filmov
tv
ABCs of Conditional Independence (Detailed Edition: Hands-on Example and Beyond!)
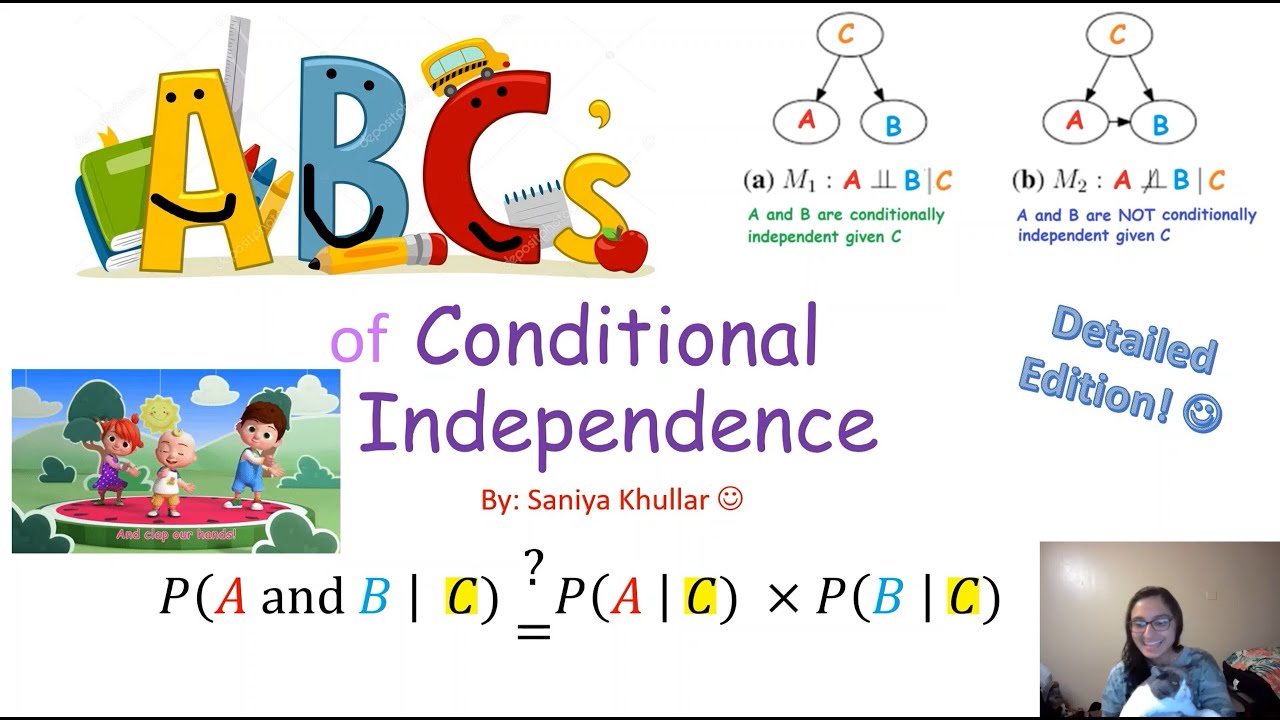
Показать описание
Do you want to be a superstar in conditional independence? Then, please fear no more!
Plus, Saniya hopes you appreciate the title pun since Saniya's example uses A, B, and C random variables to help teach ye about Conditional Independence :)
TIME STAMPS:
00:00 ABCs of Conditional Independence (Detailed Edition: Hands-on Example and Beyond!)
02:05 The Big Question (Hands-on Example we will work on here)
04:11 Conditional Independence: Common Cause versus Common Effect
06:27 What does Conditional Independence really mean?
13:26 Another example of Conditional Independence (Using Bayesian Networks)
14:58 Back to the Hands-On Example in this video
19:08 Marginal Independence for 2 random variables (intuition for conditional independence)
20:22 Interpreting and comparing what conditional independence looks like versus no conditional independence
22:40 Quick Review on Bayes Rule for Probability
25:48 Quick Review on Testing Independence for 2 Random Variables (e.g. Are A and B independent random variables)?
29:49 Extending concepts of independence to 3 random variables and conditional independence
40:01 8 tests to determine if A and B are conditionally independent given C
46:01 Obtaining Initial Probabilities from Joint Probability Table
01:05:36 Reminder about the 8 tests we need to do
01:06:26 Reference sheet of probabilities we have found
01:07:15 Test 1 (Fails): P(A = True | C = True) x P(B = True | C = True) = P(A = True and B = True | C = True)?
01:14:13 Since At least 1 Test Fails, A and B are NOT conditionally independent given C
==== Please note below Saniya shows the other tests for your practice and reinforcement (but in reality we would have been done now) ===================================
01:14:13 Test 2 (Fails): P(A = True | C = True) x P(B = False | C = True) = P(A = True and B = False | C = True)?
01:18:25 Test 3 (Fails): P(A = False | C = True) x P(B = True | C = True) = P(A = False and B = True | C = True)?
01:20:31 Test 4 (Fails): P(A = False | C = True) x P(B = False | C = True) = P(A = False and B = False | C = True)?
01:22:57 Test 5 (Passes): P(A = True | C = False) x P(B = True | C = False) = P(A = True and B = True | C = False)?
01:26:47 Test 6 (Passes): P(A = True | C = False) x P(B = False | C = False) = P(A = True and B = False | C = False)?
01:28:28 Test 7 (Passes): P(A = False | C = False) x P(B = True | C = False) = P(A = False and B = True | C = False)?
01:31:17 Test 8 (Passes): P(A = False | C = False) x P(B = False | C = False) = P(A = False and B = False | C = False)?
01:33:104 Summarizing the Answer: A and B are NOT independent given C in this example
01:36:01 Going Beyond: What if instead A and B ARE independent given C? What can we learn?
01:38:35 Derivation: P(B | A and C) = P(B | C) if A and B are independent given C
01:42:27 Derivation: P(A | B and C) = P(A | C) if A and B are independent given C
01:43:58 Conditional Independence is important in Bayesian Networks
Plus, Saniya hopes you appreciate the title pun since Saniya's example uses A, B, and C random variables to help teach ye about Conditional Independence :)
TIME STAMPS:
00:00 ABCs of Conditional Independence (Detailed Edition: Hands-on Example and Beyond!)
02:05 The Big Question (Hands-on Example we will work on here)
04:11 Conditional Independence: Common Cause versus Common Effect
06:27 What does Conditional Independence really mean?
13:26 Another example of Conditional Independence (Using Bayesian Networks)
14:58 Back to the Hands-On Example in this video
19:08 Marginal Independence for 2 random variables (intuition for conditional independence)
20:22 Interpreting and comparing what conditional independence looks like versus no conditional independence
22:40 Quick Review on Bayes Rule for Probability
25:48 Quick Review on Testing Independence for 2 Random Variables (e.g. Are A and B independent random variables)?
29:49 Extending concepts of independence to 3 random variables and conditional independence
40:01 8 tests to determine if A and B are conditionally independent given C
46:01 Obtaining Initial Probabilities from Joint Probability Table
01:05:36 Reminder about the 8 tests we need to do
01:06:26 Reference sheet of probabilities we have found
01:07:15 Test 1 (Fails): P(A = True | C = True) x P(B = True | C = True) = P(A = True and B = True | C = True)?
01:14:13 Since At least 1 Test Fails, A and B are NOT conditionally independent given C
==== Please note below Saniya shows the other tests for your practice and reinforcement (but in reality we would have been done now) ===================================
01:14:13 Test 2 (Fails): P(A = True | C = True) x P(B = False | C = True) = P(A = True and B = False | C = True)?
01:18:25 Test 3 (Fails): P(A = False | C = True) x P(B = True | C = True) = P(A = False and B = True | C = True)?
01:20:31 Test 4 (Fails): P(A = False | C = True) x P(B = False | C = True) = P(A = False and B = False | C = True)?
01:22:57 Test 5 (Passes): P(A = True | C = False) x P(B = True | C = False) = P(A = True and B = True | C = False)?
01:26:47 Test 6 (Passes): P(A = True | C = False) x P(B = False | C = False) = P(A = True and B = False | C = False)?
01:28:28 Test 7 (Passes): P(A = False | C = False) x P(B = True | C = False) = P(A = False and B = True | C = False)?
01:31:17 Test 8 (Passes): P(A = False | C = False) x P(B = False | C = False) = P(A = False and B = False | C = False)?
01:33:104 Summarizing the Answer: A and B are NOT independent given C in this example
01:36:01 Going Beyond: What if instead A and B ARE independent given C? What can we learn?
01:38:35 Derivation: P(B | A and C) = P(B | C) if A and B are independent given C
01:42:27 Derivation: P(A | B and C) = P(A | C) if A and B are independent given C
01:43:58 Conditional Independence is important in Bayesian Networks