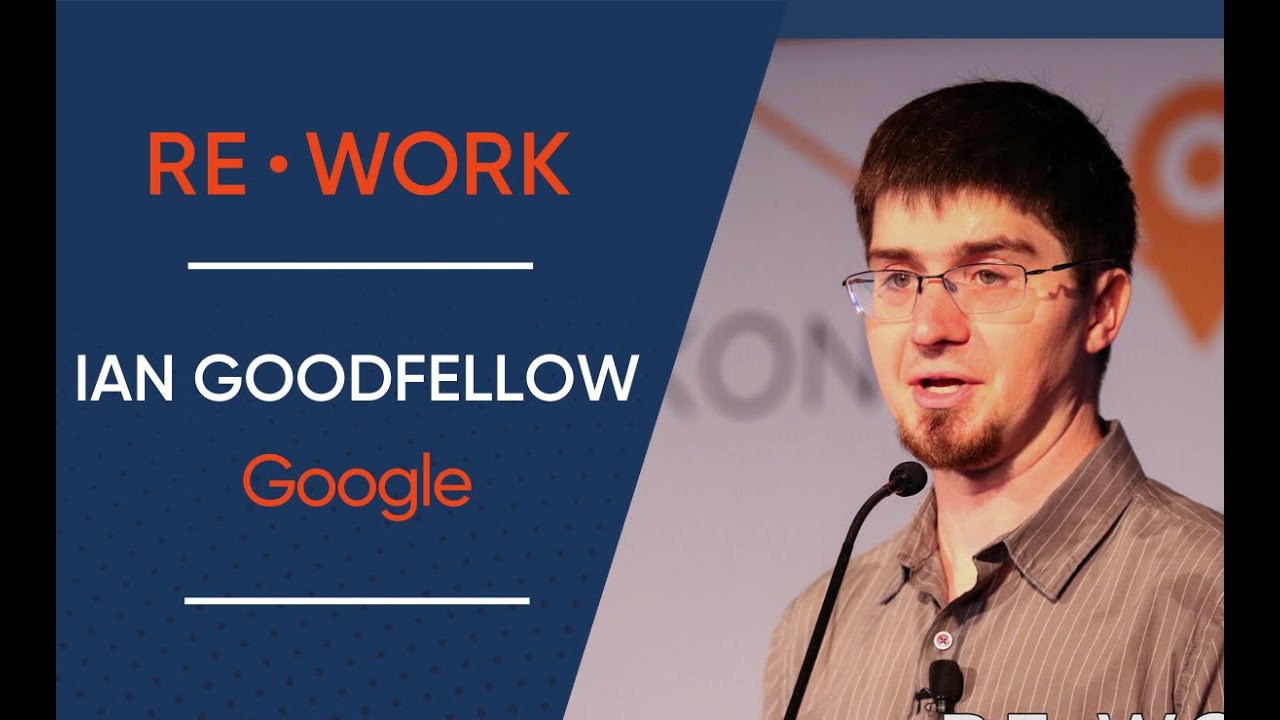
Показать описание
'Do Statistical Models Understand the World?' - Ian Goodfellow, Research Scientist, Google
Machine learning algorithms have reached human-level performance on a variety of benchmark tasks. This raises the question of whether these algorithms have also reached human-level 'understanding' of these tasks. By designing inputs specifically to confuse machine learning algorithms, we show that statistical models ranging from logistic regression to deep convolutional networks fail in predictable ways when presented with statistically unusual inputs. Our results suggest that deep networks have the potential to overcome this problem, but modern deep networks behave too much like shallow, linear models.
At the time of this presentation, Ian Goodfellow was a research scientist at Google. He earned a PhD in machine learning from Université de Montréal in 2014. His PhD advisors were Yoshua Bengio and Aaron Courville. His studies were funded by the Google PhD Fellowship in Deep Learning. During his PhD studies, he wrote Pylearn2, the open-source deep learning research library, and introduced a variety of new deep learning algorithms. Previously, he obtained a BSc and MSc in computer science from Stanford University, where he was one of the earliest members of Andrew Ng's deep learning research group.
Machine learning algorithms have reached human-level performance on a variety of benchmark tasks. This raises the question of whether these algorithms have also reached human-level 'understanding' of these tasks. By designing inputs specifically to confuse machine learning algorithms, we show that statistical models ranging from logistic regression to deep convolutional networks fail in predictable ways when presented with statistically unusual inputs. Our results suggest that deep networks have the potential to overcome this problem, but modern deep networks behave too much like shallow, linear models.
At the time of this presentation, Ian Goodfellow was a research scientist at Google. He earned a PhD in machine learning from Université de Montréal in 2014. His PhD advisors were Yoshua Bengio and Aaron Courville. His studies were funded by the Google PhD Fellowship in Deep Learning. During his PhD studies, he wrote Pylearn2, the open-source deep learning research library, and introduced a variety of new deep learning algorithms. Previously, he obtained a BSc and MSc in computer science from Stanford University, where he was one of the earliest members of Andrew Ng's deep learning research group.
Комментарии