filmov
tv
Compressing Variational Bayes by Dr. Stephan Mandt
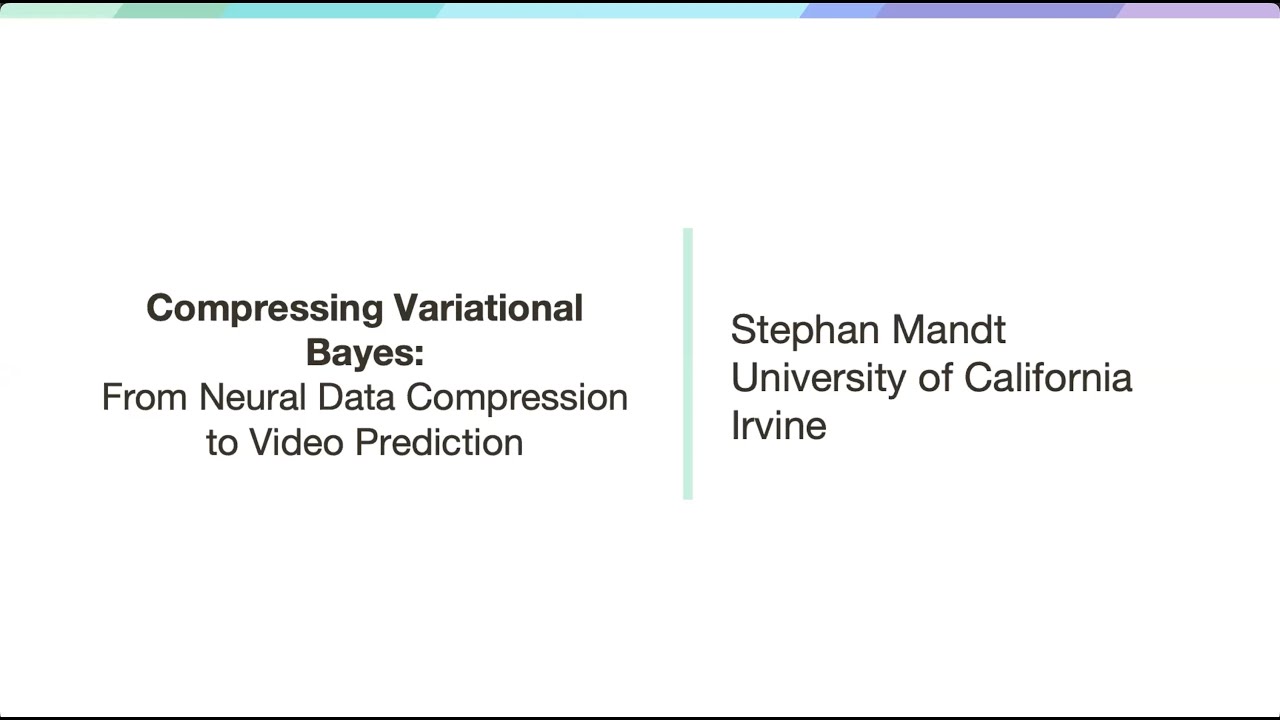
Показать описание
Content:
00:00 - Introduction
01:12 - Deep Latent Sequence Models
03:12 - Approximate Bayesian Inference
08:20 - Neural Data Compression
40:30 - High-dimensional Sequence Forecasting and Compression
48:05 - Summary
48:48 - Q&A
Abstract:
Recent research has shown surprising connections between variational Bayesian inference (VI) and neural data compression. This talk will review several theoretical and applied contributions in this domain. I will first show how VI enables computing upper and lower bounds on the information rate-distortion function of an empirical data source, a quantity that could previously not be estimated beyond a few dimensions. I will then show how semi-amortized variational inference can improve neural image compression, how posterior uncertainties give rise to a new type of adaptive entropy coding, and how sequential variational autoencoders can be converted into practical video codecs. Finally, I will show how neural video codecs can inspire design choices for extending diffusion models to video data.
Bio:
Stephan Mandt is an Assistant Professor of Computer Science and Statistics at the University of California, Irvine. From 2016 until 2018, he was a Senior Researcher and Head of the statistical machine learning group at Disney Research in Pittsburgh and Los Angeles. He held previous postdoctoral positions at Columbia University and Princeton University. Stephan holds a Ph.D. in Theoretical Physics from the University of Cologne, where he received the German National Merit Scholarship. He is furthermore a receipient of the NSF CAREER Award, the UCI ICS Mid-Career Excellence in Research Award, a Kavli Fellow of the U.S. National Academy of Sciences, a member of the ELLIS Society, and a former visiting researcher at Google Brain. Stephan regularly serves as an Area Chair, Action Editor, or Editorial Board member for NeurIPS, ICML, AAAI, ICLR, TMLR, and JMLR. His research is currently supported by NSF, DARPA, DOE, Disney, Intel, and Qualcomm.
00:00 - Introduction
01:12 - Deep Latent Sequence Models
03:12 - Approximate Bayesian Inference
08:20 - Neural Data Compression
40:30 - High-dimensional Sequence Forecasting and Compression
48:05 - Summary
48:48 - Q&A
Abstract:
Recent research has shown surprising connections between variational Bayesian inference (VI) and neural data compression. This talk will review several theoretical and applied contributions in this domain. I will first show how VI enables computing upper and lower bounds on the information rate-distortion function of an empirical data source, a quantity that could previously not be estimated beyond a few dimensions. I will then show how semi-amortized variational inference can improve neural image compression, how posterior uncertainties give rise to a new type of adaptive entropy coding, and how sequential variational autoencoders can be converted into practical video codecs. Finally, I will show how neural video codecs can inspire design choices for extending diffusion models to video data.
Bio:
Stephan Mandt is an Assistant Professor of Computer Science and Statistics at the University of California, Irvine. From 2016 until 2018, he was a Senior Researcher and Head of the statistical machine learning group at Disney Research in Pittsburgh and Los Angeles. He held previous postdoctoral positions at Columbia University and Princeton University. Stephan holds a Ph.D. in Theoretical Physics from the University of Cologne, where he received the German National Merit Scholarship. He is furthermore a receipient of the NSF CAREER Award, the UCI ICS Mid-Career Excellence in Research Award, a Kavli Fellow of the U.S. National Academy of Sciences, a member of the ELLIS Society, and a former visiting researcher at Google Brain. Stephan regularly serves as an Area Chair, Action Editor, or Editorial Board member for NeurIPS, ICML, AAAI, ICLR, TMLR, and JMLR. His research is currently supported by NSF, DARPA, DOE, Disney, Intel, and Qualcomm.